Chapter 1
Introduction
Changzhan Gu
1Department of Electronic Engineering, Shanghai Jiao Tong University, China
2Google LLC, USA
In the past 10 years, we are in the so-called mobile era where iOS, Android, and other smart devices have become the mobile computing platform that is deeply integrated into our lives. In recent years, owing to the advancement of computing, it is believed that the trend is shifting to a new era where artificial intelligence (AI) will become ubiquitous and unlock things that were unthinkable before [].
Since the debut of the modern computer, various techniques have been invented or developed as input technologies for our human beings to interact or control the functionality of a computing device. For decades, keyboard and mouse have probably been the most commonly used input devices. In gaming consoles like Sonys PlayStation or Microsofts Xbox, a remote controller might be hand-held to perform hand gestures. For smartphones that we use frequently on daily basis, the interaction happens by physically touching the screen. As technology advances, new input devices and approaches provide additional freedom and flexibility. A good example may be the wireless mouse that is free of the wire constrain. It captures hand actions like button clicks and movement positions, which is then wirelessly transmitted to the receiving computing device. The wireless nature gives the user more freedom with mobility and placement of the interacting device. Nevertheless, all the above-mentioned input devices require that the users must physically hold, touch, click, or move to enter inputs to the computing devices. What if the input devices get lost or misplaced? In that case, the users wont be able to enter inputs with that mechanism. The ubiquitous computing calls for additional freedom by removing the need for a physical device as the input mechanism. In the past few years, owing largely to the advancement of cloud computing, big data, and improved accuracy of speech recognition, digital voice assistants such as Apples Siri, Google Assistant, and Amazons Alexa are coming from fiction to our daily lives transforming the way we interact with the physical world around us. Devices integrated with voice assistant, e.g. Google Home or Amazon Eco, are believed to be the gateway that would control the vast array of Internet of things smart devices such as smart refrigerator, thermostats, light bulb, air conditioner, indoor/outdoor camera, TV, and game console.
No doubt that voice control is a natural and more advanced way to interact with the computing devices. Gesture control, on the other hand, is also very common. We human beings probably have been using hand gestures to interact with the physical world for thousands of years. It is deeply integrated in our lives. For example, while having a conversation at home, we might say can you pull the curtains its really dark while pointing at a window, or can you turn up the volume while pointing at a speaker; while answering people for directions, we might say go straight a mile and then turn right while pointing to that direction and performing the right turn gesture. Studies show that to interact with computing systems, people prefer a combination of speech and gesture over speech or gesture alone []. The multimodal interface combining both gesture and speech allows a more natural, intuitive, and intelligent way for HCI. The user may control the computing devices with their own choice of interaction that is the most natural and efficient way.
We humans have been using our hands as the natural tool to perform action gestures to interact with the physical world. The hand gesture is a simple and readily available mechanism for the user to input commands to the computing device. The small muscle groups in the twist and fingers can result in intuitive interaction by performing highly precise and controlled motions that allow for fluid, effective, and rapid manipulation. To capture the fine movement of the hand and fingers in the free space has not been an easy task. Attaching a movement sensing device to the hand doesnt remove the dependency on a peripheral device so it is not desirable. Alternatively, in the field of computer science, computer vision techniques have been developed to capture and identify hand gestures []. These new optical techniques provide depth information that can greatly improve precision. However, they have their inherent constraints. For example, they may not scale well to the small form factor or extra low power required by wearable and mobile devices. It should be noted that there is no input technology that is universally optimal for all computing devices and use cases. The choice of input technologies is not only dependent on the specific computing device but also always application driven, which involves difficult trade-offs between size, power consumption, sensing precision, sensor system bill of material cost, ease of integration to the computing device, sensitivity to light and environmental noise, impact as aggressor to neighboring sensors, and many other considerations.
Due to the technical advancements in semiconductor, signal processing, and system integration, radar has emerged as a promising technique for short-range motion sensingdetecting the objects relative displacement motions with very high accuracy. It is an alternative solution for noncontact gesture sensing as it transmits radio frequency signals to the target, and the targets movement can be extracted by analyzing the backscattering reflections []. Detecting the hands action gestures is a subset and a specific application of radar motion sensing. This book will cover the broad design phases of radar motion sensing, which includes radar sensor hardware, digital signal processing, and machine learning. The book aims to not only give the general audiences an introduction to short-range radar motion sensing but also provide researchers and professionals a glimpse of the latest advancements in the area.
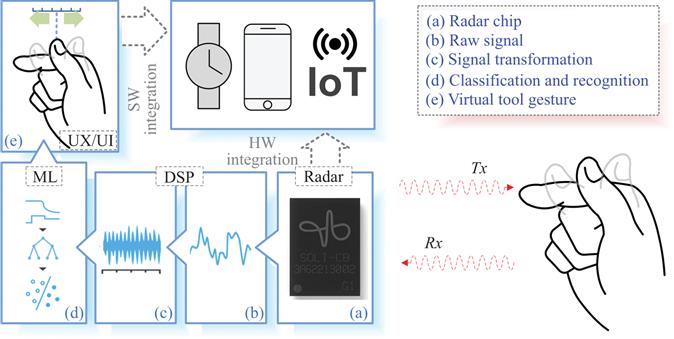
Figure 1.1 Google Soli: a complete end-to-end radar sensing system specifically designed for ubiquitous and intuitive gesture sensing []. ML machine learning; SDP digital signal processing; SW software; HW hardware
References
[1] D.M. West, and J.R. Allen How artificial intelligence is transforming the world, Available at: https://www.merantix.com/news-posts/how-artificial-intelligence-is-transforming-the-world/ (accessed on April 24, 2019).
[2] L. Dormehl, Thinking Machines: The Quest for Artificial Intelligenceand Where Its Taking Us Next (New York: PenguinTarcherPerigee, 2017).
[3] R. Rothe, Applying deep learning to real-world problems, Medium, May 23, 2017.
[4] M. Purdy, and P. Daugherty, Why artificial intelligence is the future of growth, Accenture, 2016.
[5] https://www.blog.google/products/assistant/personal-google-just-you/
[6] A.G. Hauptmann, Speech and gestures for graphic image manipulation, ACM SIGCHI, Bulletin, vol. 20, pp. 241245, 1989.
[7] J. Liu, and M. Kavakli, A survey of speech-hand gesture recognition for the development of multimodal interfaces in computer games, ICME, pp. 15641569, 2010.
[8] S.S. Rautaray, and A. Agrawal, Vision-based hand gesture recognition for human computer interaction: a survey, Artificial Intelligence Review, vol. 43, no. 1, pp. 154, 2015.
[9] T.B. Moeslund, A. Hilton, and V. Kruger, A survey of advances in vision-based human motion capture and analysis, Computer Vision and Image Understanding, vol. 104, no. 2, pp. 90126, 2006.
[10] J. Lien, N. Gillian, M.E. Karagozler, et al., Soli: Ubiquitous gesture sensing with millimeter wave radar, ACM Trans. Graph.,