Leonida Gianfagna - Explainable AI Using Python
Here you can read online Leonida Gianfagna - Explainable AI Using Python full text of the book (entire story) in english for free. Download pdf and epub, get meaning, cover and reviews about this ebook. year: 2021, publisher: Springer, genre: Children. Description of the work, (preface) as well as reviews are available. Best literature library LitArk.com created for fans of good reading and offers a wide selection of genres:
Romance novel
Science fiction
Adventure
Detective
Science
History
Home and family
Prose
Art
Politics
Computer
Non-fiction
Religion
Business
Children
Humor
Choose a favorite category and find really read worthwhile books. Enjoy immersion in the world of imagination, feel the emotions of the characters or learn something new for yourself, make an fascinating discovery.
- Book:Explainable AI Using Python
- Author:
- Publisher:Springer
- Genre:
- Year:2021
- Rating:5 / 5
- Favourites:Add to favourites
- Your mark:
- 100
- 1
- 2
- 3
- 4
- 5
Explainable AI Using Python: summary, description and annotation
We offer to read an annotation, description, summary or preface (depends on what the author of the book "Explainable AI Using Python" wrote himself). If you haven't found the necessary information about the book — write in the comments, we will try to find it.
Explainable AI Using Python — read online for free the complete book (whole text) full work
Below is the text of the book, divided by pages. System saving the place of the last page read, allows you to conveniently read the book "Explainable AI Using Python" online for free, without having to search again every time where you left off. Put a bookmark, and you can go to the page where you finished reading at any time.
Font size:
Interval:
Bookmark:
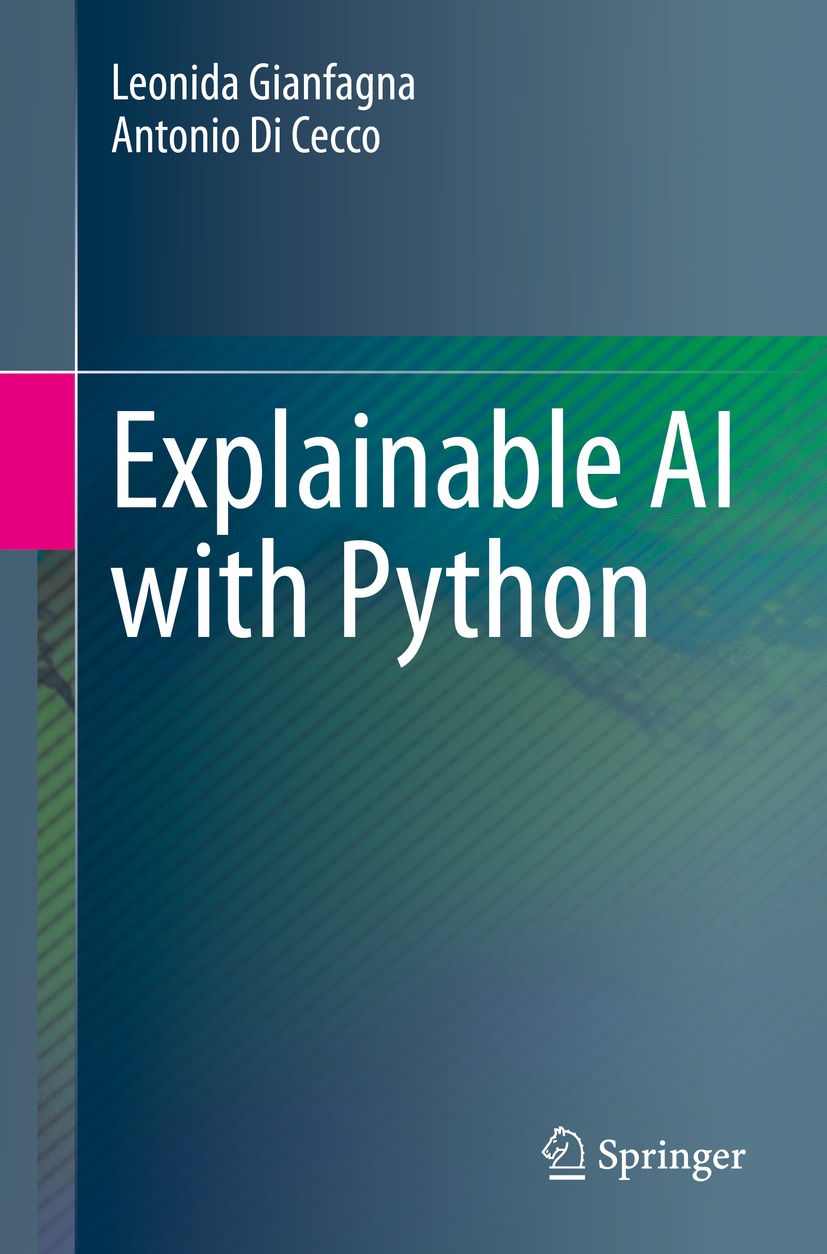
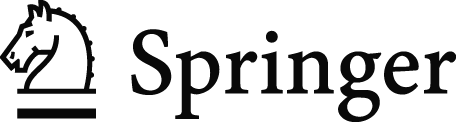
This Springer imprint is published by the registered company Springer Nature Switzerland AG
The registered company address is: Gewerbestrasse 11, 6330 Cham, Switzerland
Everyone knows that debugging is twice as hard as writing a program in the first place.
