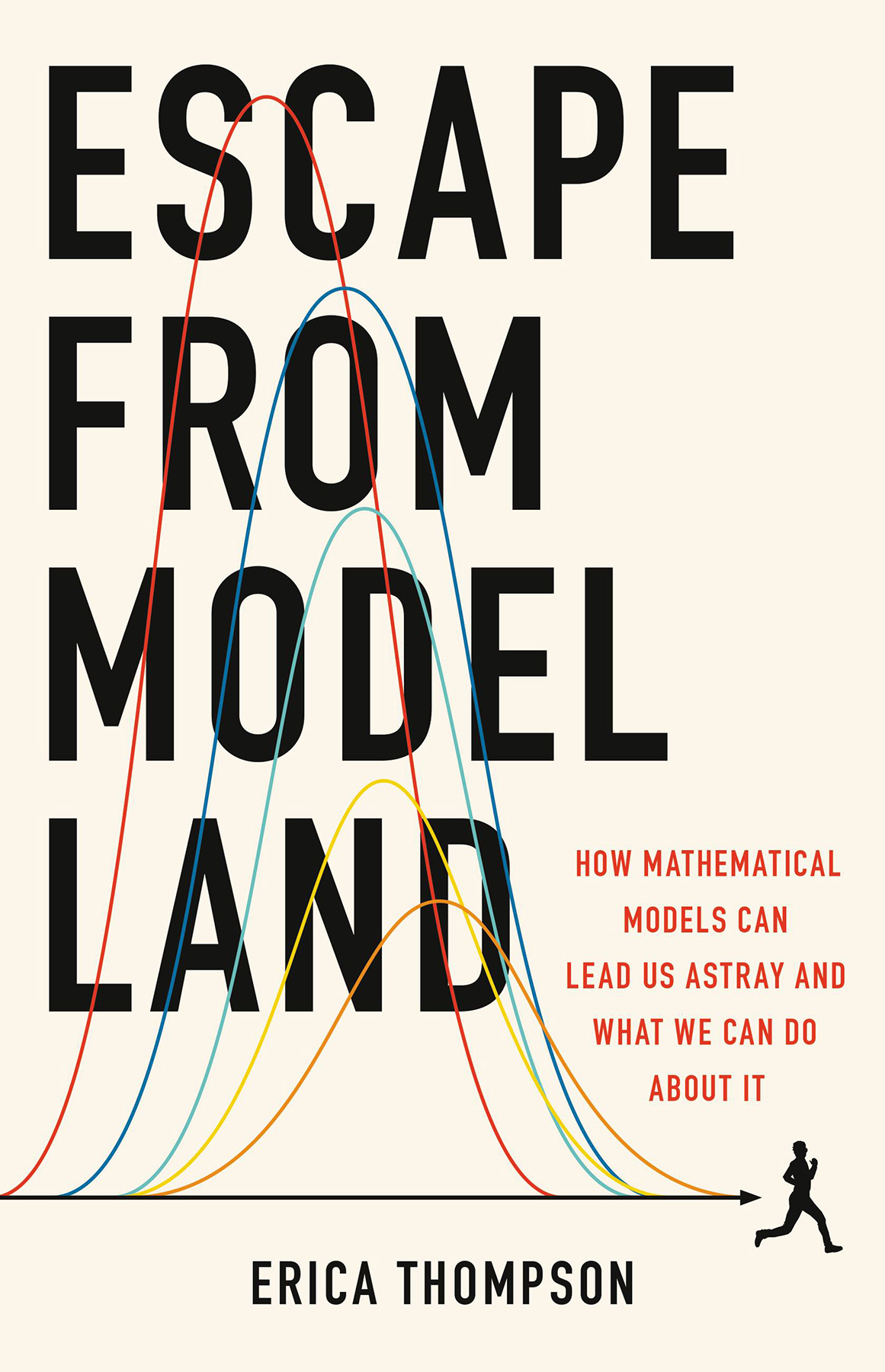
Copyright 2022 by Erica Thompson
Cover design by Kapo Ng
Cover image Sam Chung
Cover copyright 2022 by Hachette Book Group, Inc.
Hachette Book Group supports the right to free expression and the value of copyright. The purpose of copyright is to encourage writers and artists to produce the creative works that enrich our culture.
The scanning, uploading, and distribution of this book without permission is a theft of the authors intellectual property. If you would like permission to use material from the book (other than for review purposes), please contact permissions@hbgusa.com. Thank you for your support of the authors rights.
Basic Books
Hachette Book Group
1290 Avenue of the Americas, New York, NY 10104
www.basicbooks.com
Originally published in Great Britain in 2022 by Basic Books UK, an imprint of John Murray Press, an Hachette UK company
First US Edition: December 2022
Published by Basic Books, an imprint of Perseus Books, LLC, a subsidiary of Hachette Book Group, Inc. The Basic Books name and logo is a trademark of the Hachette Book Group.
