CHAPTER 1: Introducing Julia

There are dozens of programming languages out there: some generic, some focused on specific niches, each claiming to be better than the rest. The most powerful languagesthe ones capable of rapidly performing complex calculationstend to be difficult to learn (and even more difficult to master). Their audience is thus limited to those hardcore programmers who usually have some innate talent for this kind of work. The aspiring data scientists are faced with the prospect of devoting considerable time and energy to learning a language that will ultimately serve them poorly, requiring line after line of complex code to implement any useful algorithm.
On the other side of the spectrum are the plug-and-play languages, where all the complexities of programming have been carefully encapsulated. The most tedious (and often most widely-utilized) algorithms have been pre-packaged and handed over to the user; theres very little to actually learn. The problem with these languages is that they tend to be slow, with severe limitations in memory and capability. The data scientist here faces the opposite dilemma: take advantage of a language without a steep learning curve, but be stuck with a fairly weak tool for the job.
Julia is one of the languages that lies somewhere between these two extremes, offering the best of both worlds. In essence, it is a programming language designed for technical computing, offering speed, ease of use, and a variety of tools for data processing. Even though its still in its infancy (version 1.0 is still pending and is expected to be released summer 2017), those who have toyed with it enough to recognize its potential are already convinced of its utility in technical computing and data science applications.
Some of the features that make Julia stand out from other programming languages include:
- Julia exhibits incredible performance in a variety of data analyses and other programming ventures. Its performance is comparable to that of C, which is often used as a benchmark for speed.
- Julia has a strong base library , allowing for all kinds of linear algebra operations that are often essential components of data analytics moduleswithout the need to employ other platforms.
- Julia utilizes a multiple dispatch style, giving it the ability to link different processes to the same function. This makes it easy to extend existing functions and reuse them for different types of inputs .
- Julia is easy to pick up , especially if you are migrating to it from Python, R, or Matlab/Octave.
- Julia has a variety of user-friendly interfacesboth locally and on the cloud that make it an enjoyable interactive tool for all kinds of processes. It features a handy help for all functions and types.
- Julia seamlessly connects with other languages, including (but not limited to) R, Python, and C. This makes it easy to use with your existing code-base, without the need for a full migration.
- Julia and all of its documentation and tutorials are open-source, easily accessible, detailed, and comprehensible.
- Julias creators are committed to enhancing it and helping its users. They give a variety of talks, organize an annual conference, and provide consulting services.
- Julias custom functions are as fast and compact as those built into the base Julia code.
- Julia has excellent parallelization capabilities , making it easy to deploy over various cores of your computer or a whole cluster of computers.
- Julia is exceptionally flexible for developing new programs, accommodating both novice and expert users with a wide range of coding levels. This feature is rare in other languages.
As you learn and practice with Julia, youll certainly uncover many more advantages particular to the data science environment.
How Julia Improves Data Science
Data science is a rather ambiguous term that has come to mean a lot of different things since its introduction as a scientific field. In this book we will define it as: the field of science dealing with the transformation of data into useful information (or insights) by means of a variety of statistical and machine learning techniques.
Since reaching critical mass, data science has employed all kinds of tools to harness the power and overcome the challenges of big data. Since a big part of the data science process involves running scripts on large and complex datasets (usually referred to as data streams), a high performance programming language is not just a luxury but a necessity.
Consider a certain data-processing algorithm that takes several hours to run with a conventional language: even a moderate increase in performance will have a substantial impact on the overall speed of the process. As a language, Julia does exactly that. This makes it an ideal tool for data science applications, both for the experienced and the beginner data scientist.
Data science workflow
People view data science as a process comprising various parts, each intimately connected to the data at hand and the objective of the analysis involved. More often than not, this objective involves the development of a dashboard or some clever visual (oftentimes interactive), which is usually referred to as a data product.
Data science involves acquiring data from the world (from data streams stored in HDFS, datasets in CSV files, or data organized in relational databases), processing it in a way that renders useful information, and returning this information to the world in a refined and actionable form. The final product usually takes the form of a data product, but this is not essential. For instance, you may be asked to perform data science functions on a companys internal data and keep the results limited to visuals that you share with your manager.
Take for example a small company that is opting for data-driven market research, through the use of questionnaires for the subscribers to their blog. Data science involves these five steps:
- Acquisition of the data from the marketing team
- Preparation of the data into a form that can be usable for predictive analytics
- Exploratory analysis of the data to decipher whether certain people are more inclined to buy certain products
- Formatting of work to make the whole process resource-efficient and error-free
- Development of models that provide useful insights about what products the companys clients are most interested in, and how much they are expected to pay for them.
We will go into more detail about this process in Chapter 5.
Figure 1.1 introduces the big picture of data science processes and how the Julia language fits in. The three stacked circles have come to represent Julia in general; in the figure, this symbol indicates a practical place to utilize Julia. It is clear that apart from the development of the data product and the acquisition of the data, Julia can be used in almost every phase of the data science process.
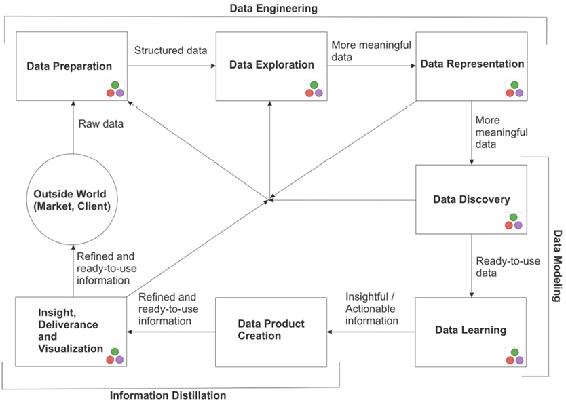
Figure 1.1 The data science process overview. The symbol with three stacked circles indicates steps where Julia fits in.
Consider how much this will simplify your workflow. No need to stitch code from other platforms into your pipeline, creating troublesome bottlenecks. Furthermore, once you have written and tested your code in Julia there is no need to translate it into a low-level language like C++ or Java, since there is no performance benefit from doing this. Thats particularly important considering that this is an essential step when prototyping in languages like R and Matlab.