De Gruyter Textbook
EBSCOhost - printed on 2/14/2022 11:29 AM via . All use subject to https://www.ebsco.com/terms-of-use
De Gruyter Textbook
EBSCOhost - printed on 2/14/2022 11:29 AM via . All use subject to https://www.ebsco.com/terms-of-use
ISBN 9783110629392
e-ISBN (PDF) 9783110629453
e-ISBN (EPUB) 9783110630534
Bibliographic information published by the Deutsche Nationalbibliothek
The Deutsche Nationalbibliothek lists this publication in the Deutsche Nationalbibliografie; detailed bibliographic data are available on the Internet at http://dnb.dnb.de.
2021 Walter de Gruyter GmbH, Berlin/Boston
EBSCOhost - printed on 2/14/2022 11:29 AM via . All use subject to https://www.ebsco.com/terms-of-use
Data science: introduction
Data science [a] is an interdisciplinary field that usesscientificmethods, processes, algorithms, and systems toextract knowledgeand insights from structural and unstructured data. Data science is related to data mining and big data.
The keyword in Data Science is not Data, it is Science [a]
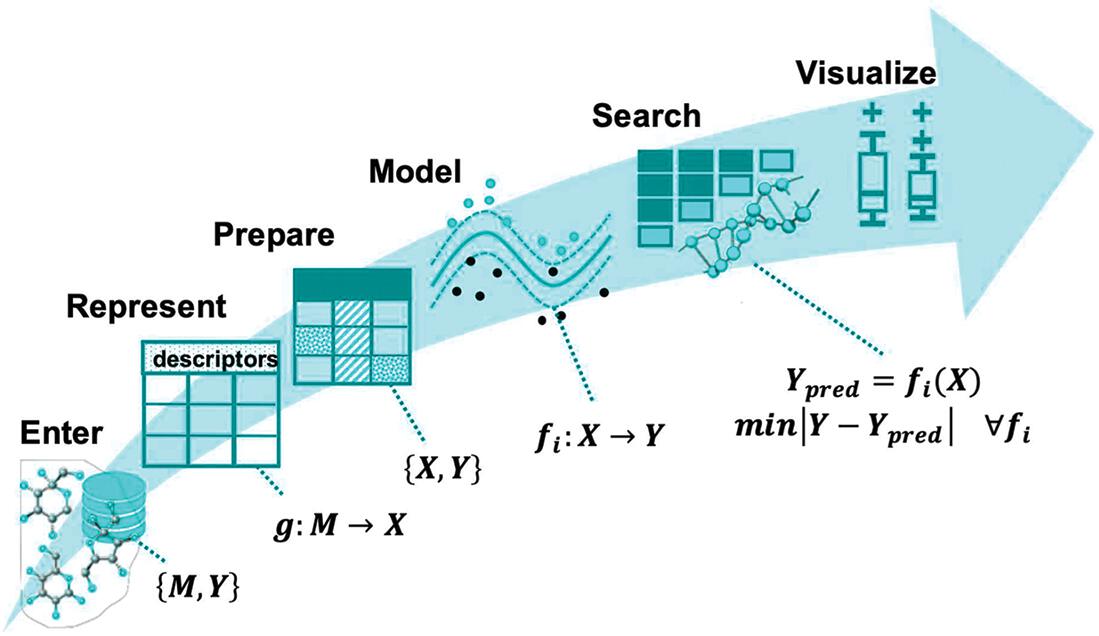
Figure 1.1: The major tasks and mathematical setup of a supervised machine learning workflow3 (Hachmann).
The first problem is that most great data scientists dont sufficiently understand business and most great business leaders dont sufficiently understand data science. [a]
Relation of science and digital research
Since the beginning of the 1980s, the term data science has appeared in various contexts, but was never well defined in the scientific community. [b]
Definitions
Data science is performed to analyze, understand, and extract actual phenomena in data. The challenge is to identify unique patterns and variables.
The goal is to:
understand
extract insights
To do this, data science is a multidisciplinary field that brings together concepts from:
Bringing together these fields of expertise in data science is also a concept of unification.
Discussion
Leek [b] discusses the relation of structure and the desired results:
It is easy to discover structure or networks in a data set. There will always be correlations for a thousand reasons if you collect enough data.
Understanding whether these correlations matter for specific, interesting questions is much harder.
Often the structure you found on the first pass is due to phenomena (measurement error, artifacts, and data processing) that do not answer an interesting question.
The two paradigms of data research
Hypothesis driven
Given a problem, what kind
of data do we need to help solve it?
Data driven
Given some data, what interesting
problems can be solved with it?
The heart of data science is to always ask questions:
What can we learn from this data?
What actions can we take, once we find whatever it is we are looking for?
Main types of problems
Two problems arise repeatedly in data science. This is discussed in detail in Chapters 33 to 37. As a rule of thumb, these are:
References
Data science (Wikipedia)
Leek, J. Simply Statistics
Haghighatlari, M.; Hachmann, J. Advances of Machine Learning in Molecular Modeling and Simulation https://www.researchgate.net/publication/330845218_Advances_of_Machine_Learning_in_Molecular_Modeling_and_Simulation.
