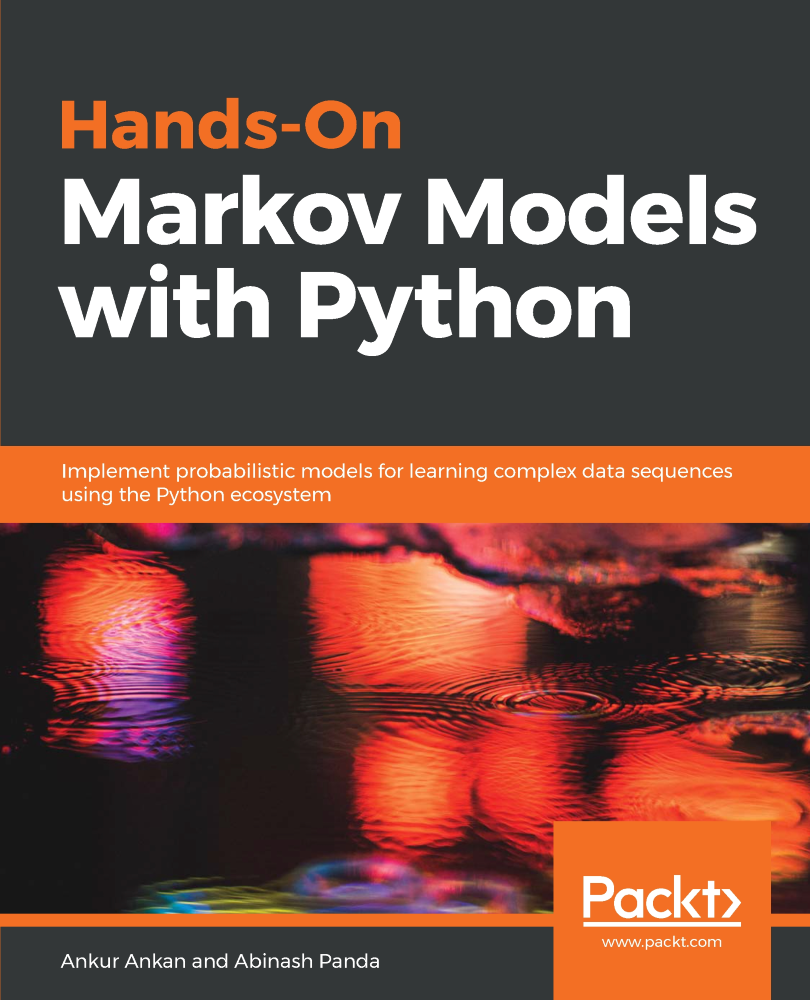
Hands-On Markov Models with Python
Implement probabilistic models for learning complex data sequences using the Python ecosystem
Ankur Ankan
Abinash Panda
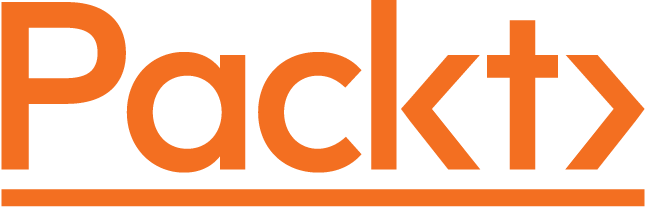
BIRMINGHAM - MUMBAI
Hands-On Markov Models with Python
Copyright 2018 Packt Publishing
All rights reserved. No part of this book may be reproduced, stored in a retrieval system, or transmitted in any form or by any means, without the prior written permission of the publisher, except in the case of brief quotations embedded in critical articles or reviews.
Every effort has been made in the preparation of this book to ensure the accuracy of the information presented. However, the information contained in this book is sold without warranty, either express or implied. Neither the author(s), nor Packt Publishing or its dealers and distributors, will be held liable for any damages caused or alleged to have been caused directly or indirectly by this book.
Packt Publishing has endeavored to provide trademark information about all of the companies and products mentioned in this book by the appropriate use of capitals. However, Packt Publishing cannot guarantee the accuracy of this information.
