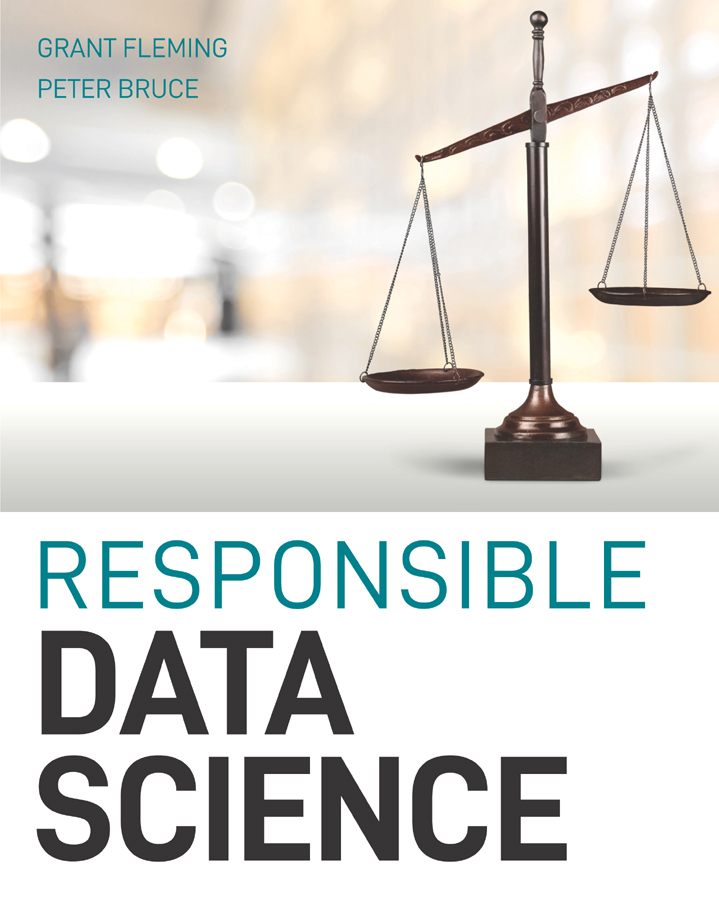
Table of Contents
List of Tables
- Chapter 2
- Chapter 7
- Chapter 8
List of Illustrations
- Chapter 1
- Chapter 2
- Chapter 3
- Chapter 4
- Chapter 5
- Chapter 6
- Chapter 7
- Chapter 8
Guide
Pages
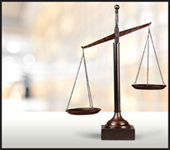
Responsible Data Science
Transparency and Fairness in Algorithms
Grant Fleming
Peter Bruce
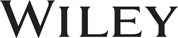
Copyright 2021 by John Wiley & Sons, Inc., Indianapolis, Indiana
Published simultaneously in Canada
ISBN: 978-1-119-74175-6
ISBN: 978-1-119-74177-0 (ebk)
ISBN: 978-1-119-74164-0 (ebk)
No part of this publication may be reproduced, stored in a retrieval system or transmitted in any form or by any means, electronic, mechanical, photocopying, recording, scanning or otherwise, except as permitted under Sections 107 or 108 of the 1976 United States Copyright Act, without either the prior written permission of the Publisher, or authorization through payment of the appropriate per-copy fee to the Copyright Clearance Center, 222 Rosewood Drive, Danvers, MA 01923, (978) 750-8400, fax (978) 646-8600. Requests to the Publisher for permission should be addressed to the Permissions Department, John Wiley & Sons, Inc., 111 River Street, Hoboken, NJ 07030, (201) 748-6011, fax (201) 748-6008, or online at www.wiley.com/go/permissions
.
Limit of Liability/Disclaimer of Warranty: The publisher and the author make no representations or warranties with respect to the accuracy or completeness of the contents of this work and specifically disclaim all warranties, including without limitation warranties of fitness for a particular purpose. No warranty may be created or extended by sales or promotional materials. The advice and strategies contained herein may not be suitable for every situation. This work is sold with the understanding that the publisher is not engaged in rendering legal, accounting, or other professional services. If professional assistance is required, the services of a competent professional person should be sought. Neither the publisher nor the author shall be liable for damages arising herefrom. The fact that an organization or Web site is referred to in this work as a citation and/or a potential source of further information does not mean that the author or the publisher endorses the information the organization or website may provide or recommendations it may make. Further, readers should be aware that Internet websites listed in this work may have changed or disappeared between when this work was written and when it is read.
