Laura Isabel Galindez Olascoaga , Wannes Meert and Marian Verhelst
Hardware-Aware Probabilistic Machine Learning Models
Learning, Inference and Use Cases
1st ed. 2021
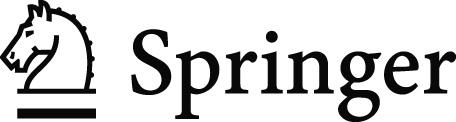
Logo of the publisher
Laura Isabel Galindez Olascoaga
Electrical Engineering, KU Leuven, Leuven, Belgium
Wannes Meert
Computer Science, KU Leuven, Leuven, Belgium
Marian Verhelst
Electrical Engineering, KU Leuven, Leuven, Belgium
ISBN 978-3-030-74041-2 e-ISBN 978-3-030-74042-9
https://doi.org/10.1007/978-3-030-74042-9
The Editor(s) (if applicable) and The Author(s), under exclusive license to Springer Nature Switzerland AG 2021
This work is subject to copyright. All rights are solely and exclusively licensed by the Publisher, whether the whole or part of the material is concerned, specifically the rights of translation, reprinting, reuse of illustrations, recitation, broadcasting, reproduction on microfilms or in any other physical way, and transmission or information storage and retrieval, electronic adaptation, computer software, or by similar or dissimilar methodology now known or hereafter developed.
The use of general descriptive names, registered names, trademarks, service marks, etc. in this publication does not imply, even in the absence of a specific statement, that such names are exempt from the relevant protective laws and regulations and therefore free for general use.
The publisher, the authors and the editors are safe to assume that the advice and information in this book are believed to be true and accurate at the date of publication. Neither the publisher nor the authors or the editors give a warranty, expressed or implied, with respect to the material contained herein or for any errors or omissions that may have been made. The publisher remains neutral with regard to jurisdictional claims in published maps and institutional affiliations.
This Springer imprint is published by the registered company Springer Nature Switzerland AG
The registered company address is: Gewerbestrasse 11, 6330 Cham, Switzerland
Preface
Throughout the last decade, cloud-computing paradigms have proven successful at exploiting the opportunities and addressing the challenges of the ever-increasing number of electronic devices populating our environment. However, the privacy, latency, and efficiency concerns of this approach have motivated users and developers to seek computing paradigms that remain closer to the data-collecting devices, otherwise known as edge devices.
One of the main challenges to overcome in the quest towards edge-computing is the significant energy and computational bandwidth constraints of the involved devices, which tend to be battery operated and portable, especially when devices have to execute complex algorithms relying on large amounts of high-quality sensory data, such as in the case with machine learning applications. Furthermore, smart portable devices are prone to dynamically changing environmental conditions and noise, and the sensory data they rely on is inherently uncertain.
Probabilistic models constitute a suitable approach to address some of these challenges, since they can represent the inherent uncertainty of edge applications, and are robust to noise and missing data. However, their implementation in resource-constrained edge devices has not been studied extensively, unlike other machine learning paradigms, such as neural networks, which have already seen tremendous progress in this research field.
This book proposes to endow probabilistic models with hardware-awareness, in an attempt to enable their efficient implementation in resource-constrained edge devices. These models can represent scalable properties of the devices that host them, such as the quality with which their sensors operate, or the complexity of the inference algorithm they ought to perform.
The strategies proposed herewith can exploit these models to evaluate the impact that a specific device configuration may have on the machine learning tasks resource consumption and performance, with the overarching goal of balancing the two optimally. The proposed models can also consider various sub-systems and their properties holistically, bringing about resource-saving opportunities that other resource-aware approaches fail to uncover. Specifically, this book focuses on augmenting Bayesian networks and probabilistic circuits with hardware-awareness. These models performance is empirically evaluated in several use cases that consider different types of systems and various scalable properties. The range of use cases proves to benefit from this thesiss contributions, with the potential of attaining significant resource-saving opportunities with minimal accuracy losses at application time.
Laura Isabel Galindez Olascoaga
Wannes Meert
Marian Verhelst
Leuven, Belgium
June 2019
Acknowledgements
We would like to acknowledge several people and institutions for their valuable contributions to this work.
We thank Nilesh Ahuja, Herman Bruyninckx, Jesse Davis, Guy Van den Broeck, and Patrick Wambacq for their feedback on previous versions of this manuscript and for all the engaging and inspiring discussions throughout the last years. We would also like to acknowledge our collaborators contributions in the articles discussed throughout this book: Martin Andraud, Komail Badami, Steven Lauwereins, and Nimish Shah.
Finally, we thank Intel for their financial support, as well as the European Research Council for their funding under the Re-SENSE project grant number ERC-2016-STG-71503.
Contents
The Author(s), under exclusive license to Springer Nature Switzerland AG 2021
L. I. Galindez Olascoaga et al. Hardware-Aware Probabilistic Machine Learning Models https://doi.org/10.1007/978-3-030-74042-9_1
1. Introduction
Laura Isabel Galindez Olascoaga
(1)
Electrical Engineering, KU Leuven, Leuven, Belgium
(2)
Computer Science, KU Leuven, Leuven, Belgium
Keywords
Internet of Things Extreme-edge computing Resource-efficient machine learning Machine learning pipeline Problem statement Introduction
The last decades have witnessed an explosion in the number of electronic devices populating our environment. As foreshadowed by Gordon E. Moore in 1965 [], this phenomenon owes to the steady decrease in computing costs facilitated by semiconductor technology scaling. Today, electronic devices are ingrained in every aspect of our lives. From the moment we wake up to our smartphone alarms, through daily commutes guided by our cars navigation systems, to the end of the day, relaxing to a good book loaded on our e-readers.
The computing advancement enabling such a scene of device ubiquity has also inspired the proposal of visionary technological paradigms such as the Internet-of-Things (IoT). Kevin Ashton devised the IoT concept in 1999 in an effort to link the then new idea of RFID to the then hot topic of the Internet [].
One of the byproducts of the technological revolutions described above is the tremendous amount of data available today, gathered by the devices connected to the IoT and by the ubiquitous mass of personal computers and smartphones. The so-called