Hands-On Generative AI with Transformers and Diffusion Models
by Pedro Cuenca , Apolinrio Passos , Omar Sanseviero , and Jonathan Whitaker
Copyright 2024 Pedro Cuenca, Apolinrio Passos, Omar Sanseviero, and Jonathan Whitaker. All rights reserved.
Printed in the United States of America.
Published by OReilly Media, Inc. , 1005 Gravenstein Highway North, Sebastopol, CA 95472.
OReilly books may be purchased for educational, business, or sales promotional use. Online editions are also available for most titles (http://oreilly.com). For more information, contact our corporate/institutional sales department: 800-998-9938 or corporate@oreilly.com.
- Acquisitions Editor: Nicole Butterfield
- Development Editor: Jill Leonard
- Production Editor: Gregory Hyman
- Interior Designer: David Futato
- Cover Designer: Karen Montgomery
- Illustrator: Kate Dullea
- September 2024: First Edition
Revision History for the Early Release
- 2023-03-16: First Release
See http://oreilly.com/catalog/errata.csp?isbn=9781098149246 for release details.
The OReilly logo is a registered trademark of OReilly Media, Inc. Hands-On Generative AI with Transformers and Diffusion Models, the cover image, and related trade dress are trademarks of OReilly Media, Inc.
The views expressed in this work are those of the authors and do not represent the publishers views. While the publisher and the authors have used good faith efforts to ensure that the information and instructions contained in this work are accurate, the publisher and the authors disclaim all responsibility for errors or omissions, including without limitation responsibility for damages resulting from the use of or reliance on this work. Use of the information and instructions contained in this work is at your own risk. If any code samples or other technology this work contains or describes is subject to open source licenses or the intellectual property rights of others, it is your responsibility to ensure that your use thereof complies with such licenses and/or rights.
978-1-098-14924-6
Chapter 1. Diffusion Models
A Note for Early Release Readers
With Early Release ebooks, you get books in their earliest formthe authors raw and unedited content as they writeso you can take advantage of these technologies long before the official release of these titles.
This will be the third chapter of the final book. Please note that the GitHub repo will be made active later on.
If you have comments about how we might improve the content and/or examples in this book, or if you notice missing material within this chapter, please reach out to the editor at .
In late 2020 a little-known class of models called diffusion modelsbegan causing a stir in the machine-learning world. Researchers figuredout how to use these models to generate synthetic images at higher qualitythan any produced by previous techniques. A flurry of papers followed,proposing improvements and modifications that pushed the quality up evenfurther. By late 2021 there were models like GLIDE that showcased incredibleresults on text-to-image tasks, and a few months later, these models hadentered the mainstream with tools like DALL-E 2 and Stable Diffusion.These models made it easy for anyone to generate images just by typingin a text description of what they wanted to see.
In this chapter, were going to dig into the details of how these modelswork. Well outline the key insights that make them so powerful,generate images with existing models to get a feel for how they work,and then train our own models to deepen this understanding further. The fieldis still rapidly evolving, but the topics covered here should give you asolid foundation to build on. Chapter 5 will explore more advanced techniques through the lens of a model called Stable Diffusion, and chapter 6 will explore applications of these techniques beyond simple image generation.
The Key Insight: Iterative Refinement
So what is it that makes diffusion models so powerful? Previoustechniques, such as VAEs or GANs, generate their final output via asingle forward pass of the model. This means the model must geteverything right on the first try. If it makes a mistake, it cant goback and fix it. Diffusion models, on the other hand, generate theiroutput by iterating over many steps. This iterative refinement allowsthe model to correct mistakes made in previous steps and graduallyimprove the output. To illustrate this, lets look at an example of adiffusion model in action.
We can load a pre-trained model using the Hugging Face diffuserslibrary. The pipeline can be used to create images directly, but thisdoesnt show us what is going on under the hood:
# Load the pipeline
image_pipe
=
DDPMPipeline
.
from_pretrained
(
"google/ddpm-celebahq-256"
)
image_pipe
.
to
(
device
);
# Sample an image
image_pipe
()
.
images
[
0
]
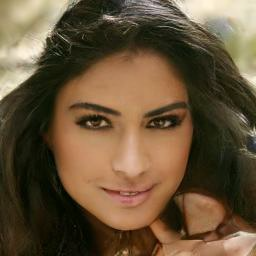
We can re-create the sampling process step by step to get a better lookat what is happening as the model generates images. We initialize oursample x with random noise and then run it through the model for 30steps. On the right, you can see the models prediction for what thefinal image will look like at specific steps - note that the initialpredictions are not particularly good! Instead of jumping right to thatfinal predicted image, we only modify x by a small amount in thedirection of the prediction (shown on the left). We then feed this new,slightly better x through the model again for the next step, hopefullyresulting in a slightly improved prediction, which can be used to updatex a little more, and so on. With enough steps, the model can producesome impressively realistic images.
# The random starting point for a batch of 4 images
x
=
torch
.
randn
(
4
,
3
,
256
,
256
)
.
to
(
device
)
# Set the number of timesteps lower
image_pipe
.
scheduler
.
set_timesteps
(
num_inference_steps
=
30
)
# Loop through the sampling timesteps
for
i
,
t
in
enumerate
(
image_pipe
.
scheduler
.
timesteps
):
# Get the prediction given the current sample x and the timestep t
with
torch
.
no_grad
():
noise_pred
=
image_pipe
.
unet
(
x
,
t
)[
"sample"
]
# Calculate what the updated sample should look like with the scheduler
scheduler_output
=
image_pipe