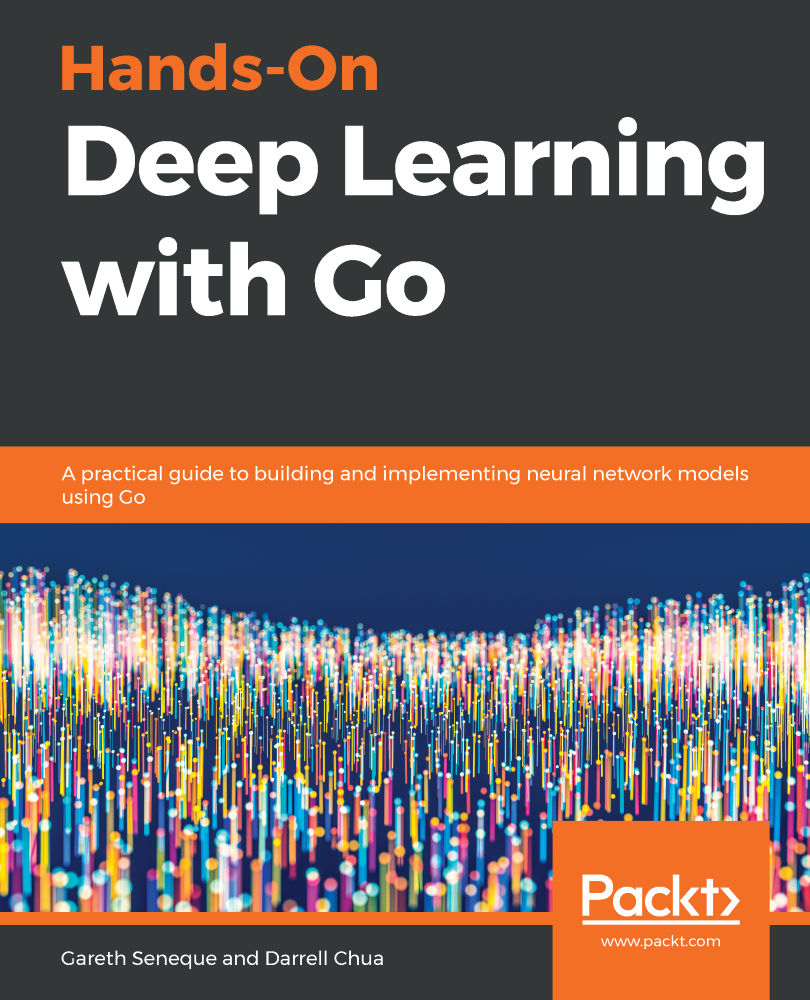
Hands-On Deep Learning
with Go
A practical guide to building and implementing neural network models using Go
Gareth Seneque
Darrell Chua
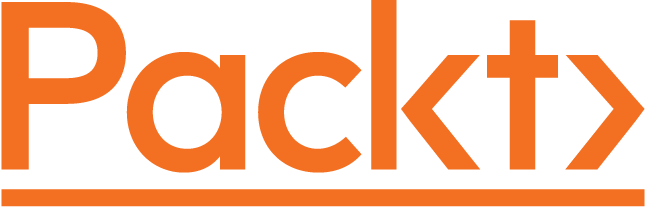
BIRMINGHAM - MUMBAI
Hands-On Deep Learning with Go
Copyright 2019 Packt Publishing
All rights reserved. No part of this book may be reproduced, stored in a retrieval system, or transmitted in any form or by any means, without the prior written permission of the publisher, except in the case of brief quotations embedded in critical articles or reviews.
Every effort has been made in the preparation of this book to ensure the accuracy of the information presented. However, the information contained in this book is sold without warranty, either express or implied. Neither the authors, nor Packt Publishing or its dealers and distributors, will be held liable for any damages caused or alleged to have been caused directly or indirectly by this book.
Packt Publishing has endeavored to provide trademark information about all of the companies and products mentioned in this book by the appropriate use of capitals. However, Packt Publishing cannot guarantee the accuracy of this information.
