Akinori Tanaka - Deep Learning and Physics
Here you can read online Akinori Tanaka - Deep Learning and Physics full text of the book (entire story) in english for free. Download pdf and epub, get meaning, cover and reviews about this ebook. year: 2021, publisher: Springer Singapore, genre: Children. Description of the work, (preface) as well as reviews are available. Best literature library LitArk.com created for fans of good reading and offers a wide selection of genres:
Romance novel
Science fiction
Adventure
Detective
Science
History
Home and family
Prose
Art
Politics
Computer
Non-fiction
Religion
Business
Children
Humor
Choose a favorite category and find really read worthwhile books. Enjoy immersion in the world of imagination, feel the emotions of the characters or learn something new for yourself, make an fascinating discovery.
- Book:Deep Learning and Physics
- Author:
- Publisher:Springer Singapore
- Genre:
- Year:2021
- Rating:5 / 5
- Favourites:Add to favourites
- Your mark:
- 100
- 1
- 2
- 3
- 4
- 5
Deep Learning and Physics: summary, description and annotation
We offer to read an annotation, description, summary or preface (depends on what the author of the book "Deep Learning and Physics" wrote himself). If you haven't found the necessary information about the book — write in the comments, we will try to find it.
Deep Learning and Physics — read online for free the complete book (whole text) full work
Below is the text of the book, divided by pages. System saving the place of the last page read, allows you to conveniently read the book "Deep Learning and Physics" online for free, without having to search again every time where you left off. Put a bookmark, and you can go to the page where you finished reading at any time.
Font size:
Interval:
Bookmark:
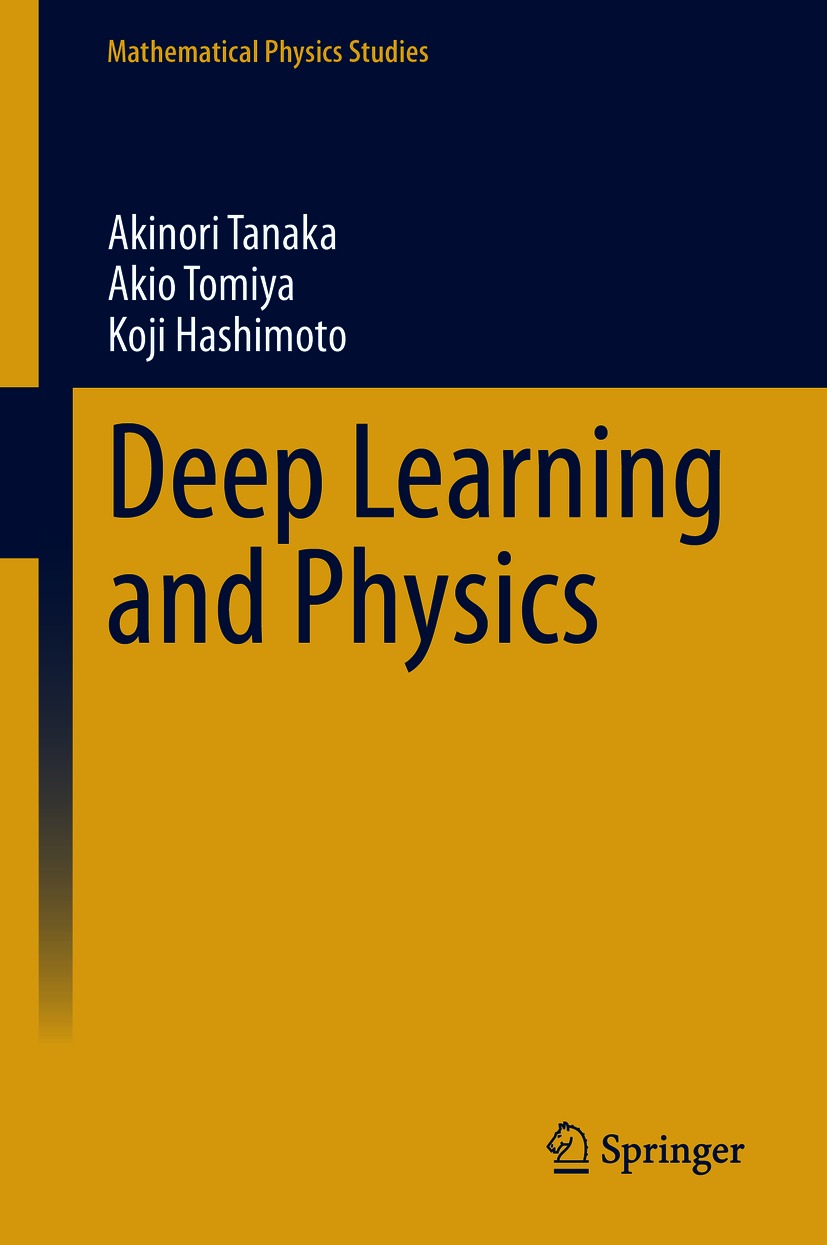
The series publishes original research monographs on contemporary mathematical physics. The focus is on important recent developments at the interface of Mathematics, and Mathematical and Theoretical Physics. These will include, but are not restricted to: application of algebraic geometry, D-modules and symplectic geometry, category theory, number theory, low-dimensional topology, mirror symmetry, string theory, quantum field theory, noncommutative geometry, operator algebras, functional analysis, spectral theory, and probability theory.
More information about this series at http://www.springer.com/series/6316
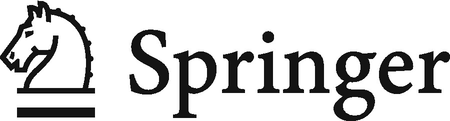
This Springer imprint is published by the registered company Springer Nature Singapore Pte Ltd.
