Barr Moses - Data Quality Fundamentals
Here you can read online Barr Moses - Data Quality Fundamentals full text of the book (entire story) in english for free. Download pdf and epub, get meaning, cover and reviews about this ebook. year: 2022, publisher: OReilly Media, Inc., genre: Politics. Description of the work, (preface) as well as reviews are available. Best literature library LitArk.com created for fans of good reading and offers a wide selection of genres:
Romance novel
Science fiction
Adventure
Detective
Science
History
Home and family
Prose
Art
Politics
Computer
Non-fiction
Religion
Business
Children
Humor
Choose a favorite category and find really read worthwhile books. Enjoy immersion in the world of imagination, feel the emotions of the characters or learn something new for yourself, make an fascinating discovery.
- Book:Data Quality Fundamentals
- Author:
- Publisher:OReilly Media, Inc.
- Genre:
- Year:2022
- Rating:4 / 5
- Favourites:Add to favourites
- Your mark:
- 80
- 1
- 2
- 3
- 4
- 5
Data Quality Fundamentals: summary, description and annotation
We offer to read an annotation, description, summary or preface (depends on what the author of the book "Data Quality Fundamentals" wrote himself). If you haven't found the necessary information about the book — write in the comments, we will try to find it.
Data Quality Fundamentals — read online for free the complete book (whole text) full work
Below is the text of the book, divided by pages. System saving the place of the last page read, allows you to conveniently read the book "Data Quality Fundamentals" online for free, without having to search again every time where you left off. Put a bookmark, and you can go to the page where you finished reading at any time.
Font size:
Interval:
Bookmark:
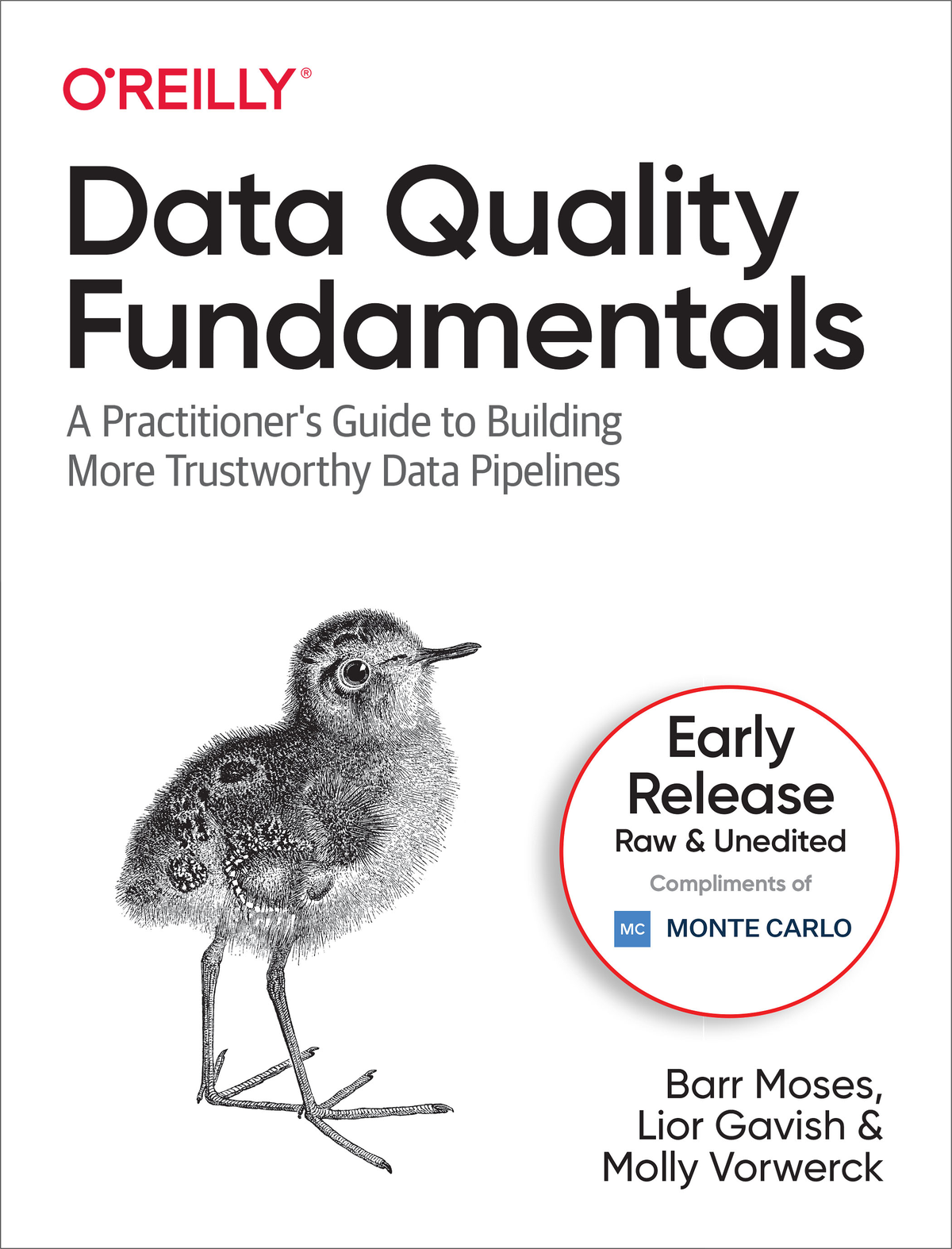
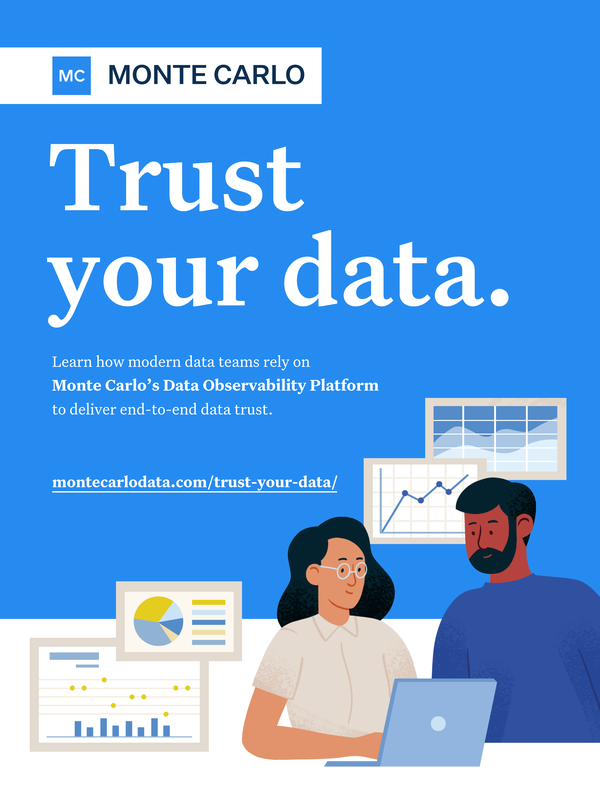
by Barr Moses , Lior Gavish , and Molly Vorwerck
