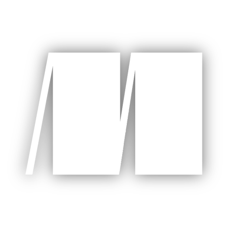
MEAP Edition
Manning Early Access Program
Machine Learning Algorithms in Depth
Version 3
Copyright 2023 Manning Publications
Manning Publications Co. We welcome reader comments about anything in the manuscript - other than typos and other simple mistakes.
These will be cleaned up during production of the book by copyeditors and proofreaders.
For more information on this and other Manning titles go to
manning.com
welcome
Thank you for purchasing the MEAP for Machine Learning Algorithms in Depth. This book will take you on a journey from mathematical derivation to software implementation of some of the most intriguing algorithms in ML.
This book dives into the design of ML algorithms from scratch. Throughout the book, you will develop mathematical intuition for classic and modern ML algorithms, learn the fundamentals of Bayesian inference and deep learning, as well as the data structures and algorithmic paradigms in ML.
Understanding ML algorithms from scratch will help you choose the right algorithm for the task, explain the results, troubleshoot advanced problems, extend an algorithm to a new application, and improve performance of existing algorithms.
Some of the prerequisites for reading this book include basic level of programming in Python and intermediate level of understanding of linear algebra, applied probability and multivariate calculus.
My goal in writing this book is to distill the science of ML and present it in a way that will convey intuition and inspire the reader to self-learn, innovate and advance the field. Your input is important. Id like to encourage you to post questions and comments in the to help improve presentation of the material.
Thank you again for your interest and welcome to the world of ML algorithms!
Vadim Smolyakov
In this book
1 Machine Learning Algorithms
This chapter covers
- Types of ML algorithms
- Importance of learning algorithms from scratch
- Introduction to Bayesian Inference and Deep Learning
- Software implementation of machine learning algorithms from scratch
An algorithm is a sequence of steps required to achieve a particular task. An algorithm takes an input, performs a sequence of operations and produces a desired output. The simplest example of an algorithm is sorting: given a list of integers, we perform a sequence of operations to produce a sorted list. A sorted list enables us to organize information better and find answers in our data.
Two popular questions to ask about an algorithm is how fast does it run (run-time complexity) and how much memory does it take (memory complexity) for an input of size n
. For example, a comparison-based sort, as well see later, has O(nlogn)
run-time complexity and requires O(n)
memory storage.
There are many approaches to sorting, and in each case, in the classic algorithmic paradigm, the algorithm designer creates a set of instructions. Imagine a world where you can learn the instructions based on a sequence of input and output examples available to you. This is a setting of ML algorithmic paradigm. Similar to how a human brain learns, when we are playing connect-the-dots game or sketching a nature landscape, we are comparing the desired output with what we have at each step and filling in the gaps. This in broad strokes is what (supervised) machine learning (ML) algorithms do. During training, ML algorithms are learning the rules (e.g. classification boundaries) based on training examples by optimizing an objective function. During testing, ML algorithms apply previously learned rules to new input data points to give a prediction as shown in Figure 1.1
1.1 Types of ML Algorithms
Lets unpack the previous paragraph a little bit and introduce some notation. This book focuses on machine learning algorithms that can be grouped together in the following categories: supervised learning, unsupervised learning and deep learning. In supervised learning, the task is to learn a mapping f
from inputs x
to outputs given a training dataset D = {(x
1,y
1),,(x
n,y
n)}
of n
input-output pairs. In other words, we are given n
examples of what the output should look like given the input. The output y
is also often referred to as the label, and it is the supervisory signal that tells our algorithm what the correct answer is.
Figure 1.1 Supervised Learning: Training (left) and Testing (right)
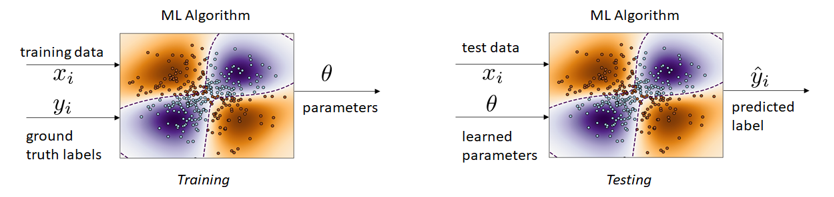
Supervised learning can be sub-divided into classification and regression based on the quantity we are trying to predict. If our output y
is a discrete quantity (e.g. K distinct classes) we have a classification problem. On the other hand, if our output y
is a continuous quantity (e.g. a real number such as stock price) we have a regression problem.
Thus, the nature of the problem changes based on the quantity y
we are trying to predict. We want to get as close as possible to the ground truth value of y.
A common way to measure performance or closeness to ground truth is the loss function. The loss function is computing a distance between the prediction and the true label. Let y = f(x; )
be our ML algorithm that maps input examples x
to output labels y,
parameterized by , where captures all the learnable parameters of our ML algorithm. Then, we can write our classification loss function as follows in Equation 1.1:
Equation 1.1 Loss function for classification

Where 1[]
is an indicator function, which is equal to 1 when the argument inside is true and 0 otherwise. What the expression above says is we are adding up all the instances in which our prediction f(x
i; )
did not match the ground truth label y
i and we are dividing by the total number of examples n
. In other words, we are computing an average misclassification rate. Our goal is to minimize the loss function, i.e. find a set of parameters , that make the misclassification rate as close to zero as possible. Note that there are other alternative loss functions for classification such as cross entropy that we will look into in later chapters.
For continuous labels or response variables, a common loss function is the Mean Square Error (MSE), defined as follows in Equation 1.2:
Equation 1.2 Loss function for regression

Essentially, we are subtracting our prediction from the ground truth label, squaring it and aggregating the result as an average over all data points. By taking the square we are eliminating the possibility of negative loss values, which would impact our summation.
One of the central goals of machine learning is to be able to generalize to unseen examples. We want to achieve high accuracy (low loss) on not just the training data (which is already labelled) but on new, unseen, test data examples. This generalization ability is what makes machine learning so attractive: if we can design ML algorithms that can see outside their training box, well be one step closer to Artificial General Intelligence (AGI).
Next page