Table of Contents
List of Tables
- Chapter 2
- Chapter 3
- Chapter 4
List of Illustrations
- Chapter 2
- Chapter 3
- Chapter 5
- Chapter 7
Guide
Pages
Founded in 1807, John Wiley & Sons is the oldest independent publishing company in the United States. With offices in North America, Europe, Australia, and Asia, Wiley is globally committed to developing and marketing print and electronic products and services for our customers professional and personal knowledge and understanding.
The Wiley Trading series features books by traders who have survived the market's ever changing temperament and have prosperedsome by reinventing systems, others by getting back to basics. Whether a novice trader, professional, or somewhere in-between, these books will provide the advice and strategies needed to prosper today and well into the future.
For a list of available titles, visit our Web site at www.WileyFinance.com.
Quantitative Trading
How to Build Your Own Algorithmic Trading Business
Second Edition
ERNEST P. CHAN
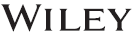
Copyright 2021 by Ernest P. Chan. All rights reserved.
Published by John Wiley & Sons, Inc., Hoboken, New Jersey.
Published simultaneously in Canada.
No part of this publication may be reproduced, stored in a retrieval system, or transmitted in any form or by any means, electronic, mechanical, photocopying, recording, scanning, or otherwise, except as permitted under Section 107 or 108 of the 1976 United States Copyright Act, without either the prior written permission of the Publisher, or authorization through payment of the appropriate per-copy fee to the Copyright Clearance Center, Inc., 222 Rosewood Drive, Danvers, MA 01923, (978) 750-8400, fax (978) 646-8600, or on the web at www.copyright.com. Requests to the Publisher for permission should be addressed to the Permissions Department, John Wiley & Sons, Inc., 111 River Street, Hoboken, NJ 07030, (201) 748-6011, fax (201) 748-6008, or online at http://www.wiley.com/go/permissions.
Limit of Liability/Disclaimer of Warranty: While the publisher and author have used their best efforts in preparing this book, they make no representations or warranties with respect to the accuracy or completeness of the contents of this book and specifically disclaim any implied warranties of merchantability or fitness for a particular purpose. No warranty may be created or extended by sales representatives or written sales materials. The advice and strategies contained herein may not be suitable for your situation. You should consult with a professional where appropriate. Neither the publisher nor author shall be liable for any loss of profit or any other commercial damages, including but not limited to special, incidental, consequential, or other damages.
For general information on our other products and services or for technical support, please contact our Customer Care Department within the United States at (800) 762-2974, outside the United States at (317) 572-3993 or fax (317) 572-4002.
Wiley also publishes its books in a variety of electronic formats. Some content that appears in print may not be available in electronic books. For more information about Wiley products, visit our web site at www.wiley.com.
Library of Congress Cataloging-in-Publication Data is Available:
ISBN 9781119800064 (hardback)
ISBN 9781119800088 (ePDF)
ISBN 9781119800071 (ePub)
Cover Design: Wiley
Cover Image: Jobalou/Getty Images
To my parents, Hung Yip and Ching, and to Ben and Sarah Ming.
Preface to the 2nd Edition
When I first started thinking about writing the 2nd edition, I had a measure of dread. What could I have added that would be new and interesting? After writing the first draft, I was relieved, and incredibly excited, at the prospect of sharing with you my latest knowledge, techniques, and insights, ranging from the addition of some new functions that make our PCA example run more than 10x faster, to a novel application of machine learning.
In the 1st edition of this book, published more than a decade ago, I maintained that independent quantitative traders can beat institutional managers at their own game. Many of you have taken that advice to heart, and many retail quantitative trading communities and platforms have been built to serve just such an ambition. But does the premise still hold?
Over the years, many readers reached out and told me how successful they have been in improving and trading the strategies I discussed in my books, and others told me how they have simply been inspired by my books to become successful traders. Our fund is invested in some of these readers, some of whom have been managing many millions more dollars than we are. So, the answer to the above question is a resounding YES!
I also exhorted retail traders new to quantitative trading to start with the simplest strategies (examples of which are described in this and my previous books). Do simple strategies still work? Or do we all have to become mathematicians or machine learning experts?
My colleagues and I traded some of the strategies described in this book live since it was first published in 2009, and ran true out-of-sample backtests on others, and I was as surprised as they are that many still work after all these years. But the issues of alpha decay, and the even-more-dreaded regime change, are ever threatening. I will talk more about that below.
Speaking of machine learning and artificial intelligence, I didn't really think much of those techniques in my first book. In fact, the only artificial intelligence platform that I described there has gone out of business. But you may hear that AI is everywhere nowadays, and many fundamental advances in AI have been made since then. For example, the dropout technique that gave birth to deep learning achieved fame in 2012 (Gershgorn, ). Should retail traders still avoid AI/ML?
It is as difficult to apply AI/ML to finance in 2021 as it was in 2009, but you may be surprised to hear that we have finally succeeded (Chan, ). We have benefited from other giants in the industry who graciously share their insights and knowledge with everyone (Lpez de Prado, 2018). We, in turn, tried to make it easier for every retail trader (even those who are not programmers) to benefit from this technology by launching predictnow.ai. Here is the spoiler: The key to successfully apply AI/ML to finance is to focus on metalabeling i.e., finding the probability of profit of your own simple basic trading strategy, and not to use it to predict the market directly. Why? Your own trading strategy's past track record is private; no one else is trying to predict its success. Meanwhile, millions of people around the world are watching the same public market, and everyone is trying to predict where it will go. Competition and arbitrage naturally mean that signal-to-noise ratio is very low and predictive successes are few and far in between.
But that's not all. There is another novel use of machine learning that I will discuss in a completely revised of this book.
Despite our luck with the longevity of some of the strategies I described, most arbitrage opportunities eventually fade awaythe notorious alpha decay that professionals like to lament. Alpha decay can be due to competitiontoo many people trading the same strategy, but equally often it is due to regime shift caused by market structure or macroeconomic changes. Adapt and evolve your strategies, or watch them die (Lo, . More so than providing specific strategy examples, I hope my books will also improve your market intuition in making these discretionary decisions.