Mark Treveil - Introducing MLOps
Here you can read online Mark Treveil - Introducing MLOps full text of the book (entire story) in english for free. Download pdf and epub, get meaning, cover and reviews about this ebook. year: 2020, publisher: OReilly Media, Inc., genre: Politics. Description of the work, (preface) as well as reviews are available. Best literature library LitArk.com created for fans of good reading and offers a wide selection of genres:
Romance novel
Science fiction
Adventure
Detective
Science
History
Home and family
Prose
Art
Politics
Computer
Non-fiction
Religion
Business
Children
Humor
Choose a favorite category and find really read worthwhile books. Enjoy immersion in the world of imagination, feel the emotions of the characters or learn something new for yourself, make an fascinating discovery.
- Book:Introducing MLOps
- Author:
- Publisher:OReilly Media, Inc.
- Genre:
- Year:2020
- Rating:3 / 5
- Favourites:Add to favourites
- Your mark:
- 60
- 1
- 2
- 3
- 4
- 5
Introducing MLOps: summary, description and annotation
We offer to read an annotation, description, summary or preface (depends on what the author of the book "Introducing MLOps" wrote himself). If you haven't found the necessary information about the book — write in the comments, we will try to find it.
Introducing MLOps — read online for free the complete book (whole text) full work
Below is the text of the book, divided by pages. System saving the place of the last page read, allows you to conveniently read the book "Introducing MLOps" online for free, without having to search again every time where you left off. Put a bookmark, and you can go to the page where you finished reading at any time.
Font size:
Interval:
Bookmark:
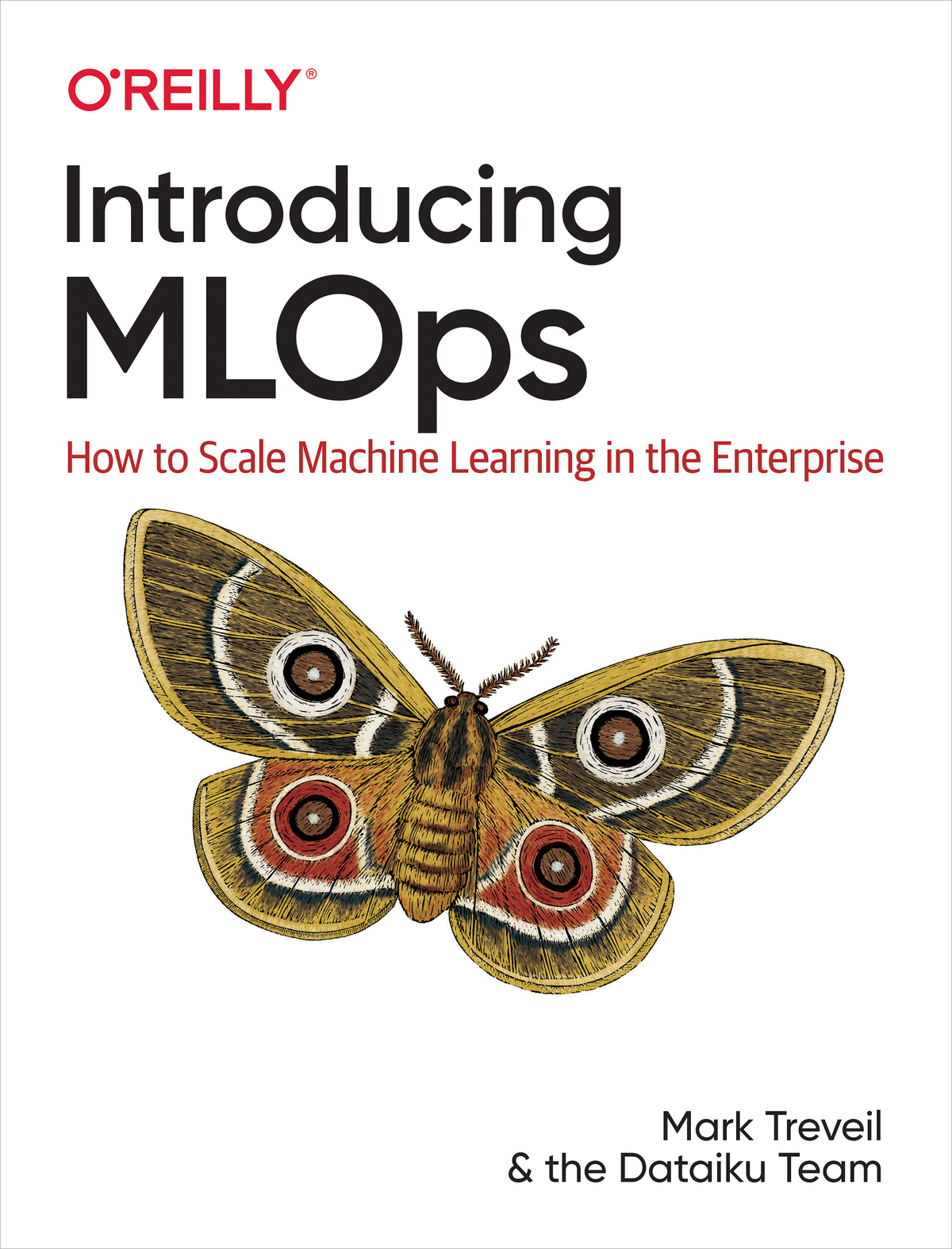
by Mark Treveil , and the Dataiku Team
Copyright 2020 Dataiku. All rights reserved.
Printed in the United States of America.
Published by OReilly Media, Inc. , 1005 Gravenstein Highway North, Sebastopol, CA 95472.
OReilly books may be purchased for educational, business, or sales promotional use. Online editions are also available for most titles (http://oreilly.com). For more information, contact our corporate/institutional sales department: 800-998-9938 or corporate@oreilly.com .
