Fred Nwanganga - Practical Machine Learning in R
Here you can read online Fred Nwanganga - Practical Machine Learning in R full text of the book (entire story) in english for free. Download pdf and epub, get meaning, cover and reviews about this ebook. year: 2020, publisher: Wiley, genre: Computer. Description of the work, (preface) as well as reviews are available. Best literature library LitArk.com created for fans of good reading and offers a wide selection of genres:
Romance novel
Science fiction
Adventure
Detective
Science
History
Home and family
Prose
Art
Politics
Computer
Non-fiction
Religion
Business
Children
Humor
Choose a favorite category and find really read worthwhile books. Enjoy immersion in the world of imagination, feel the emotions of the characters or learn something new for yourself, make an fascinating discovery.

- Book:Practical Machine Learning in R
- Author:
- Publisher:Wiley
- Genre:
- Year:2020
- Rating:3 / 5
- Favourites:Add to favourites
- Your mark:
- 60
- 1
- 2
- 3
- 4
- 5
Practical Machine Learning in R: summary, description and annotation
We offer to read an annotation, description, summary or preface (depends on what the author of the book "Practical Machine Learning in R" wrote himself). If you haven't found the necessary information about the book — write in the comments, we will try to find it.
Practical Machine Learning in R — read online for free the complete book (whole text) full work
Below is the text of the book, divided by pages. System saving the place of the last page read, allows you to conveniently read the book "Practical Machine Learning in R" online for free, without having to search again every time where you left off. Put a bookmark, and you can go to the page where you finished reading at any time.
Font size:
Interval:
Bookmark:
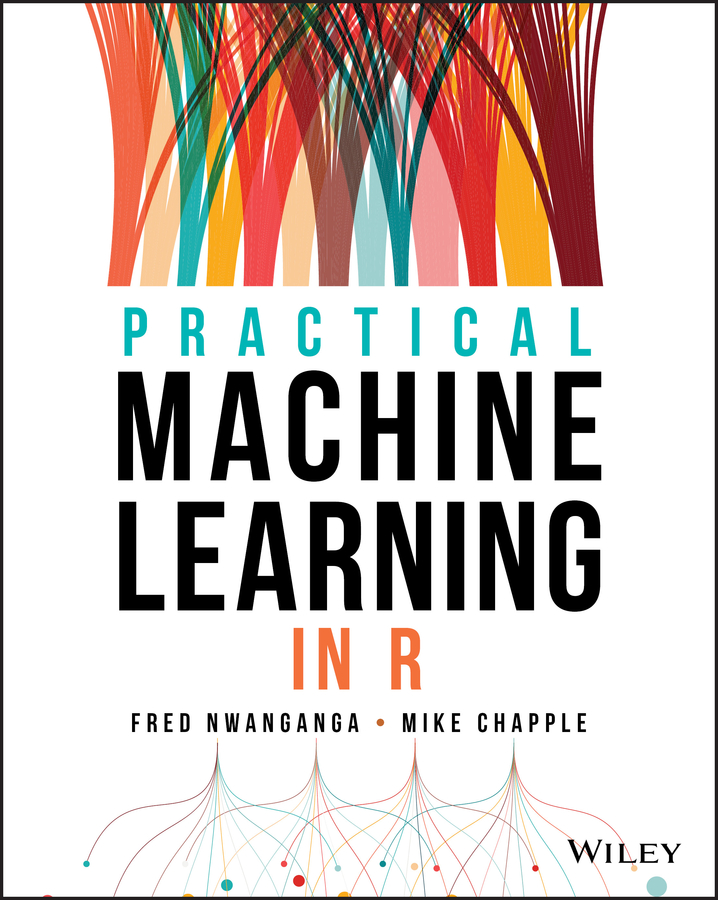
- Chapter 4
- Chapter 7
- Chapter 1
- Chapter 2
- Chapter 3
- Chapter 4
- Chapter 5
- Chapter 6
- Chapter 8
- Chapter 9
- Chapter 10
- Chapter 11
- Chapter 12
FRED NWANGANGA
MIKE CHAPPLE
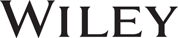
Machine learning is changing the world. Every organization, large and small, seeks to extract knowledge from the massive amounts of information that they store and process on a daily basis. The tantalizing desire to predict the future drives the work of business analysts and data scientists in fields ranging from marketing to healthcare. Our goal with this book is to make the tools of analytics approachable for a broad audience.
The R programming language is a purpose-specific language designed to facilitate statistical analysis and machine learning. We choose it for this book not only due to its strong popularity in the field but also because of its intuitive nature, particularly for individuals approaching it as their first programming language.
