Contents
List of Figures
Guide
Pagebreaks of the print version
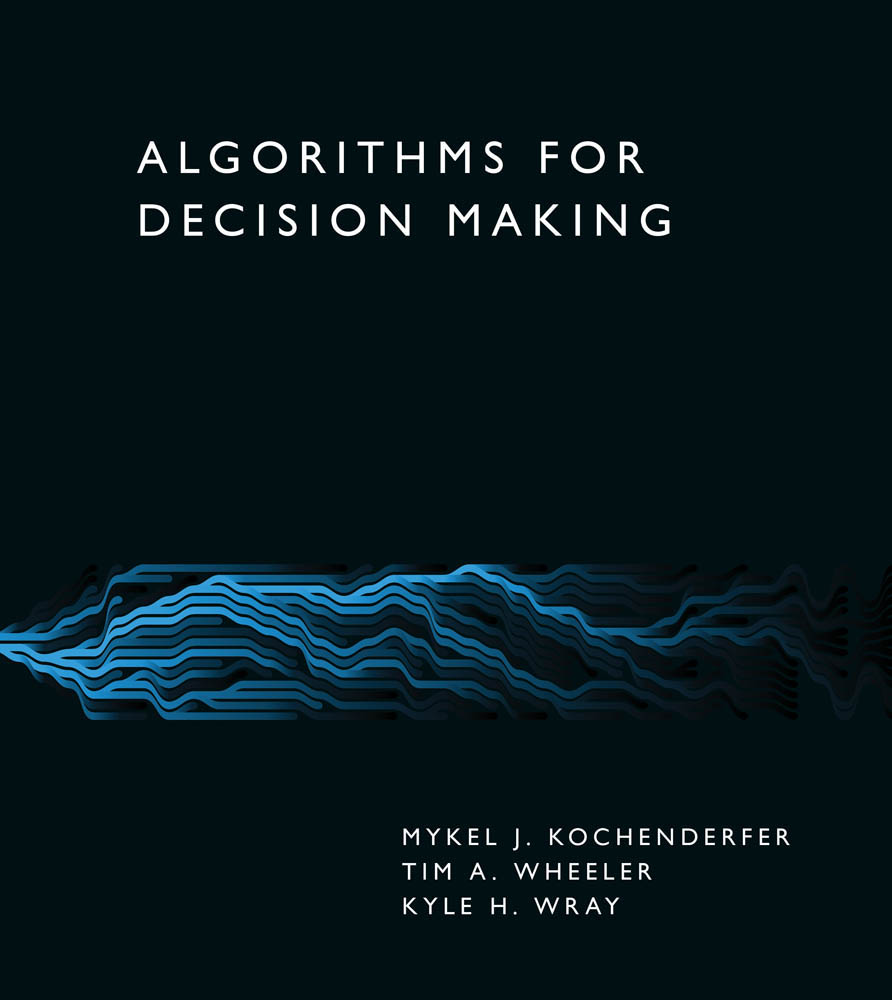
Algorithms for Decision Making
Mykel J. Kochenderfer
Tim A. Wheeler
Kyle H. Wray
The MIT Press
Cambridge, Massachusetts
London, England
2022 Massachusetts Institute of Technology
This work is subject to a Creative Commons CC-BY-NC-ND license. Subject to such license, all rights are reserved.

The MIT Press would like to thank the anonymous peer reviewers who provided comments on drafts of this book. The generous work of academic experts is essential for establishing the authority and quality of our publications. We acknowledge with gratitude the contributions of these otherwise uncredited readers.
This book was set in TEX Gyre Pagella by the authors in LATEX.
Library of Congress Cataloging-in-Publication Data
Names: Kochenderfer, Mykel J., 1980 author. | Wheeler, Tim A. (Tim Allan), author. | Wray, Kyle H., author.
Title: Algorithms for decision making / Mykel J. Kochenderfer, Tim A. Wheeler, Kyle H. Wray.
Description: Cambridge: Massachusetts Institute of Technology, [2022] | Includes bibliographical references and index.
Identifiers: LCCN 2021038701 | ISBN 9780262047012 (hardcover)
Subjects: LCSH: Decision support systemsMathematics. | Algorithms.
Classification: LCC T58.62.K666 2022 | DDC 658.4/03dc23
LC record available at https://lccn.loc.gov/2021038701
d_r0
To our families
Contents
List of Figures
List of Tables
Preface
This book provides a broad introduction to algorithms for decision making under uncertainty. We cover a wide variety of topics related to decision making, introducing the underlying mathematical problem formulations and the algorithms for solving them. Figures, examples, and exercises are provided to convey the intuition behind the various approaches.
This book is intended for advanced undergraduates and graduate students, as well as professionals. It requires some mathematical maturity and assumes prior exposure to multivariable calculus, linear algebra, and probability concepts. Some review material is provided in the appendices. Disciplines where the book would be especially useful include mathematics, statistics, computer science, aerospace, electrical engineering, and operations research.
