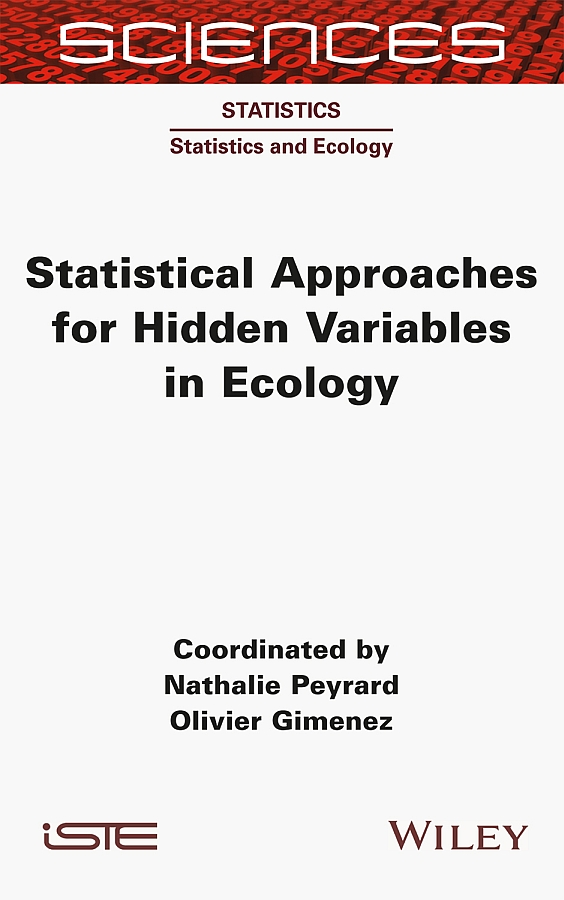
SCIENCES
Statistics, Field Directors Nikolaos Limnios, Kerrie Mengersen
Statistics and Ecology, Subject Head Nathalie Peyrard
Statistical Approaches for Hidden Variables in Ecology
Coordinated by
Nathalie Peyrard
Olivier Gimenez

First published 2022 in Great Britain and the United States by ISTE Ltd and John Wiley & Sons, Inc.
Apart from any fair dealing for the purposes of research or private study, or criticism or review, as permitted under the Copyright, Designs and Patents Act 1988, this publication may only be reproduced, stored or transmitted, in any form or by any means, with the prior permission in writing of the publishers, or in the case of reprographic reproduction in accordance with the terms and licenses issued by the CLA. Enquiries concerning reproduction outside these terms should be sent to the publishers at the undermentioned address:
ISTE Ltd
27-37 St Georges Road
London SW19 4EU
UK
www.iste.co.uk
John Wiley & Sons, Inc.
111 River Street
Hoboken, NJ 07030
USA
www.wiley.com
ISTE Ltd 2022
The rights of Nathalie Peyrard and Olivier Gimenez to be identified as the authors of this work have been asserted by them in accordance with the Copyright, Designs and Patents Act 1988.
Any opinions, findings, and conclusions or recommendations expressed in this material are those of the author(s), contributor(s) or editor(s) and do not necessarily reflect the views of ISTE Group.
Library of Congress Control Number: 2021949076
British Library Cataloguing-in-Publication Data
A CIP record for this book is available from the British Library
ISBN 978-1-78945-047-7
ERC code:
PE1 Mathematics
PE1_14 Statistics
LS8 Ecology, Evolution and Environmental Biology
Introduction
Nathalie PEYRARD
University of Toulouse, INRAE, UR MIAT, Castanet-Tolosan, France
Paris-Saclay University, AgroParisTech, INRAE, UMR MIA-Paris, France
CEFE, University of Montpellier, CNRS, EPHE, IRD, Paul Valry Montpellier 3 University, France
I.1. Hidden variables in ecology
Ecology is the study of living organisms in interaction with their environment. These interactions occur at individual level (an animal, a plant), at the level of groups of individuals (a population, a species) or across several species (a community). Statistics provides us with tools to study these interactions, enabling us to collect, organize, present, analyze and draw conclusions from data collected on ecological systems. However, some components of these ecological systems may escape observation: these are known as hidden variables. This book is devoted to models incorporating hidden variables in ecology and to the statistical inference for these models.
