Uday Kamath - Explainable Artificial Intelligence: An Introduction to Interpretable Machine Learning
Here you can read online Uday Kamath - Explainable Artificial Intelligence: An Introduction to Interpretable Machine Learning full text of the book (entire story) in english for free. Download pdf and epub, get meaning, cover and reviews about this ebook. publisher: Springer International Publishing, genre: Home and family. Description of the work, (preface) as well as reviews are available. Best literature library LitArk.com created for fans of good reading and offers a wide selection of genres:
Romance novel
Science fiction
Adventure
Detective
Science
History
Home and family
Prose
Art
Politics
Computer
Non-fiction
Religion
Business
Children
Humor
Choose a favorite category and find really read worthwhile books. Enjoy immersion in the world of imagination, feel the emotions of the characters or learn something new for yourself, make an fascinating discovery.
- Book:Explainable Artificial Intelligence: An Introduction to Interpretable Machine Learning
- Author:
- Publisher:Springer International Publishing
- Genre:
- Rating:3 / 5
- Favourites:Add to favourites
- Your mark:
- 60
- 1
- 2
- 3
- 4
- 5
Explainable Artificial Intelligence: An Introduction to Interpretable Machine Learning: summary, description and annotation
We offer to read an annotation, description, summary or preface (depends on what the author of the book "Explainable Artificial Intelligence: An Introduction to Interpretable Machine Learning" wrote himself). If you haven't found the necessary information about the book — write in the comments, we will try to find it.
Uday Kamath: author's other books
Who wrote Explainable Artificial Intelligence: An Introduction to Interpretable Machine Learning? Find out the surname, the name of the author of the book and a list of all author's works by series.
Explainable Artificial Intelligence: An Introduction to Interpretable Machine Learning — read online for free the complete book (whole text) full work
Below is the text of the book, divided by pages. System saving the place of the last page read, allows you to conveniently read the book "Explainable Artificial Intelligence: An Introduction to Interpretable Machine Learning" online for free, without having to search again every time where you left off. Put a bookmark, and you can go to the page where you finished reading at any time.
Font size:
Interval:
Bookmark:
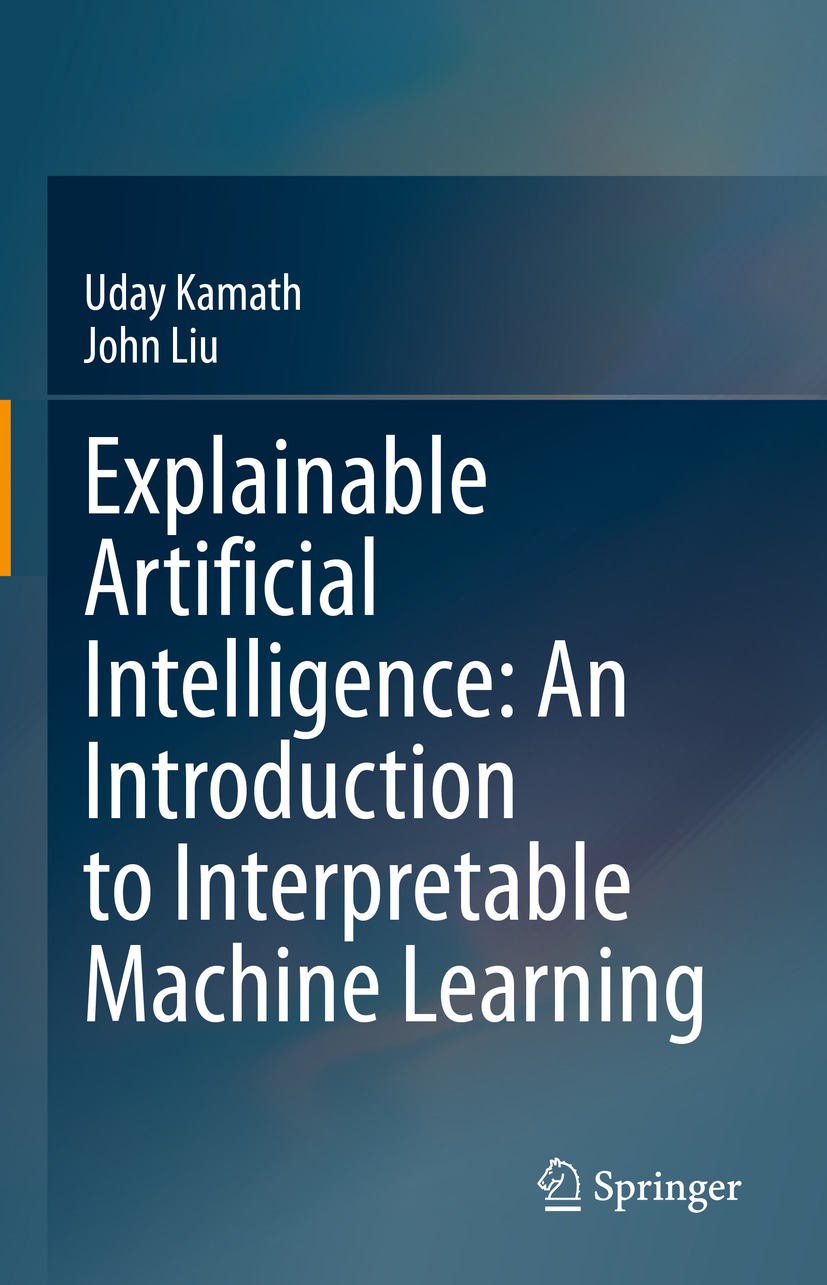
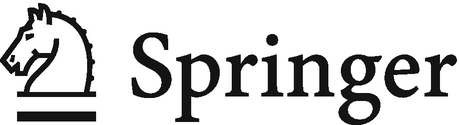
This Springer imprint is published by the registered company Springer Nature Switzerland AG
The registered company address is: Gewerbestrasse 11, 6330 Cham, Switzerland
To my parents Krishna and Bharathi, my wife Pratibha, the kids Aaroh and Brandy, my family and friends for their support.