Fundamental to this textbook are the algorithms, which are all implemented in the Julia programming language. We have found this language to be ideal for specifying algorithms in human-readable form. The priority in the design of the algorithmic implementations was interpretability rather than efficiency. Industrial applications, for example, may benefit from alternative implementations. Permission is granted, free of charge, to use the code snippets associated with this book, subject to the condition that the source of the code is acknowledged.
MYKEL J. KOCHENDERFER
TIM A. WHEELER
KYLE H. WRAY
Stanford, California
February 28, 2022
Acknowledgments
This textbook has grown from a course on decision making under uncertainty taught at Stanford. We are grateful to the students and teaching assistants who have helped shape the course over the past six years.
The authors wish to thank the many individuals who have provided valuable feedback on early drafts of our manuscript, including Dylan Asmar, Drew Bagnell, Safa Bakhshi, Edward Balaban, Jean Betterton, Raunak Bhattacharyya, Kelsey Bing, Maxime Bouton, Austin Chan, Simon Chauvin, Shushman Choudhury, Jon Cox, Matthew Daly, Victoria Dax, Richard Dewey, Dea Dressel, Ben Duprey, Torstein Eliassen, Johannes Fischer, Rushil Goradia, Jayesh Gupta, Arec Jamgochian, Rohan Kapre, Mark Koren, Liam Kruse, Tor Lattimore, Bernard Lange, Ritchie Lee, Sheng Li, Michael Littman, Robert Moss, Joshua Ott, Oriana Peltzer, Francesco Piccoli, Jeffrey Sarnoff, Marc Schlichting, Ransalu Senanayake, Chelsea Sidrane, Chris Strong, Zach Sunberg, Abiy Teshome, Alexandros Tzikas, Kemal Ure, Josh Wolff, Anl Yldz, and Zongzhang Zhang. We also would like to thank Sydney Katz, Kunal Menda, and Ayan Mukhopadhyay for their contributions to the discussion in chapter 1. Ross Alexander produced many of the exercises throughout the book. It has been a pleasure working with Elizabeth Swayze from the MIT Press in preparing this manuscript for publication.
The style of this book was inspired by Edward Tufte. Among other stylistic elements, we adopted his wide margins and use of small multiples. The typesetting of this book is based on the Tufte-LaTeX package by Kevin Godby, Bil Kleb, and Bill Wood. The books color scheme was adapted from the Monokai theme by Jon Skinner of Sublime Text ( For plots, we use the viridis color map defined by Stfan van der Walt and Nathaniel Smith.
We have also benefited from the various open-source packages on which this textbook depends (see appendix G). The typesetting of the code was done with the help of pythontex, which is maintained by Geoffrey Poore. The typeface used for the algorithms is JuliaMono (github.com/cormullion/juliamono). The plotting was handled by pgfplots, which is maintained by Christian Feuersnger.
B. Wong, Points of View: Color Blindness, Nature Methods, vol. 8, no. 6, pp. 441442, 2011.
Introduction
Many important problems involve decision making under uncertainty, including aircraft collision avoidance, wildfire management, and disaster response. When designing automated decision-making systems or decision-support systems, it is important to account for various sources of uncertainty while carefully balancing multiple objectives. We will discuss these challenges from a computational perspective, aiming to provide the theory behind decision-making models and computational approaches. This chapter introduces the problem of decision making under uncertainty, provides some examples of applications, and outlines the space of computational approaches. It then summarizes how various disciplines have contributed to our understanding of intelligent decision making and highlights areas of potential societal impact. We conclude with an outline of the remainder of the book.
1.1Decision Making
An agent is an entity that acts based on observations of its environment. Agents may be physical entities, like humans or robots, or they may be nonphysical entities, such as decision support systems that are implemented entirely in software. As shown in , the interaction between the agent and the environment follows an observe-act cycle or loop.
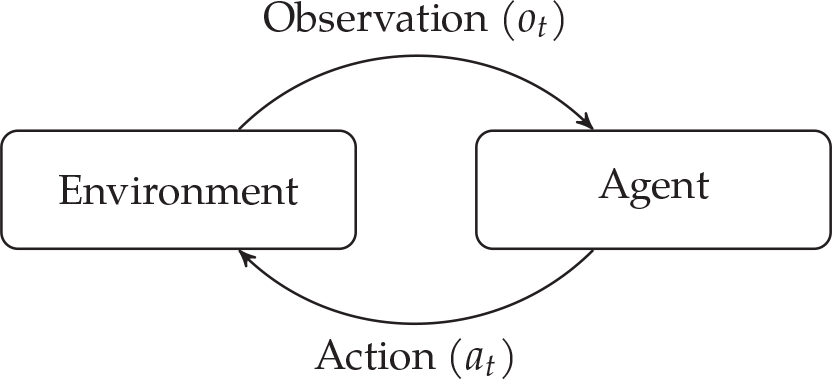
. Interaction between an agent and its environment.
The agent at time t receives an observation of the environment, denoted as ot. Observations may be made, for example, through a biological sensory process, as in humans, or by a sensor system, like radar in an air traffic control system. Observations are often incomplete or noisy; humans may not see an approaching aircraft or a radar system might miss a detection due to electromagnetic interference. The agent then chooses an action at through some decision-making process. This action, such as sounding an alert, may have a nondeterministic effect on the environment.