Commissioning Editor: Sunith Shetty
Acquisition Editor: Varsha Shetty
Content Development Editor: Karan Thakkar
Technical Editor: Sagar Sawant
Copy Editor: Safis Editing
Project Coordinator: Nidhi Joshi
Proofreader: Safis Editing
Indexer: Aishwarya Gangawane
Graphics: Jisha Chirayil
Production Coordinator: Shraddha Falebhai
First published: September 2018
Production reference: 1250918
Published by Packt Publishing Ltd.
Livery Place
35 Livery Street
Birmingham
B3 2PB, UK.
ISBN 978-1-78862-544-9
www.packtpub.com
mapt.io
Mapt is an online digital library that gives you full access to over 5,000 books and videos, as well as industry leading tools to help you plan your personal development and advance your career. For more information, please visit our website.
Why subscribe?
Spend less time learning and more time coding with practical eBooks and Videos from over 4,000 industry professionals
Improve your learning with Skill Plans built especially for you
Get a free eBook or video every month
Mapt is fully searchable
Copy and paste, print, and bookmark content
packt.com
Did you know that Packt offers eBook versions of every book published, with PDF and ePub files available? You can upgrade to the eBook version at www.packt.com and as a print book customer, you are entitled to a discount on the eBook copy. Get in touch with us at customercare@packtpub.com for more details.
At www.packt.com , you can also read a collection of free technical articles, sign up for a range of free newsletters, and receive exclusive discounts and offers on Packt books and eBooks.
Contributors
About the authors
Ankur Ankan is a BTech graduate from IIT (BHU), Varanasi. He is currently working in the field of data science. He is an open source enthusiast and his major work includes starting pgmpy with four other members. In his free time, he likes to participate in Kaggle competitions.
Abinash Panda has been a data scientist for more than 4 years. He has worked at multiple early-stage start-ups and helped them build their data analytics pipelines. He loves to munge, plot, and analyze data. He has been a speaker at Python conferences. These days, he is busy co-founding a start-up. He has contributed to books on probabilistic graphical models by Packt Publishing.
About the reviewer
Abdullah al Mamun is a professional software engineer and researcher. He has completed his graduation from rajshahi university of engineering & technology (RUET) and BSc in Computer Science and Engineering (CSE). Currently, he is working as a Senior Executive Officer of the Software section in Primeasia University. As a professional software engineer, he has experience in object-oriented design, software architectures, design patterns, test-driven development, and project management. Also, he is interested in research in the fields of artificial intelligence, neural network, pattern recognition, and machine learning. His research has been published in different international journals and conferences, including IEEE.
This Hands-On Markov Models with Python book is a good reference for those involved with teaching and also research and development. In this book, the authors explain the Hidden Markov Model and its real-time application, illustrated with Python source code. I would like to express my gratitude toward the books authors and Packt Publishing for their wonderful collaboration.
Packt is searching for authors like you
If you're interested in becoming an author for Packt, please visit authors.packtpub.com and apply today. We have worked with thousands of developers and tech professionals, just like you, to help them share their insight with the global tech community. You can make a general application, apply for a specific hot topic that we are recruiting an author for, or submit your own idea.
Preface
Using Hidden Markov Models (HMMs) is a technique for modeling Markov processes with unobserved states. They are a special case of Dynamic Bayesian Networks (DBNs) but have been found to perform well in a wide range of problems. One of the areas where HMMs are used a lot is speech recognition because HMMs are able to provide a very natural way to model speech data. This book starts by introducing the theoretical aspects of HMMs from the basics of probability theory, and then talks about the different applications of HMMs.
Who this book is for
A basic understanding of probability theory, linear algebra, and calculus will make reading this book a lot easier. For the code examples, basic familiarity with Python programming is expected.
What this book covers
, Introduction to Markov Process , starts with a discussion of basic probability theory, and then introduces Markov chains. The chapter also talks about the different types of Markov chain classifying based on continuous or discrete states and time intervals.
, Hidden Markov Models , builds on the concept of Markov processes and DBNs to introduce the concepts of the HMM.
, State Inference Predicting the States , introduces algorithms that can be used to predict the states of a defined HMM. The chapter introduces the Forward algorithm, the backward algorithm, the forward-backward algorithm, and the Viterbi algorithm.
, Parameter Inference Using Maximum Likelihood , discusses the basics of maximum likelihood learning. The chapter then moves on to applying maximum likelihood learning in the case of HMMs and introduces the Viterbi learning algorithm and Baum-Welch algorithm.
Next page