The Hachette Speakers Bureau provides a wide range of authors for speaking events. To fi nd out more, go to www.hachettespeakersbureau.com or call ( 866 ) 376-6591.
The publisher is not responsible for websites (or their content) that are not owned by the publisher.
Figure 2 : Photograph of Bill Phillips from LSE Library
Library of Congress Control Number: 2022941135
ISBNs: 9781541600980 (hardcover), 9781541600997 (ebook)
E3-20221015-JV-NF-ORI
For Anne and Alan
I would like to know if anyone has ever seen a natural work of art. Nature and art, being two different things, cannot be the same thing. Through art we express our conception of what nature is not.
Pablo Picasso, Picasso Speaks (1923)
Our world is awash with data. Satellites continuously watch the Earth below, sending back data about rainforest destruction in the Amazon, cyclones in the Indian Ocean and the extent of the polar ice sheets. Tracking algorithms continuously watch human behaviour online, monitoring our time spent on different websites, measuring our propensity to click on different adverts and noting our purchases with an eagle eye. Athletes from Olympians to casual joggers track their daily performance and physical condition. Financial markets create vast quantities of high-frequency price data.
These are all observed directly from the real world, but without a framework to interpret that data it would be only a meaningless stream of numbers. The frameworks we use to interpret data take many different forms, and I am going to refer to all of them as models. Models can be purely conceptual: an informal model of the casual jogger might be when my heart rate goes up I am exercising more intensely. But with data, we can go further and make quantitative models that seek to describe the relationships between one variable and another: 10% of people who clicked on this product listing for a bicycle pump in the last week went on to buy it. The contribution of the model is to add relationships between data. These are observed relationships, and they may have some uncertainty. We do not yet know which 10% of customers would buy the pump. They are also influenced by our own expectations. We did not test how many of the people who clicked on the bicycle pump went on to buy an ironing board or a tin of tomatoes, but we might want to test how many of the people who clicked on this bicycle pump went on to buy a competing brand of pump, or whether those people also bought other sports equipment.
And what is the point of doing that? Well, very few people are interested in these kinds of numbers for their own sake. What the vendor really wants to know is whether the purchase of the bicycle pump can be made more likely, and whether other items could be advertised as related in order to interest the customer in spending more money on the same site. What the funding agencies who send up satellites want to know is how we can understand and predict weather and climate more effectively in order to avoid negative impacts. What the athlete wants to know is how they can improve their performance. What the financial trader wants to know is how they can get an edge.
As the first wave of the Covid-19 pandemic swept across the world in early 2020, governments desperately sought advice from epidemiologists and mathematical modellers about what might happen next and how to respond effectively. Information was scarce, but decisions were urgent. There was uncertainty about the characteristics of the novel virus, uncertainty about how people and communities would respond to major government interventions, and huge uncertainty about the longer-term epidemiological and economic outcomes.
Into that context, mathematical modellers brought quantitative scenarios possible futures that might come to pass depending on certain assumptions. In particular, these models showed that if the proportion of serious cases were in the plausible range and no interventions were made, then a large number of people could be expected to require hospitalisation simultaneously. The very simplest model of infection transmission from one person to more than one other person is a framework with which you can make both large-scale conclusions about exponential growth in a population and small-scale conclusions about reduction of personal risk by reducing contacts. More complex models can represent semi-realistic differences in contact patterns over time for different sectors of society, and account for the effects of new information, better treatments, or vaccination programmes. Even the sad human toll can only be estimated through modelling. Doing so offers the possibility of predicting the effect of different kinds of interventions, obviously of interest to large-scale policy-making. And so in the years since their introduction, epidemiological models have held a place at the top table of national policy-making and on the front pages of the public media.
In short, the purpose of modelling relationships between data is to try to predict how we can take more effective actions in future in support of some overall goal. These are real-world questions informed by real-world data; and when they have answers at all, they have real-world answers. To find those answers, we have to go to Model Land.
Entering Model Land
There are no border controls between the real world and Model Land. You are free to enter any time you like, and you can do so from the comfort of your armchair simply by creating a model. It could be an ethereal, abstract conceptual model, a down-to-earth spreadsheet or a lumbering giant of a complex numerical model. Within Model Land, the assumptions that you make in your model are literally true. The model is the Model Land reality.
Of course, there are many different regions of Model Land, corresponding to the many different models we can make, even of the same situations. In one part of Model Land, the Earth is flat: a model to calculate the trajectory of a cricket ball does not need to include the radius of the Earth. In another part of Model Land, the Earth is a perfect sphere: a globe shows the relative positions of countries and continents with sufficient accuracy for most geographical purposes. To other modellers, the Earth is an oblate spheroid, 43km wider at the equator than through the poles. For the most detailed applications like constructing a GPS system or measuring the gravitational field, we also need to know about mountains and valleys, high plateaus and ocean trenches. All of these are models of the same Earth, but with different applications in mind.
Next page