Copyright 2022 Monte Carlo Data, Inc. All rights reserved.
Printed in the United States of America.
Published by OReilly Media, Inc. , 1005 Gravenstein Highway North, Sebastopol, CA 95472.
OReilly books may be purchased for educational, business, or sales promotional use. Online editions are also available for most titles (http://oreilly.com). For more information, contact our corporate/institutional sales department: 800-998-9938 or corporate@oreilly.com .
- Acquisitions Editor: Jessica Haberman
- Development Editor: Jill Leonard
- Production Editor: Gregory Hyman
- Interior Designer: David Futato
- Cover Designer: Karen Montgomery
- Illustrator: Kate Dullea
- September 2022: First Edition
- 2021-10-14: First Release
See http://oreilly.com/catalog/errata.csp?isbn=9781098112042 for release details.
The OReilly logo is a registered trademark of OReilly Media, Inc. Data Quality Fundamentals, the cover image, and related trade dress are trademarks of OReilly Media, Inc.
This work is part of a collaboration between OReilly and Monte Carlo Data. See our statement of editorial independence.
The views expressed in this work are those of the author(s), and do not represent the publishers views. While the publisher and the author(s) have used good faith efforts to ensure that the information and instructions contained in this work are accurate, the publisher and the author(s) disclaim all responsibility for errors or omissions, including without limitation responsibility for damages resulting from the use of or reliance on this work. Use of the information and instructions contained in this work is at your own risk. If any code samples or other technology this work contains or describes is subject to open source licenses or the intellectual property rights of others, it is your responsibility to ensure that your use thereof complies with such licenses and/or rights.