Uday Kamath
To my wife Catherine and daughter Gabrielle Kaili-May and my parents for their encouragement and patience.
John Liu
The extraordinarily rapid integration of AI into many (if not most) aspects of life, entertainment, and business is transforming and disrupting the processes that power the flow of human experience. The pace of AI adoption everywhere is intense, accompanied by both immense benefits and immense risks. While many of the risks reside in the human, legal, social, and political dimensions of AI applications, the primary source of risk remains in the technical implementations of AI. Most importantly and significantly, the current technical risk landscape is populated by concerns around a taxonomy of AI issues: understandability, explainability, transparency, interpretability, trustworthiness, and more.
In this comprehensive book on explainable AI (XAI) by John Liu and Uday Kamath, we find a valuable and thorough handbook for the AI/ML community, including early learners, experienced practitioners, and researchers. The book covers the various dimensions of the AI technical risk taxonomy, including methods and applications. The result of the authors extensive work is an in-depth coverage of many XAI techniques, with real-world practical examples including code, libraries, algorithms, foundational mathematics, and thorough explanations (the essence of XAI).
The XAI techniques presented in-depth here range from traditional white-box (explainable) models (e.g., regression, rule-based, graphs, network models) to advanced black-box models (e.g., neural networks). In the first case, XAI is addressed through statistical and visualization techniques, feature exploration and engineering, and exploratory data analysis. In the latter case, XAI is addressed through a remarkably powerful and rich set of methods, including feature sensitivity testing through dependence, perturbation, difference, and gradient analyses. Extra attention is given to three special cases for XAI: natural language processing (NLP), computer vision (CV), and time series.
The book aims (and succeeds) to bring the reader up to date with the most modern advances in the XAI field, while also giving significant coverage to the history and complete details of traditional methods. In all the examples, use cases, techniques, and applications, the consistent theme of the book is to provide a comprehensive overview of XAI as perhaps the most critical requirement in AI/ML both for now and in the future, from both technical and compliance perspectives. In that regard, the forward-looking discussions at the end of the book give us a glimpse of emerging XAI research areas that will advance our AI risk-compliance posture, including human-machine collaboration (as in assisted and augmented intelligence applications), causal ML, explainable security, responsible AI, and multidisciplinary research into explainable and interpretable intelligence technologies as they impact humanity.
I expect that this book will be an essential textbook, guidebook, reference book, and how-to book in XAI design discussions, operational implementations, risk and compliance monitoring applications, and essential conversations with technically informed AI end-users, stakeholders, and regulators.
Kirk Borne, Ph.D., Data Scientist, Astrophysicist, Top Influencer, and Chief Science Officer at DataPrime.ai
The field of explainable AI addresses one of the most significant shortcomings of machine learning and deep learning algorithms today: the interpretability of models. As algorithms become more powerful and make predictions with better accuracy, it becomes increasingly important to understand how and why a prediction is made. Without interpretability and explainability, it would be difficult for the users to trust the predictions of real-life applications of AI. Interpretable machine learning and explainability is of critical importance for the following reasons:
We need interpretability to explain the models working from both the diagnosis and debugging perspective.
We need explanations for the end-user to explain the decisions made by the model and the rationale behind the decisions.
Most datasets or models have been shown to have biases, and investigating these biases is imperative for model deployment. Explainability is one way of uncovering these biases in the model.
Many industries such as finance and healthcare have legal requirements on transparency, trust, explainability, and faithfulness of models, thus making interpretability of models a prerequisite. In the European Union, some interpretations of the GDPR regulations claim that AI solutions must supply explanations for their conclusions. (Other interpretations say people need only be informed that automated processes are involved in decisions that may affect them.)
There is also a constant flux of new tools that fall in various categories such as application specific toolkits, visualization frameworks, and algorithm libraries. Python is currently the lingua-franca of data scientists and researchers to perform research in the area of interpretability and explainability. There are many libraries that have evolved in Python for interpretable machine learning and explainable AI in the last few years. We found a need for a single resource that gives concrete form to traditional as well as modern techniques in explainable AI through the use of existing tools and libraries for real-world applications. The work aims to be a comprehensive go to resource presenting the most important methods of explainability, insights to help put the techniques to use, and real-world examples with code for a hands-on experience.
Font size:
Interval:
Bookmark:
Similar books «Explainable Artificial Intelligence: An Introduction to Interpretable Machine Learning»
Look at similar books to Explainable Artificial Intelligence: An Introduction to Interpretable Machine Learning. We have selected literature similar in name and meaning in the hope of providing readers with more options to find new, interesting, not yet read works.
Discussion, reviews of the book Explainable Artificial Intelligence: An Introduction to Interpretable Machine Learning and just readers' own opinions. Leave your comments, write what you think about the work, its meaning or the main characters. Specify what exactly you liked and what you didn't like, and why you think so.