The registered company address is: 152 Beach Road, #21-01/04 Gateway East, Singapore 189721, Singapore
To readers traveling beyond the boundaries of knowledge
What is deep learning for those who want to study physics? Is it completely different from physics? Or is it really similar?
In recent years, machine learning, including deep learning, has begun to be used in various physics studies. Why is that? Is knowing physics useful in machine learning? Conversely, is knowing machine learning useful in physics?
This book is devoted to answers of these questions. Starting with basic ideas of physics, neural networks are derived naturally. And you can learn the concepts of deep learning through the words of physics.
In fact, the foundation of machine learning can be attributed to physical concepts. Hamiltonians that determine physical systems characterize various machine learning structures. Statistical physics given by Hamiltonians defines machine learning by neural networks. Furthermore, solving inverse problems in physics through machine learning and generalization essentially provides progress and even revolutions in physics. For these reasons, in recent years, interdisciplinary research in machine learning and physics has been expanding dramatically.
This book is written for anyone who wants to know, learn, and apply the relationship between deep learning/machine learning and physics. The concepts of statistical mechanics and the bracket notation of quantum mechanics, which are introduced in columns, are used to explain deep learning frameworks.
This book is divided into two parts. The first part concerns understanding the machine learning method from the perspective of physics, and the second part explores the relationship between various problems in physics and the method of machine learning. That is, the first part is written as a textbook, but the second part is not a text book, it is a collection of recent advanced topics (and so, the chapters in the second part can be read almost independently). Please refer to the readers guide at the beginning of each section.
A renowned physicist, Ryoyu Uchiyama, said in the preface to his book, Theory of Relativity [1], If you read this book and do not understand it, you should give up learning the theory of relativity. On the other hand, the field of machine learning physics, which is the subject of this book, is not a discipline established over a long history like the theory of relativity, but a field of research that is still undergoing great progress. So, rather, our message is this: If you read this book and do not understand it, it will be a seed for development in the future.
