Max Bramer - Principles of Data Mining
Here you can read online Max Bramer - Principles of Data Mining full text of the book (entire story) in english for free. Download pdf and epub, get meaning, cover and reviews about this ebook. year: 0, publisher: Springer London, London, genre: Children. Description of the work, (preface) as well as reviews are available. Best literature library LitArk.com created for fans of good reading and offers a wide selection of genres:
Romance novel
Science fiction
Adventure
Detective
Science
History
Home and family
Prose
Art
Politics
Computer
Non-fiction
Religion
Business
Children
Humor
Choose a favorite category and find really read worthwhile books. Enjoy immersion in the world of imagination, feel the emotions of the characters or learn something new for yourself, make an fascinating discovery.
- Book:Principles of Data Mining
- Author:
- Publisher:Springer London, London
- Genre:
- Year:0
- Rating:4 / 5
- Favourites:Add to favourites
- Your mark:
- 80
- 1
- 2
- 3
- 4
- 5
Principles of Data Mining: summary, description and annotation
We offer to read an annotation, description, summary or preface (depends on what the author of the book "Principles of Data Mining" wrote himself). If you haven't found the necessary information about the book — write in the comments, we will try to find it.
Principles of Data Mining — read online for free the complete book (whole text) full work
Below is the text of the book, divided by pages. System saving the place of the last page read, allows you to conveniently read the book "Principles of Data Mining" online for free, without having to search again every time where you left off. Put a bookmark, and you can go to the page where you finished reading at any time.
Font size:
Interval:
Bookmark:
- The current NASA Earth observation satellites generate a terabyte (i.e.
bytes) of data every day . This is more than the total amount of data ever transmitted by all previous observation satellites.
- The Human Genome project is storing thousands of bytes for each of several billion genetic bases.
- Many companies maintain large Data Warehouses of customer transactions. A fairly small data warehouse might contain more than a hundred million transactions.
- There are vast amounts of data recorded every day on automatic recording devices, such as credit card transaction files and web logs, as well as non-symbolic data such as CCTV recordings.
- There are estimated to be over 650 million websites, some extremely large.
- There are over 900 million users of Facebook (rapidly increasing), with an estimated 3 billion postings a day.
- It is estimated that there are around 150 million users of Twitter, sending 350 million Tweets each day.
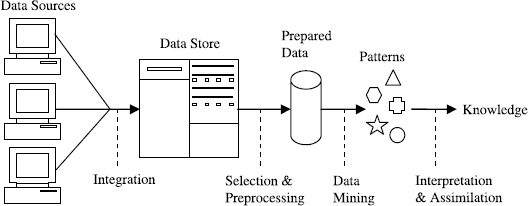
- analysing satellite imagery
- analysis of organic compounds
- automatic abstracting
- credit card fraud detection
- electric load prediction
- financial forecasting
- medical diagnosis
- predicting share of television audiences
- product design
- real estate valuation
- targeted marketing
- text summarisation
- thermal power plant optimisation
- toxic hazard analysis
- weather forecasting
- a supermarket chain mines its customer transactions data to optimise targeting of high value customers
- a credit card company can use its data warehouse of customer transactions for fraud detection
- a major hotel chain can use survey databases to identify attributes of a high-value prospect
- predicting the probability of default for consumer loan applications by improving the ability to predict bad loans
- reducing fabrication flaws in VLSI chips
- data mining systems can sift through vast quantities of data collected during the semiconductor fabrication process to identify conditions that are causing yield problems
- predicting audience share for television programmes, allowing television executives to arrange show schedules to maximise market share and increase advertising revenues
- predicting the probability that a cancer patient will respond to chemotherapy, thus reducing health-care costs without affecting quality of care
- analysing motion-capture data for elderly people
- trend mining and visualisation in social networks.
Font size:
Interval:
Bookmark:
Similar books «Principles of Data Mining»
Look at similar books to Principles of Data Mining. We have selected literature similar in name and meaning in the hope of providing readers with more options to find new, interesting, not yet read works.
Discussion, reviews of the book Principles of Data Mining and just readers' own opinions. Leave your comments, write what you think about the work, its meaning or the main characters. Specify what exactly you liked and what you didn't like, and why you think so.