Volume 12647
Lecture Notes in Computer Science Information Systems and Applications, incl. Internet/Web, and HCI
Editorial Board
Elisa Bertino
Purdue University, West Lafayette, IN, USA
Wen Gao
Peking University, Beijing, China
Bernhard Steffen
TU Dortmund University, Dortmund, Germany
Gerhard Woeginger
RWTH Aachen, Aachen, Germany
Moti Yung
Columbia University, New York, NY, USA
Founding Editors
Gerhard Goos
Karlsruhe Institute of Technology, Karlsruhe, Germany
Juris Hartmanis
Cornell University, Ithaca, NY, USA
More information about this subseries at http://www.palgrave.com/gp/series/7409 The series Lecture Notes in Computer Science (LNCS), including its subseries Lecture Notes in Artificial Intelligence (LNAI) and Lecture Notes in Bioinformatics (LNBI), has established itself as a medium for the publication of new developments in computer science and information technology research and teaching.
The cornerstone of LNCS's editorial policy is its unwavering commitment to report the latest results from all areas of computer science and information technology research, development, and education. LNCS has always enjoyed close cooperation with the computer science R & D community, with numerous renowned academics, and with prestigious institutes and learned societies. Our mission is to serve this community by providing a most valuable publication service.
LNCS commenced publication in 1973 and quite rapidly attracted attention, not least because of its thus far unprecedented publication turnaround times. The 1980s and 1990s witnessed a substantial growth in the series, particularly in terms of volumes published. In the late 1990s we developed a systematic approach to providing LNCS in a full-text electronic version, in parallel to the printed books. Another new feature introduced in the late 1990s was the conceptualization of a couple of color-cover sublines. Still, original research results reported in proceedings and postproceedings remain the core of LNCS.
Editors
Yan Jia , Zhaoquan Gu and Aiping Li
MDATA: A New Knowledge Representation Model
Theory, Methods and Applications
1st ed. 2021
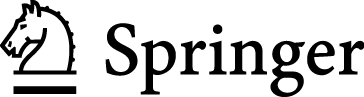
Logo of the publisher
Editors
Yan Jia
Harbin Institute of Technology, Shenzhen, Shenzhen, China
Zhaoquan Gu
Guangzhou University, Guangzhou, China
Aiping Li
National University of Defense Technology, Changsha, China
ISSN 0302-9743 e-ISSN 1611-3349
Lecture Notes in Computer Science Information Systems and Applications, incl. Internet/Web, and HCI
ISBN 978-3-030-71589-2 e-ISBN 978-3-030-71590-8
https://doi.org/10.1007/978-3-030-71590-8
Springer Nature Switzerland AG 2021
This work is subject to copyright. All rights are reserved by the Publisher, whether the whole or part of the material is concerned, specifically the rights of translation, reprinting, reuse of illustrations, recitation, broadcasting, reproduction on microfilms or in any other physical way, and transmission or information storage and retrieval, electronic adaptation, computer software, or by similar or dissimilar methodology now known or hereafter developed.
The use of general descriptive names, registered names, trademarks, service marks, etc. in this publication does not imply, even in the absence of a specific statement, that such names are exempt from the relevant protective laws and regulations and therefore free for general use.
The publisher, the authors and the editors are safe to assume that the advice and information in this book are believed to be true and accurate at the date of publication. Neither the publisher nor the authors or the editors give a warranty, expressed or implied, with respect to the material contained herein or for any errors or omissions that may have been made. The publisher remains neutral with regard to jurisdictional claims in published maps and institutional affiliations.
This Springer imprint is published by the registered company Springer Nature Switzerland AG
The registered company address is: Gewerbestrasse 11, 6330 Cham, Switzerland
Preface
With the fast development of information technologies, many researchers are dedicated to knowledge representation, a high-level understanding and abstraction of massive data. Although many knowledge representation models have been proposed, such as symbolic logic models, semantic networks, expert systems, semantic webs, and knowledge graphs, these models cannot represent procedural knowledge, i.e. dynamic knowledge that changes with temporal and spatial factors.
In this edited book, we introduce a new knowledge representation model called MDATA ( M ulti-dimensional D ata A ssociation and in T elligent A nalysis). After investigating a large number of related works in knowledge representation and cognitive models, we propose the MDATA model on the basis of the widely adopted knowledge graphs. By modifying the representation of relations and properties in knowledge graphs, dynamic knowledge can be efficiently described with temporal and spatial characteristics. The MDATA model can be regarded as a high-level temporal and spatial knowledge graph model which has strong capabilities for knowledge representation.
Similar as knowledge graphs, there are many key technologies in the MDATA model, such as entity recognition, relation extraction, property extraction, etc. The MDATA model pays particular attention to temporal and spatial characteristics, and the recognition of such temporal and spatial characteristics is important. These characteristics could be time period, location, or even IP address. In order to improve the knowledge graph, knowledge reasoning and completion are necessary, such as entity reasoning and relation completion. In the MDATA model, the dynamic knowledge should also be updated by reasoning, which combines the temporal and spatial factors. As many existing technologies have been proposed in constructing knowledge graphs, we need to extend them to the MDATA model.
The MDATA model can represent knowledge from different dimensions and it can be applied in many critical applications. The outbreak of the coronavirus pandemic has caused serious consequences. By representing personal knowledge such as dynamic travel information by the MDATA model, we can identify close contacts of infected persons in an efficient way. Considering network attacks that might happen every day around the world, the MDATA model can combine the analysis of massive data from log or network traffic to detect attack events. For social network analysis, in which information updates quickly and peoples opinion might change, the MDATA model can represent such dynamic knowledge for better analysis, such as computing individual influence, community detection and sentiment analysis.
To promote the MDATA model, we have brought together research teams from many universities, research institutes, and companies, such as the Harbin Institute of Technology, Shenzhen (China), Guangzhou University (China), National University of Defense Technology (China), Zhejiang University of Technology (China), Hangzhou Dianzi University (China), The University of Queensland (Australia), The University of New South Wales (Australia), etc. We have applied the MDATA model in many practical applications: for example, we have developed a cyberspace security situation awareness system that can detect various network attacks in a timely way. We have also organized many seminars and workshops to exchange the latest research, such as the MDATA workshop in the IEEE International Conference on Data Science in Cyberspace. We hope the MDATA model will be widely adopted in various areas and can promote the study of knowledge representation significantly.