So if youre as clever as you can be when you write it, how will you ever debug it?
Brian Kernighan
What is Explainable AI in the context of Machine Learning?
Why do we need Explainable AI?
The big picture of how Explainable AI works
For our purposes we place the birth of AI with the seminal work of Alan Turing () in which the author posed the question Can machines think? and the later famous mental experiment proposed by Searle called the Chinese Room.
The point is simple: suppose to have a black-box-based AI system that pretends to speak Chinese in the sense that it can receive questions in Chinese and provide answers in Chinese. Assume also that this agent may pass a Turing test that means it is indistinguishable from a real person that speaks Chinese. Would we be fine on saying that this AI system is capable of speaking Chinese as well? Or do we want more? Do we want the black box to explain itself clarifying some Chinese language grammar?
So, the root of Explainable AI was at the very beginning of Artificial Intelligence, albeit not in the current form as a specific discipline. The key to trust the system as a real Chinese speaker would be to make the system less opaque and explainable as a further requirement besides getting proper answers.
Jumping to our days, it is worth to mention the statement of GO champion Fan Hui commenting the famous 37th move of AlphaGo , the software developed by Google to play GO, that defeated in March 2016 the Korean champion Lee Sedol with a historical result: Its not a human move, Ive never seen a man playing such a move (Metz ). GO is known as a computationally complex game, more complex than chess, and before this result, the common understanding was that it was not a game suitable for a machine to play successfully. But for our purposes and to start this journey, we need to focus on Fan Huis quoted statement. The GO champion could not make sense of the move even after having looked at all the match; he recognized it as brilliant, but he had no way to provide an explanation. So, we have an AI system (AlphaGo) that performed very well (defeating the GO champion), but no explanation of how it worked to win the game; that is where Explainable AI inside the wider Machine Learning and Artificial Intelligence starts to play a critical role.
Before presenting the full landscape, we will give some examples that are less sensationalistic but more practical in terms of understanding what we mean by the fact that most of the current Machine Learning models are opaque and not explainable. And the fil rouge of the book will be to learn in practice leveraging different methods and how to make ML models explainable, that is, to answer the questions What, How, and Why on the results.
Explainable AI (aka XAI) is more than just a buzz word, but it is not easy to provide a definition that includes the different angles to look at the term. Basically speaking, XAI is a set of methods and tools that can be adopted to make ML models understandable to human beings in terms of providing explanations on the results provided by the ML models elaboration.
The first one is about the learning phase.
The second example is more on knowledge discovery.
The third introduces the argument of reliability and robustness against external attacks to the ML model.
One of the most brilliant successes of modern Deep Learning techniques against the traditional approach comes from computer vision. We can train a convolutional neural network (CNN) to understand the difference between different classes of labelled pictures. The applications are probably infinite: we can train a model to discriminate between different kinds of pneumonia RX pictures or teach it to translate sign language into speech. But are the results truly reliable?

ML classification of wolves and dogs (Singh )
Font size:
Interval:
Bookmark:
Similar books «Explainable AI Using Python»
Look at similar books to Explainable AI Using Python. We have selected literature similar in name and meaning in the hope of providing readers with more options to find new, interesting, not yet read works.
Discussion, reviews of the book Explainable AI Using Python and just readers' own opinions. Leave your comments, write what you think about the work, its meaning or the main characters. Specify what exactly you liked and what you didn't like, and why you think so.