For general information on our other products and services please contact our Customer Care Department within the United States at (877) 762-2974, outside the United States at (317) 572-3993 or fax (317) 572-4002.
Wiley publishes in a variety of print and electronic formats and by print-on-demand. Some material included with standard print versions of this book may not be included in e-books or in print-on-demand. If this book refers to media such as a CD or DVD that is not included in the version you purchased, you may download this material at booksupport.wiley.com
. For more information about Wiley products, visit www.wiley.com
.
Library of Congress Control Number: 2021933659
Trademarks: Wiley and the Wiley logo are trademarks or registered trademarks of John Wiley & Sons, Inc. and/or its affiliates, in the United States and other countries, and may not be used without written permission. All other trademarks are the property of their respective owners. John Wiley & Sons, Inc. is not associated with any product or vendor mentioned in this book.
About the Authors
GRANT FLEMING is a data scientist at Elder Research, Inc. His professional focus is on machine learning for social science applications, model interpretability, civic technology, and building software tools for reproducible data science.
PETER BRUCE is the Chief Learning Officer at Elder Research, Inc., author of several best-selling texts on data science, and Founder of the Institute for Statistics Education at Statistics.com
, an Elder Research Company.
About the Technical Editor
ROBERT DE GRAAF is a data scientist and statistician from Melbourne, Australia. He is the author of Managing Your Data Science Projects and coauthor of SQL Cookbook, 2nd edition. He is husband to Clare and father to Maya and Leda, and enjoys playing guitar and learning new languages.
Acknowledgments
First and foremost, we acknowledge the support of Elder Research, Inc., and of John Elder (chairman) and Gerhard Pilcher (CEO) in particular. We have benefited greatly from the technical and philosophical conversations we have shared with our colleagues. Elder Research has been most generous in permitting us to pursue this project. At the same time, this book has not been reviewed or edited by the company, and we, the authors, bear sole responsibility for all opinions, errors, and omissions.
We thank, especially, our coauthors on select chapters. Will Goodrum lent his expertise to the legal issues explored in , Auditing for Neural Networks.
Robert de Graaf served as technical editor, raising important points and contributing in many places to a better book. This book certainly would have been incomplete without his input.
Our editorial team at Wiley has been most supportive throughout the process. Jim Minatel, associate publisher, embraced our vision from the beginning. Our editor, Jan Lynn, kept us on track and patiently shepherded the various pieces of the project to all come together. Saravanan Dakshinamurthy handled the production side of things, Louise Watson did the copy-editing, and Pete Gaughan managed the process behind the scenes.
We would like to thank Matthew Dwinnell and Amy Zhang for their tips on working with HTML and CSS, as well as our co-workers Brittany Pugh and Chris Lee for their advice and feedback. Grant would like to thank his professors and mentors, including Edward Munn Sanchez, Lite Nartey, Edward R. Carr, Gregory Magai Patterson, Jennifer Bess, Mark Schaffer, Patrick Jessee, and Brandie Wagner, for their endless support of his efforts and spirit. Peter's appreciation goes to Galit Shmueli, his coauthor on other book projects, with whom he has had lively conversations on ethical issues surrounding the practice of data science.
Finally, we express our appreciation to our students at Statistics.com
who have made constructive and useful comments on the material presented here.
Introduction
In this book, we will review some of the harmful ways artificial intelligence has been used and provide a framework to facilitate the responsible practice of data science. While we will touch upon mitigating legal risks, in this book we will focus primarily on the modeling process itself, especially on how factors overlooked by current modeling practices lead to unintended harms once the model is deployed in a real-world context.
Three core themes will be developed through this book:
- Any AI algorithm can have a harmful, dark side: once they are applied in the real world, AI algorithms can cause any number of harms. An algorithm designed to help police catch murderers can later be appropriated by totalitarian states to persecute dissidents; an algorithm that expands the availability of financial credit for the vast majority of people may nonetheless intensify bias against minorities.
Next page