Boyle, D. Data Science vs. the C Suite
Che-Workshop. Framing the Role of Big Data and Modern Data Science in Chemistry
EBSCOhost - printed on 2/14/2022 11:29 AM via . All use subject to https://www.ebsco.com/terms-of-use
Data science: the fourth paradigm of science
Statistics + computer science + domain knowledge = data science
Data science interrogates (scientific) data at scale. Additional success can be achieved when data science paradigms integrate tools with domain-specific knowledge and expertise. [a]
Transform chemical sciences and engineering
Knowledge discovery at scale
Interdisciplinary: statistics, computer science, applied math, AI, and domain tools
The fourth paradigm: knowledge discovery from data (KDD)
The Fourth Paradigm:Data-Intensive Scientific Discovery [a] is a 2009 anthology of essays on the topic of data science-based on data-intensive computing.
Theory
Experiment
Simulation
Data science
Increase in the use of data is bringing a paradigm shift to the nature of science..
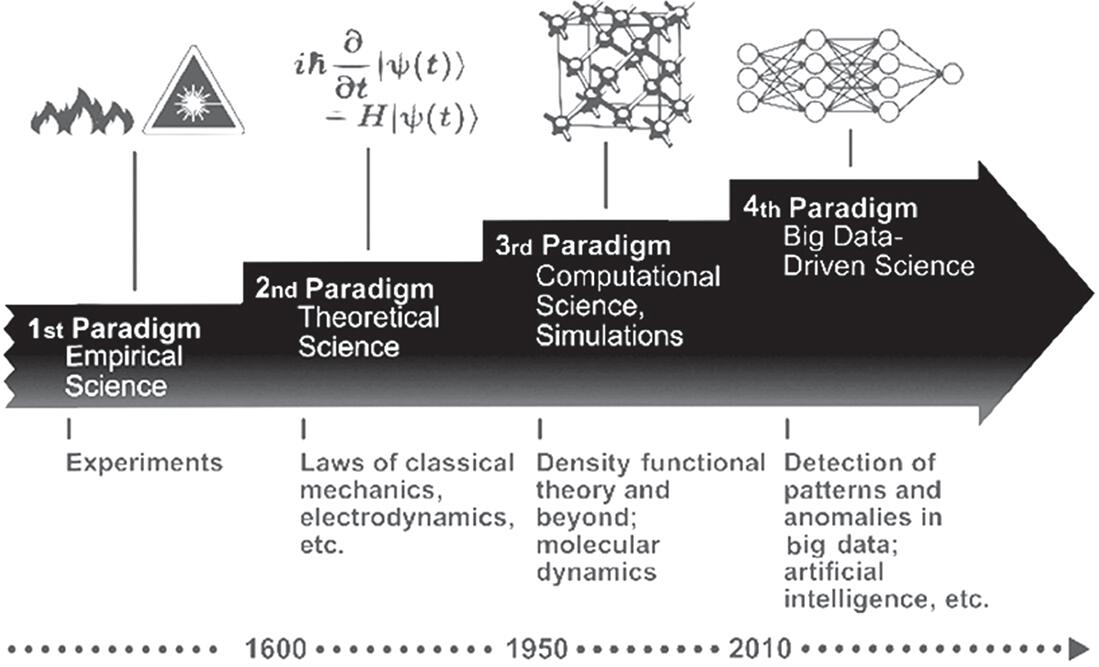
[a] (Draxl, Scheffler).
New technologies and approaches are generating large, diverse data sets. Data science offers methods and tools that are needed to integrate, analyze, and manage these data sets. However, data science applications in the chemical sciences and engineering communities have been relatively limited and many opportunities for advancing the fields have gone unexplored. [a]
Data science life cycle
In general, the life cycle has phases of exploration and production as shown in [a]
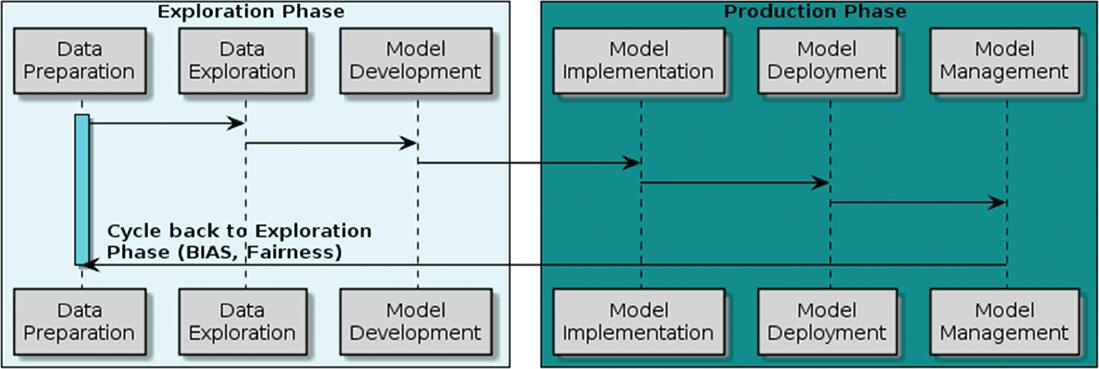
Figure 2.2: Data science life cycle (Gressling).
Challenges
Although data science is a rapidly growing field, some of the building blocks still experience difficulties:
Big data
Data sets are so large that standard approaches and tools for storage, analysis, and sharing fail.
Data may be too large to fit in a 10 TB memory.
Data mining
Machine learning/statistical learning
Artificial intelligence
So, data science brings statistical methods to new scales and prioritizes approximation and uncertainty. It brings new challenges to IT with demand for computing power, memory, and hardware.