Patrick K. Lin
Copyright 2021 Patrick K. Lin
Whether AI will help us reach our aspirations or reinforce the unjust inequalities is ultimately up to us.
For every person who has ever been shortchanged, excluded, and underestimated by machines.
For the public interest technologists improving the world with their hope, vision, and perseverance.
For the activists and advocates protecting civil liberties through organizing, education, and dedication.
Introduction
Much of what New York City looks like today is attributed to a man who never held elected office or received any formal training in architecture or urban planning.
Robert Moses has been called the master builder of mid-twentieth century New York and its surrounding suburbs. He shaped much of the infrastructure of modern New York City, Long Island, Rockland County, and Westchester County (Caro, 1974).
Over the course of his forty-four-year career, Moses built nearly seven hundred miles of road, including massive highways that stretched out of Manhattan into Long Island and Upstate New York; twenty thousand acres of parkland and public beaches; 658 playgrounds; seven new bridges; the UN Headquarters; the Central Park Zoo; and the Lincoln Center for the Performing Arts (Burkeman, 2015). It would be an understatement to say Moses left a lasting mark on New York. In the twentieth century, the influence of Robert Moses on the cities of America was greater than that of any other person, wrote American historian Lewis Mumford.
However, new, large-scale developments come with a priceand not everyone pays the same amount.
To build hundreds of miles of highways and dozens of housing and urban renewal projects, Moses had more than five hundred thousand people evicted (Gratz, 2007). Black and Brown people comprised 40 percent of the evicted population at a time when those demographics made up only about 10 percent of the New York Citys overall population (Census Bureau, 2021). The construction of Lincoln Center alone displaced more than seven thousand working-class families and eight hundred businesses. Many of these evicted New Yorkers ended up in Harlem and the Bronx, further segregating the city (Williams, 2017). Moses also avoided building public pools in Black neighborhoods and instead designed those same neighborhoods to be prone to traffic congestion, not only withholding public goods from Black neighborhoods, but also forcing them to bear the brunt of the social costs (Schindler, 2015).
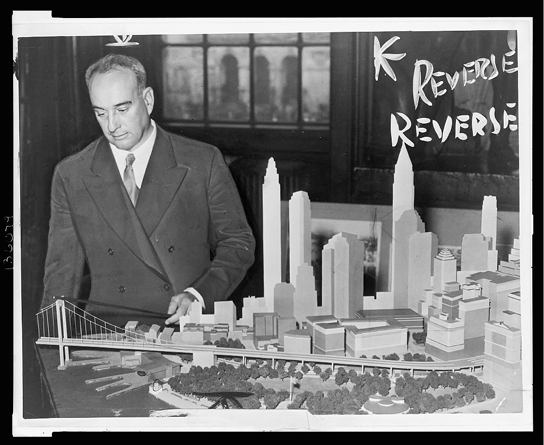
Robert Moses with a model of the proposed Battery Bridge. Source: The Library ofCongress.
Moses infamously hated the idea of poor peopleparticularly poor people of colorusing the new public parks and beaches he was building on Long Island (Burkeman, 2015). To that end, Moses used his influence and connections to pass a law forbidding public buses on highways, but he knew laws could someday be repealed. Legislation can always be changed, Moses said. Its very hard to tear down a bridge once its up. So Moses built scores of bridges that were too low to let public buses pass, literally concretizing discrimination (Bornstein, 2017). The effect of these decisions has been profound and enduring. Decades later, the bus laws Moses fought for were overturned. Still, the towns he built along the highways remain as segregated as ever.
People often do not want to believe seemingly innocuous objectslike bridges or highwayscan be racial or political, but as Moses buildings and plans show, human history is inherently racial and political. Moses racist views played out in what he built, how he built, and where he built.
But Moses was not alone. He wielded tremendous power and influence throughout his career, but he was still just an individual operating within a system built on bias and racism. For example, the Federal Housing Administrations Underwriting Manual states incompatible racial groups should not be permitted to live in the same communities, recommending highways be built as a way to separate Black neighborhoods from white neighborhoods (Gross, 2017). Rooting out bias isnt only about powerful individuals; it isnt even just about you or me. Its about history and systems that continue to exist, bridges that are too difficult to tear down.
Discriminatory decisions and policies of the past impact the present. Racial and social inequity affect the very fabric of our reality. Everything has costs and benefits, and these are not evenly distributed. The decision, whether conscious or unconscious, to advance or burden some members of society over others is fundamentally racial and political.
Artificial intelligence is no different. The technology is relentlessly improving and increasingly pervasive, yet despite well-documented biases, AI developed in the private and public sectors alike consistently fail to account for it. Somehow, in the past two decades, we got the idea machines make better decisions than humans. We began saying things like, People are biased, but AI will be more objective. We have forgotten humans design and deploy AI to serve their purposes. Humans, even those with the best intentions, can introduce bias to the AI they develop. Technology is not inherently objective or fair.
Todays technology, built from yesterdays data, will reflect the biased environment from which that data came. Bias often appears in AI systems through factors like race and gender, which generally are not directly inputted into the system but still have a strong influence over the systems decisions. The system is especially prone to bias when one of these factors is strongly correlated with information directly used by the system.
For example, suppose a system that makes determinations about someones level of education uses zip code as a factor to make its decisions. Direct information about race is never given to the system, so how can a system like that be biased?
Zip code is correlated with race since a lot of neighborhoods in America are still segregated, senior staff technologist Daniel Kahn Gillmor at the ACLUs Speech, Privacy, and Technology Project said to me. Gillmors work focuses on the way our technical infrastructure shapes society and impacts civil liberties. The data youre using to make these guesses is ultimately going to be pulled from a society that has a bunch of other problems, and the system is going to just reflect those problems back.
By using zip code as a factor, the AI system is indirectly making decisions based on race. In other words, zip code is a proxy for race. Therefore, even if the systems math and logic is all correct, an underlying ethical question reveals itself: is it appropriate to make these decisions based on these inputs?
A magical machine offering the promise of objectivity and fairness is extremely appealing. The public can be tricked into accepting an imperfect or even incompetent algorithm, particularly when the current state of an institution has historically been plagued by prejudice and bias, like the judicial system. We know things need to change and we want to believe technology can be that change. However, unlike humans, an algorithm cannot dissent, disobey, or make exceptions. People, on the other hand, can learn to account for ways in which data is a representation of the past.