One of our favorites is the words by S. Weinberg, a particle physicist [2]:
My advice is to go for the messes thats where the action is.
It is up to the readers to make their own way. We hope this book helps the readers learn and study.
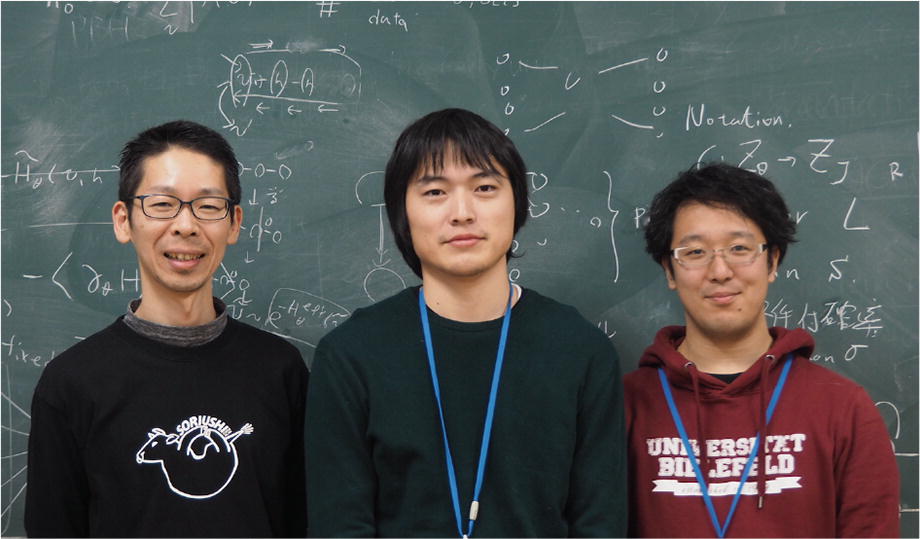
The authors
This book was written based on discussions about physics and machine learning with many people around us. Particularly essential were the discussions at the symposia Deep Learning and Physics and Deep Learning and Physics 2018 held at Osaka University, as well as at research meetings and seminars around the world. We would like to take this opportunity to thank all those involved. We thank Tetsuya Akutagawa, Naoyuki Sumimoto, Norihito Shirai, Yuki Nagai, Yuta Kikuchi, Shuji Kitora, and Sayaka Sone for reading and commenting on the Japanese draft. Akinori Tanaka gratefully acknowledges support from the RIKEN Center for Advanced Intelligence Project and RIKEN Interdisciplinary Theoretical and Mathematical Sciences Program (iTHEMS). Akio Tomiya thanks Prof. Heng-Tong Ding at Central China Normal University. If he had not hired Akio as a postdoc and given Akio an environment to freely study issues which are different from his profession, the papers that sparked this book would not have been possible. Akio would like to thank Prof. Makoto Matsumoto at Hiroshima University for pointing out the relationship between true random numbers and the Kolmogorov complexity. Koji Hashimoto thanks his wife, Haruko Hashimoto, who understands writing and research well. We all thank Haruko Hashimoto for her support on English translation and Mr. Atsushi Keiyama, an editor at Kodansha Scientific, for his effort on the publication of the Japanese version of this book (published in June 2019). We are grateful to Prof. Hirosi Ooguri and Prof. Masatoshi Imada who kindly supported the publication of this book at Springer, and also to Mr. Nakamura for his editorial work on this book. Finally, we would like to thank the researchers from all over the world for discussing physics and machine learning at various locations.
Font size:
Interval:
Bookmark:
Similar books «Deep Learning and Physics»
Look at similar books to Deep Learning and Physics. We have selected literature similar in name and meaning in the hope of providing readers with more options to find new, interesting, not yet read works.
Discussion, reviews of the book Deep Learning and Physics and just readers' own opinions. Leave your comments, write what you think about the work, its meaning or the main characters. Specify what exactly you liked and what you didn't like, and why you think so.