Leslie F. Sikos - Provenance in Data Science: From Data Models to Context-Aware Knowledge Graphs
Here you can read online Leslie F. Sikos - Provenance in Data Science: From Data Models to Context-Aware Knowledge Graphs full text of the book (entire story) in english for free. Download pdf and epub, get meaning, cover and reviews about this ebook. year: 2021, publisher: Springer Nature, genre: Politics. Description of the work, (preface) as well as reviews are available. Best literature library LitArk.com created for fans of good reading and offers a wide selection of genres:
Romance novel
Science fiction
Adventure
Detective
Science
History
Home and family
Prose
Art
Politics
Computer
Non-fiction
Religion
Business
Children
Humor
Choose a favorite category and find really read worthwhile books. Enjoy immersion in the world of imagination, feel the emotions of the characters or learn something new for yourself, make an fascinating discovery.
- Book:Provenance in Data Science: From Data Models to Context-Aware Knowledge Graphs
- Author:
- Publisher:Springer Nature
- Genre:
- Year:2021
- Rating:4 / 5
- Favourites:Add to favourites
- Your mark:
- 80
- 1
- 2
- 3
- 4
- 5
Provenance in Data Science: From Data Models to Context-Aware Knowledge Graphs: summary, description and annotation
We offer to read an annotation, description, summary or preface (depends on what the author of the book "Provenance in Data Science: From Data Models to Context-Aware Knowledge Graphs" wrote himself). If you haven't found the necessary information about the book — write in the comments, we will try to find it.
Leslie F. Sikos: author's other books
Who wrote Provenance in Data Science: From Data Models to Context-Aware Knowledge Graphs? Find out the surname, the name of the author of the book and a list of all author's works by series.
Provenance in Data Science: From Data Models to Context-Aware Knowledge Graphs — read online for free the complete book (whole text) full work
Below is the text of the book, divided by pages. System saving the place of the last page read, allows you to conveniently read the book "Provenance in Data Science: From Data Models to Context-Aware Knowledge Graphs" online for free, without having to search again every time where you left off. Put a bookmark, and you can go to the page where you finished reading at any time.
Font size:
Interval:
Bookmark:
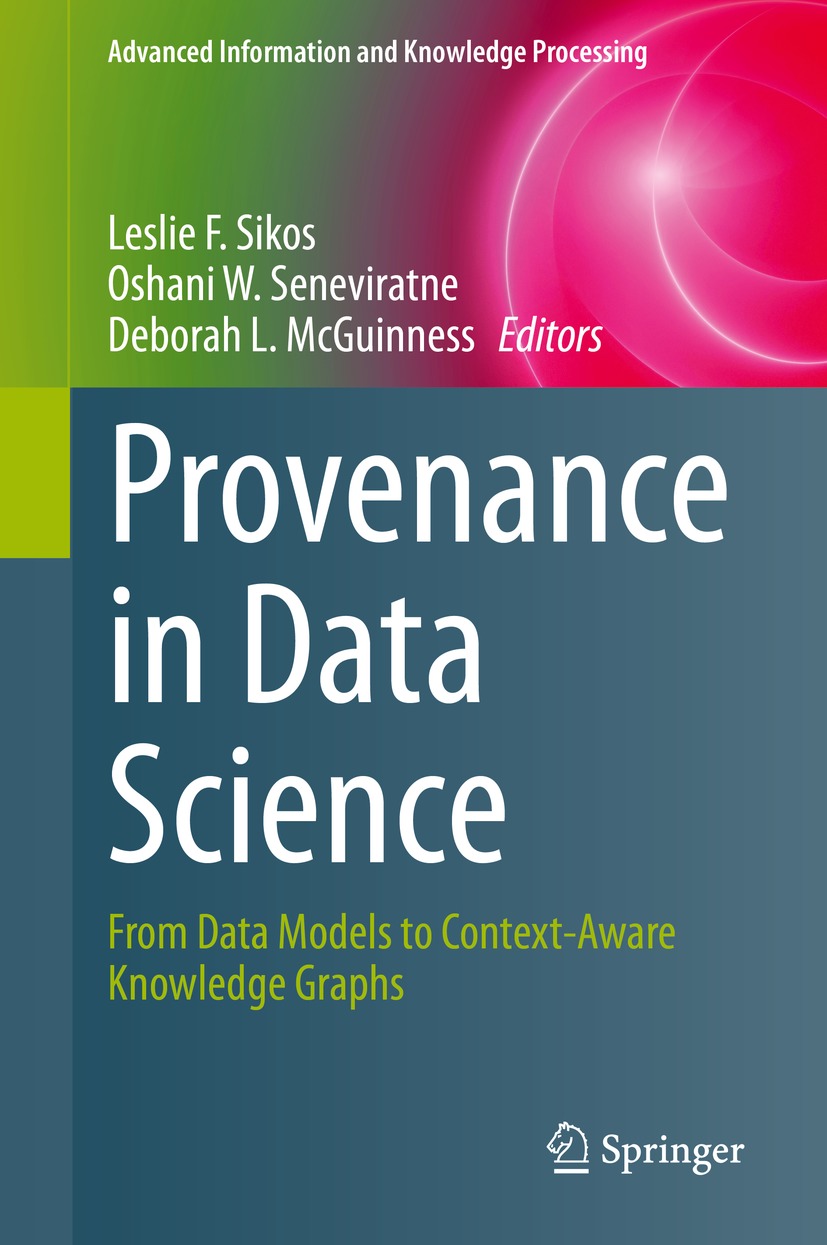
Information systems and intelligent knowledge processing are playing an increasing role in business, science and technology. Recently, advanced information systems have evolved to facilitate the co-evolution of human and information networks within communities. These advanced information systems use various paradigms including artificial intelligence, knowledge management, and neural science as well as conventional information processing paradigms.
