Getting Started with Streamlit for Data Science
Create and deploy Streamlit web applications from scratch in Python
Tyler Richards
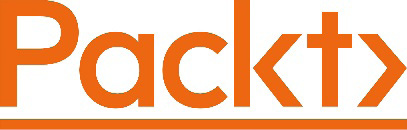
BIRMINGHAMMUMBAI
Getting Started with Streamlit for Data Science
Copyright 2021 Packt Publishing
All rights reserved. No part of this book may be reproduced, stored in a retrieval system, or transmitted in any form or by any means, without the prior written permission of the publisher, except in the case of brief quotations embedded in critical articles or reviews.
Every effort has been made in the preparation of this book to ensure the accuracy of the information presented. However, the information contained in this book is sold without warranty, either express or implied. Neither the author, nor Packt Publishing or its dealers and distributors, will be held liable for any damages caused or alleged to have been caused directly or indirectly by this book.
Packt Publishing has endeavored to provide trademark information about all of the companies and products mentioned in this book by the appropriate use of capitals. However, Packt Publishing cannot guarantee the accuracy of this information.
Group Product Manager: Kunal Parikh
Publishing Product Manager: Reshma Raman
Senior Editor: Mohammed Yusuf Imaratwale
Content Development Editor: Sean Lobo
Technical Editor: Devanshi Deepak Ayare
Copy Editor: Safis Editing
Project Coordinator: Aparna Ravikumar Nair
Proofreader: Safis Editing
Indexer: Rekha Nair
Production Designer: Vijay Kamble
First published: August 2021
Production reference: 1150721
Published by Packt Publishing Ltd.
Livery Place
35 Livery Street
Birmingham
B3 2PB, UK.
ISBN 978-1-80056-550-0
www.packt.com
Contributors
About the author
Tyler Richards is a data scientist at Facebook, working on community integrity. Before this gig, his focus was on helping bolster the state of US elections for the nonprofit Protect Democracy. He is a data scientist and industrial engineer by training, which he gets to make use of in fun ways such as applying machine learning to local campus elections, creating algorithms to help P&G target Tide Pod users, and finding ways to determine the best ping pong players in friend groups. He is always looking for a new project, a new adventure.
About the reviewers
Randy Zwitch is head of developer relations at Streamlit. The developer relations team at Streamlit works with community members from around the world to help develop data apps and democratize decision-making across the enterprise. Randy is also a prolific open source contributor in the Python, Julia, and R communities. In his free time, Randy is an amateur luthier, building electric guitars and other stringed instruments at http://zwitchguitars.com/.
Weston Willingham studied industrial and systems engineering at the University of Florida before pivoting to data science. While completing the Galvanize Data Science Immersive program, Weston built several projects including a neural network for image detection and an audio transcriber trained to his own voice to improve presentation captioning. When not reading books, Weston can be found playing jazz piano and saxophone.
Preface
Data scientists and machine learning engineers throughout the 2010s have primarily produced static analyses. We create documents to inform decisions, filled with plots and metrics about our findings, or about the models we create. Creating complete web applications that allow users to interact with analyses is cumbersome, to say the least! Enter Streamlit, a Python library for creating web applications built with data folks in mind at every step.
Streamlit shortens the development time for the creation of data-focused web applications, allowing data scientists to create web app prototypes in Python in hours instead of days.
This book takes a hands-on approach to help you learn the tips and tricks that will have you up and running with Streamlit in no time. You'll start with the fundamentals of Streamlit by creating a basic app and gradually build on this foundation by producing high-quality graphics with data visualization and testing machine learning models. As you advance through the chapters, you'll walk through practical examples of both personal and work-related data-focused web applications, and will learn about more complicated topics such as using Streamlit Components, beautifying your apps, and the quick deployment of your new apps.