Srivastava - Natural Language Processing with Python: Hands-On Labs to Apply Deep Learning Architectures to NLP Applications
Here you can read online Srivastava - Natural Language Processing with Python: Hands-On Labs to Apply Deep Learning Architectures to NLP Applications full text of the book (entire story) in english for free. Download pdf and epub, get meaning, cover and reviews about this ebook. year: 2021, genre: Children. Description of the work, (preface) as well as reviews are available. Best literature library LitArk.com created for fans of good reading and offers a wide selection of genres:
Romance novel
Science fiction
Adventure
Detective
Science
History
Home and family
Prose
Art
Politics
Computer
Non-fiction
Religion
Business
Children
Humor
Choose a favorite category and find really read worthwhile books. Enjoy immersion in the world of imagination, feel the emotions of the characters or learn something new for yourself, make an fascinating discovery.

- Book:Natural Language Processing with Python: Hands-On Labs to Apply Deep Learning Architectures to NLP Applications
- Author:
- Genre:
- Year:2021
- Rating:4 / 5
- Favourites:Add to favourites
- Your mark:
- 80
- 1
- 2
- 3
- 4
- 5
Natural Language Processing with Python: Hands-On Labs to Apply Deep Learning Architectures to NLP Applications: summary, description and annotation
We offer to read an annotation, description, summary or preface (depends on what the author of the book "Natural Language Processing with Python: Hands-On Labs to Apply Deep Learning Architectures to NLP Applications" wrote himself). If you haven't found the necessary information about the book — write in the comments, we will try to find it.
Natural Language Processing with Python: Hands-On Labs to Apply Deep Learning Architectures to NLP Applications — read online for free the complete book (whole text) full work
Below is the text of the book, divided by pages. System saving the place of the last page read, allows you to conveniently read the book "Natural Language Processing with Python: Hands-On Labs to Apply Deep Learning Architectures to NLP Applications" online for free, without having to search again every time where you left off. Put a bookmark, and you can go to the page where you finished reading at any time.
Font size:
Interval:
Bookmark:
Sachin Srivastava
All rights reserved. No part of this book may be reproduced, stored in a retrieval system, or transmitted in any form or by any means, without the prior written permission of the Author and publisher, except in the case of brief quotations embedded in critical articles or reviews.
Every effort has been made in the preparation of this book to ensure the accuracy of the information presented. However, the information contained in this book is sold without warranty, either express or implied. Neither the Author, nor Publisher or its dealers and distributors, will be held liable for any damages caused or alleged to have been caused directly or indirectly by this book.
Author has endeavored to provide trademark information about all of the companies and products mentioned in this book by the appropriate use of capitals. However, he cannot guarantee the accuracy of this information.Intelligent digital assistants in form of voice transcription, machine translation, conversational agents, and sentiment analysis are ubiquitously applied across various domains to facilitate human-computer interactions. Chatbot's are becoming an integrated part of any website; while virtual assistants are gaining more popularity in homes and office spaces. Consequently, given numerous existing resources that contain these topics under the notion of natural language processing (NLP ), a contribution that covers a comprehensive guide to the fundamentals and the state-of-the-art, and on top of that includes practical examples with most popular frameworks and tool kits, is a rare find.
When I was first asked to write the foreword for this book, I was very delighted to convey the level of passion that drove the authors to write it, and yet was uncertain on how to best present an excellent source of up-to-date knowledge and a practical handbook of machine learning (ML ) for NLP that truly stands out from the crowd.
The leading authors' reputation in ML needs no further explanation. With both equipped with academic education in world-class universities and many years of leadership in ML development, Rajesh and Rajalingappa's qualification to lead the authorship of this book is confirmed. I have come to know them not only as knowledgeable individuals but also as passionate educators who convey the most sophisticated concepts in the simplest words. Raja's passion to help start-ups get off the ground and to offer his expertise to young companies with an open heart is admirable. I'm sure that, even as readers of this book, you can approach him for questions and be sure of a convincing response.
The book itself is very well organized and written to serve the purpose. From concrete examples to explain the fundamentals to code snippets for guiding readers with different levels of deep learning backgrounds, the chapters are structured to retain full attention of the reader all throughout. You will discover an exciting combination of the most popular techniques and state-of-the-art approaches to text processing and classification.
By reading this book, you can expect to learn how to perform common NLP tasks, such as preprocessing and exploratory analysis of text using the Python's Natural Language Toolkit. You will understand deep neural networks, Google's TensorFlow framework, and the building blocks of recurrent neural networks (RNNs ), including Long ShortTerm Memory. And you will grasp the notion of word embeddings to allow for semantics in context.
Having taught the basics, the book further takes you through the development of architectures and deep neural network models for a variety of applications, including text classification, text generation and summarization, question-answering, language translation, speech recognition, and text-to-speech.