The aim of this series is to publish books on new designs and applications of advanced information and knowledge processing paradigms in areas including but not limited to aviation, business, security, education, engineering, health, management, and science.
Books in the series should have a strong focus on information processing - preferably combined with, or extended by, new results from adjacent sciences. Proposals for research monographs, reference books, coherently integrated multi-author edited books, and handbooks will be considered for the series and each proposal will be reviewed by the Series Editors, with additional reviews from the editorial board and independent reviewers where appropriate. Titles published within the Advanced Information and Knowledge Processing Series are included in Thomson Reuters Book Citation Index and Scopus.
More information about this series at http://www.springer.com/series/4738
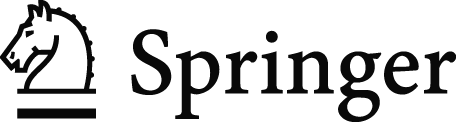
This Springer imprint is published by the registered company Springer Nature Switzerland AG
The registered company address is: Gewerbestrasse 11, 6330 Cham, Switzerland
Since their popularization by Google in 2012, knowledge graphs became the new powerhouse for representing interconnected information of various knowledge domains. What is common in these graphs is that they all use a graph-based data model. The encoding language, style, and infrastructures may vary. Ultimately, knowledge graphs contain information about things and their interrelationships, and the content is represented with something like the Resource Description Framework (RDF). Knowledge graphs broadly cover graphical encodings of semantics and thus include the space of conceptual graphs, labeled property graphs, and hypergraphs. They are often stored in graph databases or triplestores. Knowledge graphs may be seen as marking a new era in data mining, integration, and visualization. They are also powering a range of applications that require distributed processing, complex querying, and automated reasoning. This is witnessed by the growing number of software implementations and feature supportsee the now available RDF knowledge graph support in the latest releases of the industry-leading Oracle Database, which was originally designed as a purely relational database, for example; the Oracle Spatial and Graph support for Graph Visualization using Cytoscape; or the Amazon Neptune fully managed, scalable graph database, which supports both the RDF data model and the property graph model of Apache TinkerPop.
The structure of data determines the tasks that software agents can perform on the data. Knowledge graphs are gaining traction in areas including natural language processing and machine learning implementations as those applications can leverage the structured or semi-structured content captured in the knowledge graphs. Graph-based knowledge representations are highly accessible, scalable, and easy to visualize. They support advanced, semantic search, automated recommendation, and various data querying options corresponding to graph traversal algorithms. While there are many research challenges, knowledge graphs already play an increasingly important role in the development of a range of hybrid AI systems that significantly outperform traditional data processing systems, such as via enhanced deep learning based on knowledge-infused learning processes.
The most significant knowledge graph to date, the World Wide Web, has seen its fair share of challenges and opportunities in its three-plus decades of existence. Users have linked to other documents that resulted in one of the first forms of rudimentary knowledge graphs. Wikipedia started crowd-sourcing knowledge on the Web to create the largest encyclopedia ever to be compiled with many links and connections, resulting in a versatile knowledge graph. The research and development work on Semantic Web has given rise to more structured data formats and even richer data sources on the Web, such as DBPedia and WikiData. Online social networks connected individuals worldwide, giving rise to yet another very large-scale knowledge graph. With the increasing volume of information, questions about where things are coming from became one of the fundamental driving forces behind RDF-based provenance expression, ultimately resulting in a W3C recommendation for provenance.
Font size:
Interval:
Bookmark:
Similar books «Provenance in Data Science: From Data Models to Context-Aware Knowledge Graphs»
Look at similar books to Provenance in Data Science: From Data Models to Context-Aware Knowledge Graphs. We have selected literature similar in name and meaning in the hope of providing readers with more options to find new, interesting, not yet read works.
Discussion, reviews of the book Provenance in Data Science: From Data Models to Context-Aware Knowledge Graphs and just readers' own opinions. Leave your comments, write what you think about the work, its meaning or the main characters. Specify what exactly you liked and what you didn't like, and why you think so.