978-1-098-11204-2
With Early Release ebooks, you get books in their earliest formthe authors raw and unedited content as they writeso you can take advantage of these technologies long before the official release of these titles.
This will be the first chapter of the final book. Please note that the GitHub repo will be made active later on.
If you have comments about how we might improve the content and/or examples in this book, or if you notice missing material within this chapter, please reach out to the editor at .
Raise your hand (or spit out your coffee, sigh deeply, and shake your head) if this scenario rings a bell.
Data is a priority for your CEO, as it often is for digital-first companies, and she is fluent in the latest and greatest business intelligence tools. Your CTO is excited about migrating to the cloud, and constantly sends your team articles highlighting performance measurements against some of the latest technologies. Your downstream data consumers including product analysts, marketing leaders, and sales teams rely on data-driven tools like CRMs, CXPs, CMSs, and any other acronym under the sun to do their jobs quickly and effectively.
As the data analyst or engineer responsible for managing this data and making it usable, accessible, and trustworthy, rarely a day goes by without having to field some request from your stakeholders. But what happens when the data is wrong?
Have you ever been about to sign off after a long day running queries or building data pipelines only to get pinged by your Head of Marketing that the data is missing from a critical report? What about a frantic email from your CTO about duplicate data in a business intelligence dashboard? Or a memo from your CEO, the same one who is so bullish on data, about a confusing or inaccurate number in his latest board deck?
If any of these situations hit home for you, youre not alone.
This problem, often referred to as data downtime, happens to even the most innovative and data-first companies, and, in our opinion, its one of the biggest challenges facing businesses in the 21st century. Data downtime refers to periods of time where data is missing, inaccurate, or otherwise erroneous, and it manifests in stale dashboards, inaccurate reports, and even poor decision making.
The root of data downtime? Unreliable data, and lots of it.
Data downtime can cost companies upwards of millions of dollars per year , not to mention customer trust. In fact, ZoomInfo found in 2019 that 1 in 5 companies lost a customer due to a data quality issue.
As youre likely aware, your companys bottomline isnt the only thing thats suffering from data downtime. Handling data quality issues consumes upwards of 40 percent of your teams time that could otherwise be spent working on more interesting projects or actually innovating for the business.
This statistic probably comes as no surprise. It certainly didnt to us.
In a former life, Barr Moses, served as VP of Operations at a customer success software company. Her team was responsible for managing reporting for the broader business, from generating dashboards for her CEO to use during All Hands meetings to setting strategy to reduce customer churn based on user metrics. She was responsible for managing her companys data operations and making sure stakeholders were set up for success when working with data.
Barr will never forget the day she came back to her desk from a grueling, hours-long planning session to find a sticky note with the words The data is wrong on her computer monitor. Not only was this revelation embarrassing, but unfortunately, it wasnt uncommon. Time and again her and her team would encounter these silent and small, but potentially detrimental, issues with their data
There had to be a better way.
Poor data quality and unreliable data have been a problem for organizations for decades, whether its caused by poor reporting, false information, or technical errors. And as organizations increasingly leverage data and build more and more complex data ecosystems and infrastructure, this problem is only slated to increase.
The concept of bad data and poor data quality has been around nearly as long as humans have existed, albeit in different forms. With Captain Robert Falcon Scott and other early Antarctic explorers, poor data quality (or rather, data-uninformed decision making) led them to inaccurately forecast where and how long it would take to get to the South Pole, their target destination.
There are several in more recent memory that stick out, too. Take the infamous Mars Climate Orbiter crash in 1999. A NASA space probe, the Mars Climate Orbiter crashed as a result of a data entry error that produced outputs in non-SI (International System) units versus SI units, bringing it too close to the planet. This crash cost NASA a whopping $125 million dollars. Like spacecraft, analytic pipelines can be extremely vulnerable to the most innocent changes at any stage of the process. Another example comes from the 2008 financial crash, which was spurred in part by inaccurate data that overstated how much mortgage-backed securities and other derivatives.
And this just scratches the surface.
Font size:
Interval:
Bookmark:
Similar books «Data Quality Fundamentals»
Look at similar books to Data Quality Fundamentals. We have selected literature similar in name and meaning in the hope of providing readers with more options to find new, interesting, not yet read works.
Discussion, reviews of the book Data Quality Fundamentals and just readers' own opinions. Leave your comments, write what you think about the work, its meaning or the main characters. Specify what exactly you liked and what you didn't like, and why you think so.