The book concludes by presenting various methods to deploy a trained model for NLP tasks, on a variety of platforms. By the end of your experience with the book, you will have learned the data science paradigm in NLP and can hopefully deploy deep learning models in commercial applications in a production environment as the authors envisioned.
Sachin SrivastavaAbout the Author
Sachin Srivastava is an ML developer. Previously, he developed ML solutions for smart city development in areas such as passenger flow analysis in public transit systems and optimization of energy consumption in buildings when working with Centre for Social Innovation. He has published papers in conferences and has pending patents in storage and ML..
I would like to thank my parents: Vijay and Shobha for their support and understanding while writing this book.
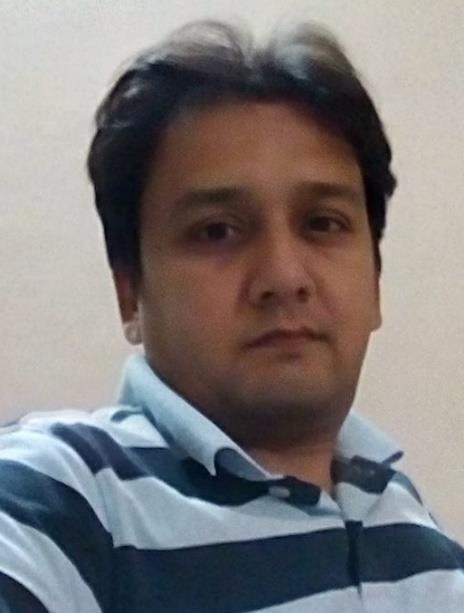
Auchitya Khinchi is an associate senior consultant. He has played dynamic roles during his career in developing ERP, search engines with Python, Single-Page Applications (SPA ), and mobile apps with Node.js, MongoDB, and AngularJS.
He received multiple awards in recognition of his valuable contributions to the team and the company.Chapter 1: Getting Started 8
Basic concepts and terminologies in NLP 8
Text corpus or corpora 9
Paragraph 9
Sentences 9
Phrases and words 10
N-grams 10
Bag-of-words 10
Applications of NLP 11
Analyzing sentiment 11
Recognizing named entities 12
Linking entities 13
Translating text 14
Natural Language Inference 16
Semantic Role Labeling 16
Relation extraction 17
SQL query generation, or semantic parsing 17
Machine Comprehension 19
Textual Entailment 21
Coreference resolution 21
Searching 22
Question answering and chatbots 23
Converting text-to-voice 23
Converting voice-to-text 25
Speaker identification 25
Spoken dialog systems 26
Other applications 26
Summary 27
Chapter 2: Text Classification and POS Tagging Using NLTK 28
Installing NLTK and its modules 28
Text preprocessing and exploratory analysis 30
Tokenization 30
Stemming 32
Removing stop words 33
Exploratory analysis of text 34
POS tagging 37
What is POS tagging? 38
Applications of POS tagging 39
Training a POS tagger 40
Training a sentiment classifier for movie reviews 44
Training a bag-of-words classifier 49
Summary 51
Chapter 3: Deep Learning and TensorFlow 52
Deep learning 52
Perceptron 53
Activation functions 53
Sigmoid 54
Hyperbolic tangent 55
Rectified linear unit 55
Neural network 56
One-hot encoding 57
Softmax 57
Cross-entropy 58
Training neural networks 58
Backpropagation 58
Gradient descent 59
Stochastic gradient descent 60
Regularization techniques 60
Dropout 61
Batch normalization 61
L1 and L2 normalization 61
Convolutional Neural Network 62
Kernel 62
Max pooling 63
Recurrent neural network 63
Long-Short Term Memory 64
TensorFlow 64
General Purpose Graphics Processing Unit 64
CUDA 65
cuDNN 66
Installation 66
Hello world! 66
Adding two numbers 67
TensorBoard 68
The Keras library 69
Summary 69
Chapter 4: Semantic Embedding Using Shallow Models 70
Word vectors 70
The classical approach 71
Word2vec 72
The CBOW model 74
The skip-gram model 74
A comparison of skip-gram and CBOW model architectures 74
Font size:
Interval:
Bookmark:
Similar books «Natural Language Processing with Python: Hands-On Labs to Apply Deep Learning Architectures to NLP Applications»
Look at similar books to Natural Language Processing with Python: Hands-On Labs to Apply Deep Learning Architectures to NLP Applications. We have selected literature similar in name and meaning in the hope of providing readers with more options to find new, interesting, not yet read works.
Discussion, reviews of the book Natural Language Processing with Python: Hands-On Labs to Apply Deep Learning Architectures to NLP Applications and just readers' own opinions. Leave your comments, write what you think about the work, its meaning or the main characters. Specify what exactly you liked and what you didn't like, and why you think so.