Peng Liu
Singapore, Singapore
ISBN 978-1-4842-9062-0 e-ISBN 978-1-4842-9063-7
https://doi.org/10.1007/978-1-4842-9063-7
Peng Liu 2023
Apress Standard
The use of general descriptive names, registered names, trademarks, service marks, etc. in this publication does not imply, even in the absence of a specific statement, that such names are exempt from the relevant protective laws and regulations and therefore free for general use.
The publisher, the authors and the editors are safe to assume that the advice and information in this book are believed to be true and accurate at the date of publication. Neither the publisher nor the authors or the editors give a warranty, expressed or implied, with respect to the material contained herein or for any errors or omissions that may have been made. The publisher remains neutral with regard to jurisdictional claims in published maps and institutional affiliations.
This Apress imprint is published by the registered company APress Media, LLC, part of Springer Nature.
The registered company address is: 1 New York Plaza, New York, NY 10004, U.S.A.
Introduction
Bayesian optimization provides a unified framework that solves the problem of sequential decision-making under uncertainty. It includes two key components: a surrogate model approximating the unknown black-box function with uncertainty estimates and an acquisition function that guides the sequential search. This book reviews both components, covering both theoretical introduction and practical implementation in Python, building on top of popular libraries such as GPyTorch and BoTorch. Besides, the book also provides case studies on using Bayesian optimization to seek a simulated function's global optimum or locate the best hyperparameters (e.g., learning rate) when training deep neural networks. The book assumes readers with a minimal understanding of model development and machine learning and targets the following audiences:
Students in the field of data science, machine learning, or optimization-related fields
Practitioners such as data scientists, both early and middle in their careers, who build machine learning models with good-performing hyperparameters
Hobbyists who are interested in Bayesian optimization as a global optimization technique to seek the optimal solution as fast as possible
All source code used in this book can be downloaded from github.com/apress/Bayesian-optimization .
Any source code or other supplementary material referenced by the author in this book is available to readers on GitHub (https://github.com/Apress). For more detailed information, please visit http://www.apress.com/source-code.
Acknowledgments
This book summarizes my learning journey in Bayesian optimization during my (part-time) Ph.D. study. It started as a personal interest in exploring this area and gradually grew into a book combining theory and practice. For that, I thank my supervisors, Teo Chung Piaw and Chen Ying, for their continued support in my academic career.
Table of Contents
About the Author
Peng Liu
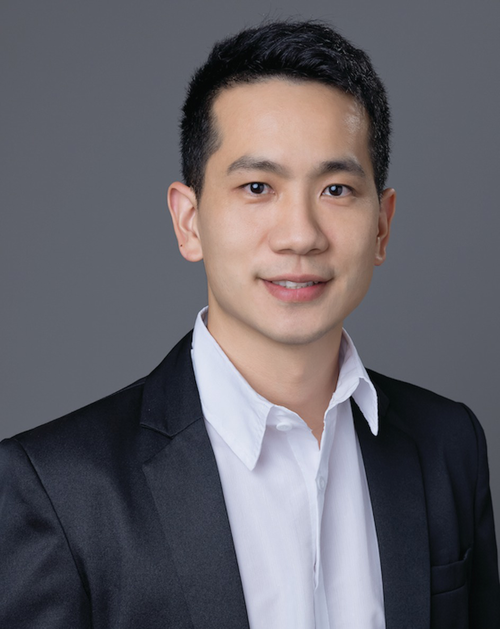
The photograph of Peng Liu.
is an assistant professor of quantitative finance (practice) at Singapore Management University and an adjunct researcher at the National University of Singapore. He holds a Ph.D. in Statistics from the National University of Singapore and has ten years of working experience as a data scientist across the banking, technology, and hospitality industries.
About the Technical Reviewer
Jason Whitehorn
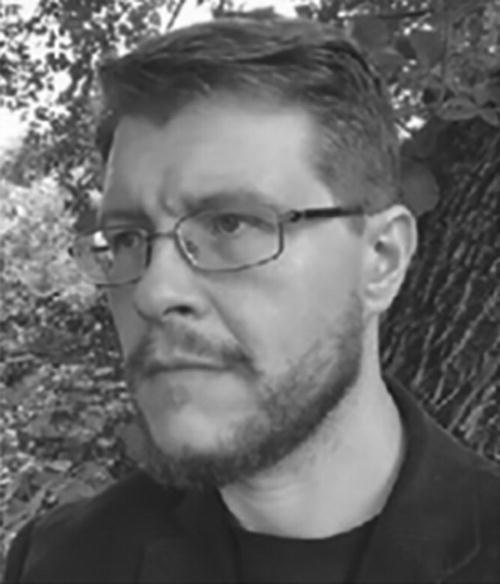
The photograph of Jason Whitehorn.
is an experienced entrepreneur and software developer and has helped many companies automate and enhance their business solutions through data synchronization, SaaS architecture, and machine learning. Jason obtained his Bachelor of Science in Computer Science from Arkansas State University, but he traces his passion for development back many years before then, having first taught himself to program BASIC on his familys computer while in middle school. When hes not mentoring and helping his team at work, writing, or pursuing one of his many side-projects, Jason enjoys spending time with his wife and four children and living in the Tulsa, Oklahoma, region. More information about Jason can be found on his website: https://jason.whitehorn.us .
The Author(s), under exclusive license to APress Media, LLC, part of Springer Nature 2023
P. Liu Bayesian Optimization https://doi.org/10.1007/978-1-4842-9063-7_1
1. Bayesian Optimization Overview
As the name suggests, Bayesian optimization is an area that studies optimization problems using the Bayesian approach. Optimization aims at locating the optimal objective value (i.e., a global maximum or minimum) of all possible values or the corresponding location of the optimum in the environment (the search domain). The search process starts at a specific initial location and follows a particular policy to iteratively guide the following sampling locations, collect new observations, and refresh the guiding policy.
As shown in Figure , the overall optimization process consists of repeated interactions between the policy and the environment. The policy is a mapping function that takes in a new input observation (plus historical ones) and outputs the following sampling location in a principled way. Here, we are constantly learning and improving the policy, since a good policy guides our search toward the global optimum more efficiently and effectively. In contrast, a good policy would save the limited sampling budget on promising candidate locations. On the other hand, the environment contains the unknown objective function to be learned by the policy within a specific boundary. When probing the functional value as requested by the policy, the actual observation revealed by the environment to the policy is often corrupted by noise, making learning even more challenging. Thus, Bayesian optimization, a specific approach for
global optimization , would like to learn a policy that can help us efficiently and effectively navigate to the global optimum of an unknown, noise-corrupted environment as quickly as possible.