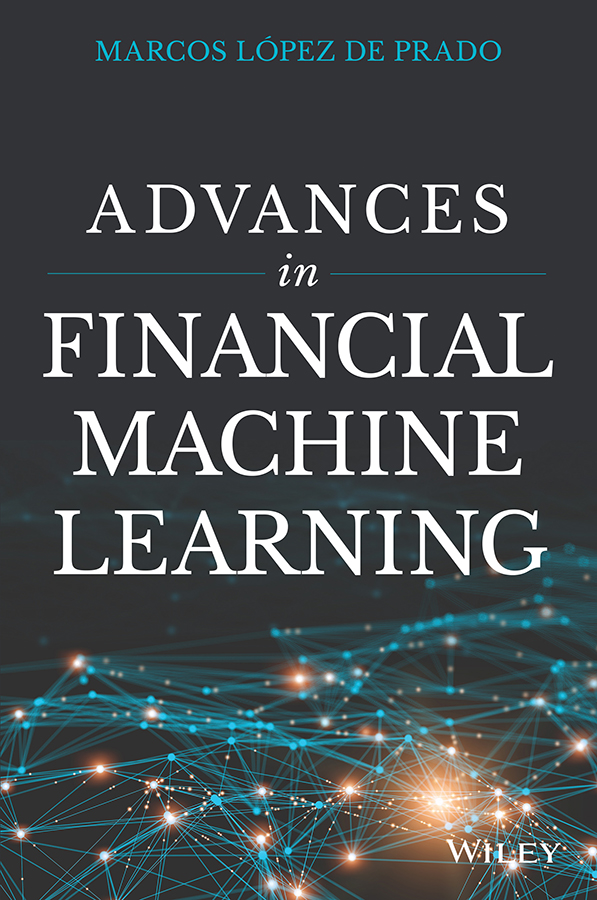
Praise for Advances in Financial Machine Learning
In his new book Advances in Financial Machine Learning, noted financial scholar Marcos Lpez de Prado strikes a well-aimed karate chop at the naive and often statistically overfit techniques that are so prevalent in the financial world today. He points out that not only are business-as-usual approaches largely impotent in todays high-tech finance, but in many cases they are actually prone to lose money. But Lpez de Prado does more than just expose the mathematical and statistical sins of the finance world. Instead, he offers a technically sound roadmap for finance professionals to join the wave of machine learning. What is particularly refreshing is the authors empirical approachhis focus is on real-world data analysis, not on purely theoretical methods that may look pretty on paper but which, in many cases, are largely ineffective in practice. The book is geared to finance professionals who are already familiar with statistical data analysis techniques, but it is well worth the effort for those who want to do real state-of-the-art work in the field.
Dr. David H. Bailey, former Complex Systems Lead,
Lawrence Berkeley National Laboratory. Co-discoverer of the
BBP spigot algorithm
Finance has evolved from a compendium of heuristics based on historical financial statements to a highly sophisticated scientific discipline relying on computer farms to analyze massive data streams in real time. The recent highly impressive advances in machine learning (ML) are fraught with both promise and peril when applied to modern finance. While finance offers up the nonlinearities and large data sets upon which ML thrives, it also offers up noisy data and the human element which presently lie beyond the scope of standard ML techniques. To err is human, but if you really want to f**k things up, use a computer. Against this background, Dr. Lpez de Prado has written the first comprehensive book describing the application of modern ML to financial modeling. The book blends the latest technological developments in ML with critical life lessons learned from the authors decades of financial experience in leading academic and industrial institutions. I highly recommend this exciting book to both prospective students of financial ML and the professors and supervisors who teach and guide them.
Prof. Peter Carr, Chair of the Finance and Risk Engineering
Department, NYU Tandon School of Engineering
Marcos is a visionary who works tirelessly to advance the finance field. His writing is comprehensive and masterfully connects the theory to the application. It is not often you find a book that can cross that divide. This book is an essential read for both practitioners and technologists working on solutions for the investment community.
