Boris Belousov - Reinforcement Learning Algorithms: Analysis and Applications
Here you can read online Boris Belousov - Reinforcement Learning Algorithms: Analysis and Applications full text of the book (entire story) in english for free. Download pdf and epub, get meaning, cover and reviews about this ebook. year: 2021, publisher: Springer International Publishing, genre: Romance novel. Description of the work, (preface) as well as reviews are available. Best literature library LitArk.com created for fans of good reading and offers a wide selection of genres:
Romance novel
Science fiction
Adventure
Detective
Science
History
Home and family
Prose
Art
Politics
Computer
Non-fiction
Religion
Business
Children
Humor
Choose a favorite category and find really read worthwhile books. Enjoy immersion in the world of imagination, feel the emotions of the characters or learn something new for yourself, make an fascinating discovery.
- Book:Reinforcement Learning Algorithms: Analysis and Applications
- Author:
- Publisher:Springer International Publishing
- Genre:
- Year:2021
- Rating:4 / 5
- Favourites:Add to favourites
- Your mark:
- 80
- 1
- 2
- 3
- 4
- 5
Reinforcement Learning Algorithms: Analysis and Applications: summary, description and annotation
We offer to read an annotation, description, summary or preface (depends on what the author of the book "Reinforcement Learning Algorithms: Analysis and Applications" wrote himself). If you haven't found the necessary information about the book — write in the comments, we will try to find it.
Reinforcement Learning Algorithms: Analysis and Applications — read online for free the complete book (whole text) full work
Below is the text of the book, divided by pages. System saving the place of the last page read, allows you to conveniently read the book "Reinforcement Learning Algorithms: Analysis and Applications" online for free, without having to search again every time where you left off. Put a bookmark, and you can go to the page where you finished reading at any time.
Font size:
Interval:
Bookmark:
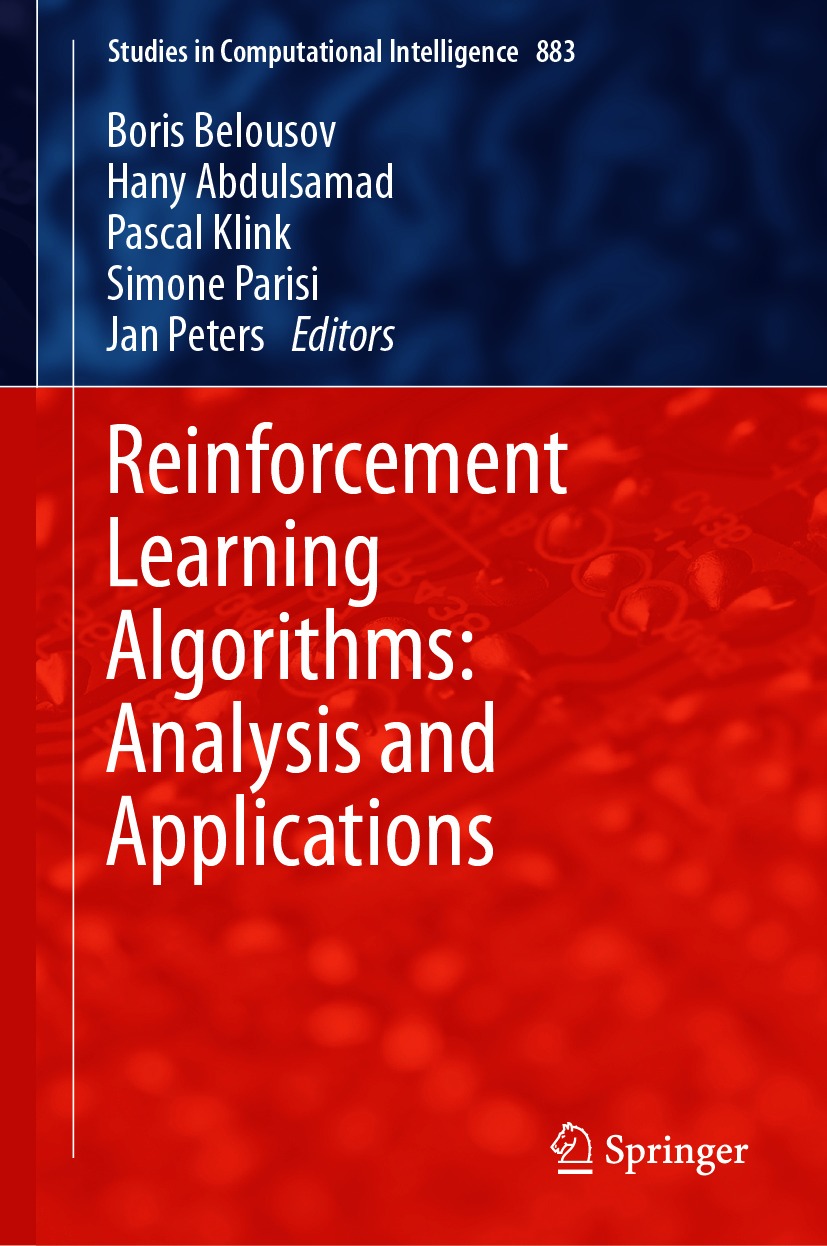
The series "Studies in Computational Intelligence" (SCI) publishes new developments and advances in the various areas of computational intelligencequickly and with a high quality. The intent is to cover the theory, applications, and design methods of computational intelligence, as embedded in the fields of engineering, computer science, physics and life sciences, as well as the methodologies behind them. The series contains monographs, lecture notes and edited volumes in computational intelligence spanning the areas of neural networks, connectionist systems, genetic algorithms, evolutionary computation, artificial intelligence, cellular automata, self-organizing systems, soft computing, fuzzy systems, and hybrid intelligent systems. Of particular value to both the contributors and the readership are the short publication timeframe and the world-wide distribution, which enable both wide and rapid dissemination of research output.