Commissioning Editor: Pravin Dhandre
Acquisition Editor: Joshua Nadar
Content Development Editor: Roshan Kumar
Senior Editor: Jack Cummings
Technical Editor: Dinesh Chaudhary
Copy Editor: Safis Editing
Project Coordinator: Namrata Swetta
Proofreader: Safis Editing
Indexer: Manju Arasan
Production Designer: Jayalaxmi Raja
First published: August 2019
Production reference: 1060819
Published by Packt Publishing Ltd.
Livery Place
35 Livery Street
Birmingham
B3 2PB, UK.
ISBN 978-1-78934-099-0
www.packtpub.com
Packt.com
Subscribe to our online digital library for full access to over 7,000 books and videos, as well as industry leading tools to help you plan your personal development and advance your career. For more information, please visit our website.
Why subscribe?
Spend less time learning and more time coding with practical eBooks and Videos from over 4,000 industry professionals
Improve your learning with Skill Plans built especially for you
Get a free eBook or video every month
Fully searchable for easy access to vital information
Copy and paste, print, and bookmark content
Did you know that Packt offers eBook versions of every book published, with PDF and ePub files available? You can upgrade to the eBook version at www.packt.com and as a print book customer, you are entitled to a discount on the eBook copy. Get in touch with us at customercare@packtpub.com for more details.
At www.packt.com , you can also read a collection of free technical articles, sign up for a range of free newsletters, and receive exclusive discounts and offers on Packt books and eBooks.
Contributors
About the authors
Gareth Seneque is a machine learning engineer with 11 years' experience of building and deploying systems at scale in the finance and media industries. He became interested in deep learning in 2014 and is currently building a search platform within his organization, using neuro-linguistic programming and other machine learning techniques to generate content metadata and drive recommendations. He has contributed to a number of open source projects, including CoREBench and Gorgonia. He also has extensive experience with modern DevOps practices, using AWS, Docker, and Kubernetes to effectively distribute the processing of machine learning workloads.
Darrell Chua is a senior data scientist with more than 10 years' experience. He has developed models of varying complexity, from building credit scorecards with logistic regression to creating image classification models for trading cards. He has spent the majority of his time working with in fintech companies, trying to bring machine learning technologies into the world of finance . He has been programming in Go for several years and has been working on deep learning models for even longer. Among his achievements is the creation of numerous business intelligence and data science pipelines that enable the delivery of a top-of-the-line automated underwriting system, producing near-instant approval decisions.
About the reviewer
Xuanyi Chew is the primary author of Gorgonia. In his day job, he is the chief data scientist of a rapidly growing local start-up in Sydney . At night, he works on his hobbies of building deep learning AI (using Gorgonia), furthering his hopes of one day building an AGI. He wants to make Go the primary ecosystem for machine learning work and would love your help.
Packt is searching for authors like you
If you're interested in becoming an author for Packt, please visit authors.packtpub.com and apply today. We have worked with thousands of developers and tech professionals, just like you, to help them share their insight with the global tech community. You can make a general application, apply for a specific hot topic that we are recruiting an author for, or submit your own idea.
Preface
Go is an open source programming language designed by Google to handle huge projects efficiently. It makes building reliable, simple, and efficient software straightforward and easy.
This book immediately jumps into the practicalities of implementing Deep Neural Networks (DNNs) in Go. Simply put, the book's title contains its aim. This means there will be a lot of technical detail, a lot of code, and (not too much) math. By the time you finally close the book or turn off your Kindle, you'll know how (and why) to implement modern, scalable DNNs, and be able to repurpose them for your needs in whatever industry or mad science project you're involved.
Who this book is for
This book is for data scientists, machine learning engineers, and deep learning aspirants who are looking to inject deep learning into their Go applications. Familiarity with machine learning and basic Golang code is expected in order to get the most out of this book.
What this book covers
, Introduction to Deep Learning in Go , introduces the history and applications of deep learning. This chapter also gives an overview of ML with Go.
, What is a Neural Network and How Do I Train One? , covers how to build a simple neural network and how to inspect a graph, as well as many of the commonly used activation functions. This chapter also discusses some of the different options for gradient descent algorithms and optimizations for your neural network.
, Beyond Basic Neural Networks Autoencoders and RBMs , shows how to build a simple multilayer neural network and an autoencoder. This chapter also explores the design and implementation of a probabilistic graphical model, an RBM, used in an unsupervised manner to create a recommendation engine for films.
Next page