Setareh Rafatirad , Houman Homayoun , Zhiqian Chen and Sai Manoj Pudukotai Dinakarrao
Machine Learning for Computer Scientists and Data Analysts
From an Applied Perspective
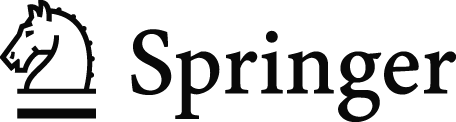
Logo of the publisher
Setareh Rafatirad
George Mason University, Fairfax, VA, USA
Houman Homayoun
University of California, Davis, Davis, CA, USA
Zhiqian Chen
Mississippi State University, Mississippi State, MS, USA
Sai Manoj Pudukotai Dinakarrao
George Mason University, Fairfax, VA, USA
ISBN 978-3-030-96755-0 e-ISBN 978-3-030-96756-7
https://doi.org/10.1007/978-3-030-96756-7
The Editor(s) (if applicable) and The Author(s), under exclusive license to Springer Nature Switzerland AG 2022
This work is subject to copyright. All rights are solely and exclusively licensed by the Publisher, whether the whole or part of the material is concerned, specifically the rights of translation, reprinting, reuse of illustrations, recitation, broadcasting, reproduction on microfilms or in any other physical way, and transmission or information storage and retrieval, electronic adaptation, computer software, or by similar or dissimilar methodology now known or hereafter developed.
The use of general descriptive names, registered names, trademarks, service marks, etc. in this publication does not imply, even in the absence of a specific statement, that such names are exempt from the relevant protective laws and regulations and therefore free for general use.
The publisher, the authors, and the editors are safe to assume that the advice and information in this book are believed to be true and accurate at the date of publication. Neither the publisher nor the authors or the editors give a warranty, expressed or implied, with respect to the material contained herein or for any errors or omissions that may have been made. The publisher remains neutral with regard to jurisdictional claims in published maps and institutional affiliations.
This Springer imprint is published by the registered company Springer Nature Switzerland AG
The registered company address is: Gewerbestrasse 11, 6330 Cham, Switzerland
Preface
The recent popularity gained by the field of machine learning (ML) has led to its adaptation into almost all the known applications. The applications range from smart homes, smart grids, and forex markets to military applications and autonomous drones. There exists a plethora of machine learning techniques that were introduced in the past few years, and each of these techniques fits greatly for a specific set of applications rather than a one-size-fits-all approach.
In order to better determine the application of ML for a given problem, it is non-trivial to understand the current state of the art of the existing ML techniques, pros and cons, their behavior, and existing applications that have already adopted them. This book thus aims at researchers and practitioners who are familiar with their application requirements, and are interested in the application of ML techniques in their applications not only for better performance but also for ensuring that the adopted ML technique is not an overkill to the considered application. We hope that this book will provide a structured introduction and relevant background to aspiring engineers who are new to the field, while also helping to revise the background for the researchers familiar with this field. This introduction will be further used to build and introduce current and emerging ML paradigms and their applications in multiple case studies.
Organization
This book is organized into three parts that consist of multiple chapters. The first part introduces the relevant background information pertaining to ML, traditional learning approaches that are widely used.
Chapter introduces the concept of applied machine learning. The metrics used for evaluating the machine learning performance, data pre-processing, and techniques to visualize and analyze the outputs (classification or regression or other applications) are discussed.
Chapter presents a brief review of the probability theory and linear algebra that are essential for a better understanding of the ML techniques discussed in the later parts of the book.
Chapter introduces the machine learning techniques. Supervised learning is primarily discussed in this chapter. Multiple supervised learning techniques, learning techniques, and applications along with pros and cons for each of the techniques are discussed. A qualitative comparison of different supervised learning techniques is presented along with their suitability to different kinds of applications.
Unsupervised learning is introduced in Chap.. The differences compared to the supervised learning and application scenarios are discussed first. Different supervised learning for different applications including classification and feature selection is discussed along with examples in this chapter.
Reinforcement learning is a human learning-inspired technique, which can be laid between supervised and unsupervised learning techniques in the spectrum. Chapter discusses the basics of reinforcement learning along with its variants together with a comparison among different techniques.
Building on top of the basic concepts of machine learning, advanced machine learning techniques used in real-world applications are discussed in the second part of this book.
The majority of the supervised learning techniques and their learning mechanisms discussed in the first part of this book focus on offline or batch learning. However, the learning in real-world applications needs to happen in an online manner. As such, Chap. introduces the online learning technique and different variants of online learning techniques.
With a diverse spectrum of Web applications demonstrating the importance of learning from user behavior, recommender systems are widely used by the bulk of social media companies. Chapter of this book discusses approaches for recommender learning.
Chapter offers approaches for graph learning. Graphs are used to depict things and their connections in a variety of real-world applications, including social networking, transportation, and disease spreading. Methods for learning graphs and the relationships between nodes are discussed.
In addition to advancements in machine learning algorithms, researchers have also focused on exploiting the vulnerabilities in machine learning techniques. Chapter introduces adversarial machine learning techniques that discuss techniques to inject the adversarial perturbations into the input samples to mislead the machine learning algorithms. In addition, the techniques to harden the machine learning techniques against these adversarial perturbations are discussed.
In addition to the advanced learning techniques, the application of machine learning algorithms with entire discussions dedicated to real-world applications is presented in the third part of this book.
The application of machine learning techniques for health monitoring is one of the critical real-world applications, especially with the introduction of wearable devices including fitness trackers. Chapters focus on the application of machine learning techniques for health applications, particularly in the context of wearable devices.