- Acquisitions Editor: Rebecca Novack
- Development Editor: Angela Rufino
- Production Editor: Katherine Tozer
- Copyeditor: Penelope Perkins
- Proofreader: Kim Wimpsett
- Indexer: Ellen Troutman-Zaig
- Interior Designer: David Futato
- Cover Designer: Karen Montgomery
- Illustrator: Kate Dullea
- December 2020: First Edition
- 2020-11-30: First Release
See http://oreilly.com/catalog/errata.csp?isbn=9781492083290 for release details.
The OReilly logo is a registered trademark of OReilly Media, Inc. Introducing MLOps, the cover image, and related trade dress are trademarks of OReilly Media, Inc.
The views expressed in this work are those of the authors, and do not represent the publishers views. While the publisher and the authors have used good faith efforts to ensure that the information and instructions contained in this work are accurate, the publisher and the authors disclaim all responsibility for errors or omissions, including without limitation responsibility for damages resulting from the use of or reliance on this work. Use of the information and instructions contained in this work is at your own risk. If any code samples or other technology this work contains or describes is subject to open source licenses or the intellectual property rights of others, it is your responsibility to ensure that your use thereof complies with such licenses and/or rights.
This work is part of a collaboration between OReilly and Dataiku. See our statement of editorial independence .
978-1-492-08329-0
[LSI]
Weve reached a turning point in the story of machine learning where the technology has moved from the realm of theory and academics and into the real worldthat is, businesses providing all kinds of services and products to people across the globe. While this shift is exciting, its also challenging, as it combines the complexities of machine learning models with the complexities of the modern organization.
One difficulty, as organizations move from experimenting with machine learning to scaling it in production environments, is maintenance. How can companies go from managing just one model to managing tens, hundreds, or even thousands? This is not only where MLOps comes into play, but its also where the aforementioned complexities, both on the technical and business sides, appear. This book will introduce readers to the challenges at hand, while also offering practical insights and solutions for developing MLOps capabilities.
We wrote this book specifically for analytics and IT operations team managers, that is, the people directly facing the task of scaling machine learning (ML) in production. Given that MLOps is a new field, we developed this book as a guide for creating a successful MLOps environment, from the organizational to the technical challenges involved.
This book is divided into three parts. The first is an introduction to the topic of MLOps, diving into how (and why) it has developed as a discipline, who needs to be involved to execute MLOps successfully, and what components are required.
The second part roughly follows the machine learning model life cycle, with chapters on developing models, preparing for production, deploying to production, monitoring, and governance. These chapters cover not only general considerations, but MLOps considerations at each stage of the life cycle, providing more detail on the topics touched on in .
The final part provides tangible examples of how MLOps looks in companies today, so that readers can understand the setup and implications in practice. Though the company names are fictitious, the stories are based on real-life companies experience with MLOps and model management at scale.
The following typographical conventions are used in this book:
ItalicIndicates new terms, URLs, email addresses, filenames, and file extensions.
Constant width
Used for program listings, as well as within paragraphs to refer to program elements such as variable or function names, databases, data types, environment variables, statements, and keywords.
Constant width bold
Shows commands or other text that should be typed literally by the user.
Constant width italic
Shows text that should be replaced with user-supplied values or by values determined by context.
For more than 40 years, OReilly Media has provided technology and business training, knowledge, and insight to help companies succeed.
Our unique network of experts and innovators share their knowledge and expertise through books, articles, and our online learning platform. OReillys online learning platform gives you on-demand access to live training courses, in-depth learning paths, interactive coding environments, and a vast collection of text and video from OReilly and 200+ other publishers. For more information, visit http://oreilly.com.
Please address comments and questions concerning this book to the publisher:
- OReilly Media, Inc.
- 1005 Gravenstein Highway North
- Sebastopol, CA 95472
- 800-998-9938 (in the United States or Canada)
- 707-829-0515 (international or local)
- 707-829-0104 (fax)
We have a web page for this book, where we list errata, examples, and any additional information. You can access this page at https://oreil.ly/intro-mlops.
Email to comment or ask technical questions about this book.
For news and information about our books and courses, visit http://oreilly.com.
Find us on Facebook: http://facebook.com/oreilly
Follow us on Twitter: http://twitter.com/oreillymedia
Watch us on YouTube: http://www.youtube.com/oreillymedia
We would like to thank the entire Dataiku team for their support in developing this book, from conception to completion. Its been a true team effort and, like most things we do at Dataiku, rooted in fundamental collaboration between countless people and teams.
Thanks to those who supported our vision from the beginning of writing this book with OReilly. Thanks to those who stepped in to help with writing and editing. Thanks to those who provided honest feedback (even when it meant more writing and rewriting and re-rewriting). Thanks to those who were internal cheerleaders and, of course, those who helped us promote the finished product to the world.
Machine learning operations (MLOps) is quickly becoming a critical component of successful data science project deployment in the enterprise (). Its a process that helps organizations and business leaders generate long-term value and reduce risk associated with data science, machine learning, and AI initiatives. Yet its a relatively new concept; so why has it seemingly skyrocketed into the data science lexicon overnight? This introductory chapter delves into what MLOps is at a high level, its challenges, why it has become essential to a successful data science strategy in the enterprise, and, critically, why it is coming to the forefront now.
Font size:
Interval:
Bookmark:
Similar books «Introducing MLOps»
Look at similar books to Introducing MLOps. We have selected literature similar in name and meaning in the hope of providing readers with more options to find new, interesting, not yet read works.
Discussion, reviews of the book Introducing MLOps and just readers' own opinions. Leave your comments, write what you think about the work, its meaning or the main characters. Specify what exactly you liked and what you didn't like, and why you think so.