Editors
Jenny Benois-Pineau and Akka Zemmari
Multi-faceted Deep Learning
Models and Data
1st ed. 2021
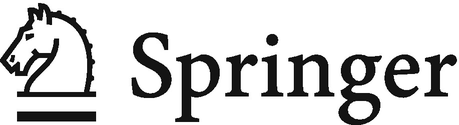
Logo of the publisher
Editors
Jenny Benois-Pineau
LaBRI UMR 5800, University of Bordeaux, Talence Cedex, France
Akka Zemmari
LaBRI UMR 5800, University of Bordeaux, Talence Cedex, France
ISBN 978-3-030-74477-9 e-ISBN 978-3-030-74478-6
https://doi.org/10.1007/978-3-030-74478-6
Springer Nature Switzerland AG 2021
This work is subject to copyright. All rights are reserved by the Publisher, whether the whole or part of the material is concerned, specifically the rights of translation, reprinting, reuse of illustrations, recitation, broadcasting, reproduction on microfilms or in any other physical way, and transmission or information storage and retrieval, electronic adaptation, computer software, or by similar or dissimilar methodology now known or hereafter developed.
The use of general descriptive names, registered names, trademarks, service marks, etc. in this publication does not imply, even in the absence of a specific statement, that such names are exempt from the relevant protective laws and regulations and therefore free for general use.
The publisher, the authors and the editors are safe to assume that the advice and information in this book are believed to be true and accurate at the date of publication. Neither the publisher nor the authors or the editors give a warranty, expressed or implied, with respect to the material contained herein or for any errors or omissions that may have been made. The publisher remains neutral with regard to jurisdictional claims in published maps and institutional affiliations.
This Springer imprint is published by the registered company Springer Nature Switzerland AG
The registered company address is: Gewerbestrasse 11, 6330 Cham, Switzerland
And now, if you will set us to our task,
We will serve you four and twenty hours a day...
Rudyard Kipling, The secret of machines
We dedicate this book to our students.
Be curious, be inventive, persevere and
serve the Dame Science!
Preface
Today, artificial intelligence approaches penetrate all areas of societal activity. One of its main branches, artificial neural networks, got a new life with the drastic augmentation of computational capacities due to graphical processing units and cloud computing. Neural networks have become deep. Deep learning is now a winner in all supervised machine learning approaches which have been ever used for data mining and decision-making.
These tools are specifically interesting in the field which has been traditionally called multimedia. Indeed, this field supplies a huge amount of heterogeneous data: images, video, audio and music, text, and multimodal signals. Furthermore, these data have a spatio-temporal grid structure which is convenient for one of the varieties of deep learning networks, such as convolutional neural networks.
Hence, in this book, we tried to provide a snapshot of methods, models, and data which are being developed or used in this research community. This book is a collective work of selected researchers at the French National Network GDR-ISIS and ACM Special Interest Group on Multimedia. We hope this book will be interesting for young researchers, student, and professionals who are employing existing models and designing new ones in the framework of deep learning.
Jenny Benois-Pineau
Akka Zemmari
Bordeaux, France
December 2020
Acknowledgments
The editors of this book acknowledge the French National Research Network CNRS GDR-ISIS and also ACM-SIGMM which enabled the authors of this book to collaborate together.
Contents
Jenny Benois-Pineau
Akka Zemmari and Jenny Benois-Pineau
Alexandre Benoit , Badih Ghattas , Emna Amri , Joris Fournel and Patrick Lambert
Nicolas Thome
Stefan Duffner , Christophe Garcia , Khalid Idrissi and Atilla Baskurt
Yannick Le Cacheux , Herv Le Borgne and Michel Crucianu
Danny Francis and Benoit Huet
Ofer Hadar and Raz Birman
Pierre-Etienne Martin , Jenny Benois-Pineau , Renaud Pteri , Akka Zemmari and Julien Morlier
Geoffroy Peeters and Gal Richard
Pascal Bourdon , Olfa Ben Ahmed , Thierry Urruty , Khalifa Djemal and Christine Fernandez-Maloigne
Leonardo Galteri , Lorenzo Seidenari , Tiberio Uricchio , Marco Bertini and Alberto del Bimbo
Jenny Benois-Pineau and Akka Zemmari
Springer Nature Switzerland AG 2021
J. Benois-Pineau, A. Zemmari (eds.) Multi-faceted Deep Learning https://doi.org/10.1007/978-3-030-74478-6_1
1. Introduction
Jenny Benois-Pineau
(1)
LaBRI UMR 5800, University of Bordeaux, Talence Cedex, France
Jenny Benois-Pineau
Email:
Artificial Intelligence (AI), and particularly Deep Learning, is changing our daily life. In last few years, this domain gained much interest both from theoretical and practical point of views. AI based solutions are deployed in many applications and are used in many fields. This includes financial applications, security, trading, autonomous vehicles, etc.
Deep Learning gained very high interest with the huge amount of available data and the new computation capabilities. It benefits also from a long research activities on neural networks started by the first works of W. McCulloch and W. Pitts on 1943 who first defined the formal neuron and crowned by the award of the Turing prize to Y. LeCun, Y. Bengio and G. Hinton in 2018.
In this book, we present most popular Artificial Intelligence methods for data mining of nowadays form multifaceted perspective: in problems, methods and data. The book gives a rich overview of ongoing research in the community and is written by the first rank international researchers.
The book starts by introducing the design and implementation of various architectures for Deep Learning, together with optimization algorithms. It discusses the most state-of-the-art networks, such as Artificial Neural Networks, Convolutional Neural Networks and Recurrent Networks. Then it presents some other models like Generative Neural Networks, Autoencoders and Siamese CNNs.
As a first application of Deep Learning methods, we consider its use for semantic segmentation. A chapter reviews the image semantic segmentation task and recent advanced strategies to face typical training issues (few training samples, specific data, strong target imbalance, ) in a variety of application domains. Another chapter considers image and video captioning using deep learning. It aims at giving insights on how to generate descriptive sentences from images and videos. A third application investigates the use of the 3D Convolutional Neural Networks for action recognition with application to sport gesture recognition.
As mentioned above, the impressive success of Deep Learning is due to the huge amount of available data. However, for supervised learning, this data have to be labeled. In a dedicated chapter, we present solutions based on three families of methods for learning with less expensive labelling. We detail an approach based on these methods applied for using incomplete annotations for medical image segmentation.