The hidden variables studied throughout this book can be grouped into three classes corresponding to three types of questions that can be posed concerning an ecological system. We may consider the identification of groups of individuals or species, such as groups of individuals with the same behavior or similar genetic profiles, or groups of species that interact with the same species or with their environment in a similar way. Alternatively, we may wish to study variables which can only be observed in a noisy form, often called a proxy. For example, the presence of certain species may be missed as a result of detection difficulties or errors (confusion with another species), or as a result of noisy data resulting from technology-related measurement errors. Finally, in the context of data analysis, we may wish to reduce the dimension of the information contained in data sets to a small number of explanatory variables. Note the shift from the notion of a variable which escapes observation, in the first cases, to a more generalized notion of hidden variables.
All three of these problems can be translated into questions of inference concerning variables which, in statistical terms, are said to be latent. Inference poses statistical problems that require specific methods, described in detail here. The ecological interpretation of these variables will also be discussed at length. As we shall see, while the statistical treatment of these variables may be complex, their inclusion in models is essential in providing us with a better understanding of ecological systems.
I.2. Hidden variables in statistical modeling
The term hidden variable, widely used in ecology, finds its translation in the more general notion of latent variables in statistical modeling. This notion encompasses several situations and goes beyond the idea of unobservable physical variables alone. In statistics, a latent variable is generally defined as a variable of interest, which is not observable and does not necessarily have a physical meaning, the value of which must be deduced from observations. More precisely, latent variables are characterized by the following two specificities: (i) in terms of number, they are comparable to the number of data items, unlike parameters that are fewer in number. Consider, for example, the case of a hidden Markov chain, where the number of observed variables and latent variables is equal to the number of observation time steps; (ii) if their value were known, then model parameter estimation would be easier. For example, consider the estimation of parameters of a mixture model where the groups of individuals are known.
In practice, if a latent variable has a physical reality but cannot be observed in the field (e.g. the precise trajectory of an animal, or the abundance of a seedbank), it is often referred to as a hidden variable (although both terms are often used interchangeably). In other cases, the latent variable naturally plays a role in the description of a given process or system, but has no physical existence. This is the case, for example, of latent variables corresponding to a classification of observations into different groups. We will refer to them as fictitious variables. Finally, latent variables may also play an instrumental role in describing a source of variability in observations that cannot be explained by known covariates, or in establishing a concise description of a dependency structure. They may result from a dimension reduction operation applied to a group of explanatory variables in the context of regression, as we see in the case of the principal components of a principal component analysis.
The notion of latent variables is connected to that of hierarchical models: if they are not parameters, the elements in the higher levels of the model are latent variables. It is important to note that the notion of latent variables may be extended to cover the case of determinist quantities (represented by a constant in a model). For example, this holds true in cases where the latent variable is the trajectory of an ordinary differential equation (ODE) for which only noisy observations are available.
I.3. Statistical methods
Some of the most common examples of statistical models featuring latent variables are described here.
Mixture models are used to define a small number of groups into which a set of observations may be sorted. In this case, the latent variables are discrete variables indicating which group each observation belongs to. Stochastic block models (SBMs) or latent block models (LBMs, or bipartite SBM) are specific forms of mixture models used in cases where the observations take the form of a network. Hidden Markov models (HMMs) are often used to analyze data collected over a period of time (such as the trajectory of an animal, observed over a series of dates) and take account of a subjacent process (such as the activity of the tracked animal: sleep, movement, hunting, etc.), which affects observations (the animals position or trajectory). In this case, the latent variables are discrete and represent the activity of the animal at every instant. In other models, the hidden process itself may be continuous. Mixed (generalized) linear models are one of the key tools used in ecology to describe the effects of a set of conditions (environmental or otherwise) on a population or community. These models include random effects which are, in essence, latent variables, used to account for higher than expected dispersions or dependency relationships between variables. In most cases, these latent variables are continuous and essentially instrumental in nature. Joint species distribution models (JSDMs) are a multidimensional version of generalized linear models, used to describe the composition of a community as a function of both environmental variables and of the interactions between constituent species. Many JSDMs use a multidimensionsal (e.g. Gaussian) latent variable, the dependency structure of which is used to describe inter-species interactions.
Next page