Landon Downs, President and Cofounder, 1QBit
Academics who want to understand modern investment management need to read this book. In it, Marcos Lpez de Prado explains how portfolio managers use machine learning to derive, test, and employ trading strategies. He does this from a very unusual combination of an academic perspective and extensive experience in industry, allowing him to both explain in detail what happens in industry and to explain how it works. I suspect that some readers will find parts of the book that they do not understand or that they disagree with, but everyone interested in understanding the application of machine learning to finance will benefit from reading this book.
Prof. David Easley, Cornell University. Chair of the
NASDAQ-OMX Economic Advisory Board
For many decades, finance has relied on overly simplistic statistical techniques to identify patterns in data. Machine learning promises to change that by allowing researchers to use modern nonlinear and highly dimensional techniques, similar to those used in scientific fields like DNA analysis and astrophysics. At the same time, applying those machine learning algorithms to model financial problems would be dangerous. Financial problems require very distinct machine learning solutions. Dr. Lpez de Prados book is the first one to characterize what makes standard machine learning tools fail when applied to the field of finance, and the first one to provide practical solutions to unique challenges faced by asset managers. Everyone who wants to understand the future of finance should read this book.
Prof. Frank Fabozzi, EDHEC Business School. Editor of
The Journal of Portfolio Management
This is a welcome departure from the knowledge hoarding that plagues quantitative finance. Lpez de Prado defines for all readers the next era of finance: industrial scale scientific research powered by machines.
John Fawcett, Founder and CEO, Quantopian
Marcos has assembled in one place an invaluable set of lessons and techniques for practitioners seeking to deploy machine learning techniques in finance. If machine learning is a new and potentially powerful weapon in the arsenal of quantitative finance, Marcoss insightful book is laden with useful advice to help keep a curious practitioner from going down any number of blind alleys, or shooting oneself in the foot.
Ross Garon, Head of Cubist Systematic Strategies. Managing
Director, Point72 Asset Management
The first wave of quantitative innovation in finance was led by Markowitz optimization. Machine Learning is the second wave, and it will touch every aspect of finance. Lpez de Prados Advances in Financial Machine Learning is essential for readers who want to be ahead of the technology rather than being replaced by it.
Prof. Campbell Harvey, Duke University. Former President of
the American Finance Association
The complexity inherent to financial systems justifies the application of sophisticated mathematical techniques. Advances in Financial Machine Learning is an exciting book that unravels a complex subject in clear terms. I wholeheartedly recommend this book to anyone interested in the future of quantitative investments.
Prof. John C. Hull, University of Toronto. Author of
Options, Futures, and other Derivatives
Prados book clearly illustrates how fast this world is moving, and how deep you need to dive if you are to excel and deliver top of the range solutions and above the curve performing algorithms... Prados book is clearly at the bleeding edge of the machine learning world.
Irish Tech News
Financial data is special for a key reason: The markets have only one past. There is no control group, and you have to wait for true out-of-sample data. Consequently, it is easy to fool yourself, and with the march of Moores Law and the new machine learning, its easier than ever. Lpez de Prado explains how to avoid falling for these common mistakes. This is an excellent book for anyone working, or hoping to work, in computerized investment and trading.
Dr. David J. Leinweber, Former Managing Director, First Quadrant.
Author of Nerds on Wall Street: Math, Machines and Wired Markets
In his new book, Dr. Lpez de Prado demonstrates that financial machine learning is more than standard machine learning applied to financial datasets. It is an important field of research in its own right. It requires the development of new mathematical tools and approaches, needed to address the nuances of financial datasets. I strongly recommend this book to anyone who wishes to move beyond the standard Econometric toolkit.
Dr. Richard R. Lindsey, Managing Partner, Windham Capital Management.
Former Chief Economist, U.S. Securities and Exchange Commission
Next page