Indexed by SCOPUS, DBLP, WTI Frankfurt eG, zbMATH, SCImago.
All books published in the series are submitted for consideration in Web of Science.
More information about this series at http://www.springer.com/series/7092
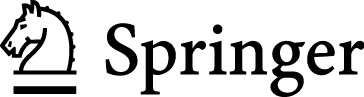
This Springer imprint is published by the registered company Springer Nature Switzerland AG
The registered company address is: Gewerbestrasse 11, 6330 Cham, Switzerland
This book grew out of a lecture course on reinforcement learning held by Prof. Jan Peters in the winter semester 2018/2019 at Technische Universitt Darmstadt. Student research papers covering in depth most prominent research directions in reinforcement learning constitute the core of this volume.
Each chapter of the book provides an overview of a specific topic considered in the lecture, with the parts of the book corresponding to big overarching themes in reinforcement learning. The first part is devoted to the connections with psychology and reward signals in nature. The second part focuses on information-geometric aspects of policy optimization algorithms. The third part covers model-free actor-critic methods, which combine value-based and policy-based algorithms to achieve a better bias-variance trade-off. The fourth part describes model-based approaches, which hold the promise to be more sample-efficient than their model-free counterparts.
The board of editors consists of doctoral students and research assistants at TU Darmstadt headed by Prof. Jan Peters. Each part of the book was reviewed and edited by specialists in the corresponding research area.
The book is intended for machine learning and reinforcement learning students and researchers. Knowledge of linear algebra and statistics is desirable. Nevertheless, all key concepts are introduced in each respective part and chapter of the book, keeping the presentation self-contained and accessible.
We would like to thank our colleagues who helped in organizing the course and assisted in supervising the student research projects: Dr. Riad Akrour, Dr. Joni Pajarinen, Oleg Arenz, Daniel Tanneberg, Svenja Stark, Fabio Muratore, Samuele Tosatto, and Michael Lutter. Last but not least, we thank our families and friends who supported and encouraged us at all stages of this project.
Font size:
Interval:
Bookmark:
Similar books «Reinforcement Learning Algorithms: Analysis and Applications»
Look at similar books to Reinforcement Learning Algorithms: Analysis and Applications. We have selected literature similar in name and meaning in the hope of providing readers with more options to find new, interesting, not yet read works.
Discussion, reviews of the book Reinforcement Learning Algorithms: Analysis and Applications and just readers' own opinions. Leave your comments, write what you think about the work, its meaning or the main characters. Specify what exactly you liked and what you didn't like, and why you think so.