There are many books on the market that cover practical applications of machine learning, designed for businesspeople and onlookers. Likewise, there are many deeply technical resources that dive into the mathematics and computer science of machine learning. In this book, we strive to bridge these two worlds. We attempt to bring the reader an intuitive introduction to machine learning with an eye on the practical applications of machine learning in today's world. At the same time, we don't shy away from code. As we do in our undergraduate and graduate courses, we seek to make the R programming language accessible to everyone. Our hope is that you will read this book with your laptop open next to you, following along with our examples and trying your hand at the exercises.
Best of luck as you begin your machine learning adventure!
This book provides an introduction to machine learning using the R programming language.
- : What Is Machine Learning?This chapter introduces the world of machine learning and describes how machine learning allows the discovery of knowledge in data. In this chapter, we explain the differences between unsupervised learning, supervised learning, and reinforcement learning. We describe the differences between classification and regression problems and explain how to measure the effectiveness of machine learning algorithms.
- : Introduction to R and RStudioIn this chapter, we introduce the R programming language and the toolset that we will be using throughout the rest of the book. We approach R from the beginner's mind-set, explain the use of the RStudio integrated development environment, and walk readers through the creation and execution of their first R scripts. We also explain the use of packages to redistribute R code and the use of different data types in R.
- : Managing DataThis chapter introduces readers to the concepts of data management and the use of R to collect and manage data. We introduce the tidyverse, a collection of R packages designed to facilitate the analytics process, and we describe different approaches to describing and visualizing data in R. We also cover how to clean, transform, and reduce data to prepare it for machine learning.
- : Linear RegressionIn this chapter, we dive into the world of supervised machine learning as we explore linear regression. We explain the underlying statistical principles behind regression and demonstrate how to fit simple and complex regression models in R. We also explain how to evaluate, interpret, and apply the results of regression models.
- : Logistic RegressionWhile linear regression is suitable for problems that require the prediction of numeric values, it is not well-suited to categorical predictions. In this chapter, we describe logistic regression, a categorical prediction technique. We discuss the use of generalized linear models and describe how to build logistic regression models in R. We also explain how to evaluate, interpret, and improve upon the results of a logistic regression model.
- : k-Nearest NeighborsThe k-nearest neighbors technique allows us to predict the classification of a data point based on the classifications of other, similar data points. In this chapter, we describe how the k-NN process works and demonstrate how to build a k-NN model in R. We also show how to apply that model, making predictions about the classifications of new data points.
- : Nave BayesThe nave Bayes approach to classification uses a table of probabilities to predict the likelihood that an instance belongs to a particular class. In this chapter, we discuss the concepts of joint and conditional probability and describe how the Bayes classification approach functions. We demonstrate building a nave Bayes classifier in R and use it to make predictions about previously unseen data.
- : Decision TreesDecision trees are a popular modeling technique because they produce intuitive results. In this chapter, we describe the creation and interpretation of decision tree models. We also explain the process of growing a tree in R and using pruning to increase the generalizability of that model.
- : Evaluating PerformanceNo modeling technique is perfect. Each has its own strengths and weaknesses and brings different predictive power to different types of problems. In this chapter, we discuss the process of evaluating model performance. We introduce resampling techniques and explain how they can be used to estimate the future performance of a model. We also demonstrate how to visualize and evaluate model performance in R.
- : Improving PerformanceOnce we have tools to evaluate the performance of a model, we can then apply them to help improve model performance. In this chapter, we look at techniques for tuning machine learning models. We also demonstrate how we can enhance our predictive power by simultaneously harnessing the predictive capability of multiple models.
- : Discovering Patterns with Association RulesAssociation rules help us discover patterns that exist within a dataset. In this chapter, we introduce the association rules approach and demonstrate how to generate association rules from a dataset in R. We also explain ways to evaluate and quantify the strength of association rules.
- : Grouping Data with ClusteringClustering is an unsupervised learning technique that groups items based on their similarity to each other. In this chapter, we explain the way that the k-means clustering algorithm segments data and demonstrate the use of k-means clustering in R.
In order to make the most of this book, we encourage you to make use of the student and instructor materials made available on the companion site. We also encourage you to provide us with meaningful feedback on ways in which we could improve the book.
As you work through the examples in this book, you may choose either to type in all the code manually or to use the source code files that accompany the book. If you choose to follow along with the examples, you will also want to use the same datasets we use throughout the book. All the source code and datasets used in this book are available for download from
Font size:
Interval:
Bookmark:
Similar books «Practical Machine Learning in R»
Look at similar books to Practical Machine Learning in R. We have selected literature similar in name and meaning in the hope of providing readers with more options to find new, interesting, not yet read works.
Discussion, reviews of the book Practical Machine Learning in R and just readers' own opinions. Leave your comments, write what you think about the work, its meaning or the main characters. Specify what exactly you liked and what you didn't like, and why you think so.