The paper follows (1) trying in each case to explain the concept and its merits, before embarking on some controversial consequences and some urgent issues. What is the best way to implement NL interpretation is the best way to implement NL interpretation and the brain is also what it is. Therefore (a) and (b) will have to be settled by future research. (c) and (d) however can be seen as a thesis about the nature of NL interpretation. (c) and (d) commit one to a Bayesian analysis of Grices views on meaning and the repercussions of those analysis for the notion of rational NL interpretation. (a) and (b), and especially the characterisation they give of the problem of NL interpretation as finding ones way through massive ambiguity would give important support to this thesis. The more speculative (e) and (f) deal with a counterargument to the thesis, i.e. the argument that it cannot deal with the causal component of the thesis.
Computational BNLSP
Bayesian Interpretation is an important technique in the AI discipline of signal processing, especially in speech recognition and computer vision. Its basis is Bayes theorem, stated in (2). The theorem analyses the posterior probability of hypothesis H given a signal S , p ( H | S ) as the product of the prior probability of the hypothesis p ( H ) and the likelihood p (( S | H ) divided by the probability of the signal p ( S ). It essentially gives an alternative way to find p ( H | S ) if p ( H | S ) cannot be reliably measured in a direct way.
This is simply derived as follows:
Therefore

and
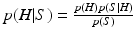
If S is given, the most probable H for a given S written
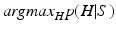
can be computed in a different way given Bayes theorem.

, since

if S is given. If one is searching for the most probable interpretation H of a signal S , it will therefore suffice to have a good estimation of the probability of H (called the prior, the probability assigned to H before the signal occurred) and of the probability p ( S | H ) (the likelihood of the signal given the interpretation). This way of finding the most probable H is useful and often unavoidable, since it can be difficult or even impossible to directly estimate p ( H | S ) (the posterior probability of the interpretation H , after the occurrence of the signal S ).
The difficulty for direct estimation is especially clear when the set of signals is infinite or continuous, as in speech perception or in vision. For example, in speech perception, one measures the distance between the perceived signal and the predicted signal to estimate likelihood and uses the prediction for the next phoneme from the preceding phoneme sequence obtained from a large body of written text to estimate the prior.
The definition just given makes BNLI a special way of obtaining the most probable interpretation of an utterance and BNLI is thereby a branch of stochastic signal interpretation.
One needs a good argument for using Bayesian interpretation rather than the direct method of estimating the posterior. And one needs to accept that natural language is ambiguous to go stochastic in the first place.
The method by itself does not entail that both likelihood and prior need to be estimated stochastically. The prior can be partly computed by symbolic techniques, e.g. by a theorem prover showing that the prior is not 0 by showing the consistency of the interpretation with the context. Similarly, one can use symbolic techniques (e.g. a text generator in NLIthese are standardly symbolic) to estimate likelihood.
Doing NLI or NLSP with this technique makes BNLSP a branch of stochastic NLSP. Stochastic NLSP obviously helps in obtaining good results in interpretation, since NL utterances severely underdetermine their interpretations in context and other techniques for disambiguation are limited in scope. It is also easy to see that very often disambiguation cannot be explained by an appeal to hard facts and logic, but needs to appeal to what is probable in the world. For example, if I tell you that I took the bus, you will understand me as having used the public transport system and not as having lifted an autobus with my crane. Another basic example is (3).
If you are like me, Tweety is the bird from AI discussions about default reasoning and flies means can fly using his wings. There must be many other Tweeties however and there is no logical reason why the verbal form could not mean uses aeroplanes or can pilot aeroplanes.