
Anything in these pages that's politically incorrect is my fault alone. And for any content that might be inadvertently misinterpreted, I'm likely to blame. But for everything that came out right, I have many people to thank.
I'm forever thankful to my wonderful loving family, Mark, Jana, and Eva, for their encouragement and for giving me opportunities to learn, explore, and write. I give my deepest gratitude to journalist and author Paul Chutkow for his expert and selfless mentorship throughout the journey of this book. I'm also profoundly grateful to Francis Fukuyama for his invaluable support, and for being an inspiration as a scholar, a thinker, and a professor.
I'm especially thankful to Robert Mindelzun for sharing his lucid writing advice and his vast knowledge of medicine and history, to Glenn Lyons for reading through the entire manuscript and offering his insightful feedback, and to Cecilia Sedano for her tutoring in genetics and microbiology. I'm grateful to my agent Don Fehr for taking on a rookie with a challenging project, and to Steven L. Mitchell, Brian McMahon, Jill Maxick, Meghan Quinn, Melissa Shofner, Catherine Roberts-Abel, Jade Zora Scibilia, and the entire team at Prometheus Books.
For their reviews of technical sections and their help in accurately communicating their research, I owe a great debt to Jri Allik, Jeremy Bailenson, Sir Patrick Bateson, Alan Bittles, Thomas Bouchard, Carl-Johan Dalgaard, Richard Ebstein, Alejandro Feged-Rivadeneira, James Fowler, R. Chris Fraley, George Garratty, Chris Gonzalez, Barbara Harff, Weijing He, Agnar Helgason, Robert Hoshowsky, Hillard Kaplan, Kathleen Knight, Rodrigo Labouriau, Joris Lammers, Neil Van Leeuwen, Jason E. Lewis, Marco Lopez, Elainie Madsen, Nicholas G. Martin, Stewart McCann, Ola Olsson, Peter Parham, Geraint Rees, Peter Rentfrow, Camilo Andrs Rivera, Toms Rodrguez Barraquer, Gidi Rubinstein, David Sears, Robert Shapiro, William Shields, Michael Shifter, Kevin B. Smith, Randy Thornhill, Jacob Miguel Vigil, Alexander Weiss, Robin Weiss, Arthur Wolf, and Richie Zweigenhaft.
For sharing their research or their knowledge, I'm grateful to Daphna Canetti, Shirley Chen, David Cohen, Tlin Daloglu, Chengdiao Fan, Christopher Federico, Marcus Feldman, Israel Gershoni, Sandeep K. Giri, Arash Hazeghi, Miguel Hilario, James Jankowski, Matthew Jobin, Tim King, Charles Laidley, Noam Lupu, Rahul Madhavan, Jim Moore, Lee Nelson, Sven Oskarsson, Raj Patel, Mark Potok, Elly Power, Andrew T. Shepard, Paul M. Sniderman, Frank Sulloway, Sarah Tishkoff, Derya Tokdemir, Polly Weissner, and Vanessa Yorke.
I'd like to thank Lynore Banchoff, Tom Banchoff, Bruce Beron, Daniel Brehon, Yosem Companys, David Cotacachi, Larry Diamond, Linda Donley-Reid, Ben Dooley, Lusio Filiba, Diane Jordan Wexler, Kevin Mack, Moshe Malkin, Lynn Meisch, Moiss Nam, David Oestreicher, Vincent Philip, Zach Pogue, Jerrold M. Post, David Rubenstein, Lorena Sander, Atul Singh, and Robert Trivers for their encouragement on this project.
My appreciation goes to Paul Chutkow, Mary Bisbee-Beek, Jeannette Boudreau, Tyler Bridges, Blake Charlton, Yael Goldstein Love, Sara Houghteling, Don Jacobs, Daniel Mason, Bill Murphy Jr., and indexer Ellen Sherron for advising me on the publishing process. I thank coach Brian Callaghan, coach Allison Shapira, Roy Blitzer, Paul Rovner, Ceevah Sobel, Irwin Sobel, Sarah Sobel, and Joe Tuschman for their help with public speaking and presentations. For their advice on graphics and design, I'm grateful to Joan Hausman, Robert Kato, Larry Stueck, and Richard Tuschman; although I haven't been able to apply their advice in full, they're to thank for the absence of numerous indecipherable diagrams that I wrongly thought made sense.
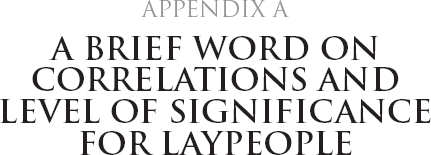
CORRELATIONS
So what exactly is a correlation? Correlations are the most common type of comparison that scholars of public opinion make. In fact, correlations help all kinds of scientists to identify relationships between the myriad interacting parts of our surroundings and ourselves. By taking measurements of different phenomena, we can begin to tease apart which elements change in relation to one another; doing so oftentimes enables us to see the world in a much more objective way than we might if left to our own biases.
It's easiest to illustrate the concept with a specific relationship. In the 1880s, Sir Francis Galton, who was the half-cousin of Charles Darwin, decided to study what determines adult height. He hypothesized that we inherit it from our parents. Galton found a correlation of 0.57 between the average height of parents and that of their offspring. What does 0.57 mean?
A perfect correlation is that of 1.0, which would have occurred if every child had grown to a height that was exactly proportional to the average height of his or her parents. In this case, every data point would fall on a straight line. A correlation of 0.0, on the other hand, would have occurred if one measurement had no linear predictive power whatsoever over the other; the graph would be a dispersed scattering of dots, with no linear trend. A perfect negative correlation of 1.0 would occur if people's stature were to decrease in a proportional manner to increases in their parents average height, and vice-versa, creating a straight line with a downhill slope.
As it turns out, Galton's correlation did not reach 1.0 because a number of other independent variables weakened the relationship; these may have included different levels of malnutrition and stress between the generations compared, misattributed paternity, acquired or genetic illnesses, maternal health and age during pregnancy, or the combination of the many height-influencing genes that happened to be inherited.
Thus, a correlation between two sets of measurements indicates the strength of the linear relationship between them when plotted on a graph. It's easiest to understand correlations visually (as in
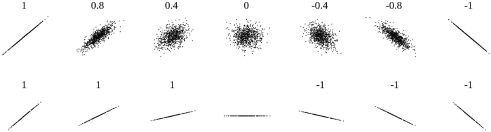
Figure 45. Correlation Coefficients and Graphs of the Two Variables Compared.
No explanation of correlations could be complete without the following warning: correlation does not prove causation! Consider this relationship: if an American individual disapproves of black-white dating, there is a good chance that this person approves of schools firing homosexual teachers. The correlation between these two attitudes, according to the Pew Research Center's 19872003 Values Survey, is 0.77.
But what does one view have to do with the other? It could be that homophobia directly causes disapproval of black-white dating (ethnocentrism); alternatively, maybe ethnocentrism is the direct cause of homophobia. Perhaps ethnocentrism indirectly brings about homophobia through some third factor. Or it could be that conservatism is the independent cause of all three factors. More often than not, especially in the social sciences, multiple factors correlate with the phenomenon of interest.
To avoid cluttering the pages with numbers, this book uses words to denote the strength of a correlation:
A very weak correlation is between 0.00 and 0.19
A weak correlation is between 0.20 and 0.39
A moderate correlation is between 0.40 and 0.59
A strong correlation is between 0.60 and 0.79
A very strong correlation is between 0.80 and 1.0
More specific information can be found in the original studies cited in the bibliographic notes.
Next page