Studies in Autonomic, Data-driven and Industrial Computing
Series Editors
Swagatam Das
Indian Statistical Institute, Kolkata, West Bengal, India
Jagdish Chand Bansal
South Asian University, Chanakyapuri, India
The book series Studies in Autonomic, Data-driven and Industrial Computing (SADIC) aims at bringing together valuable and novel scientific contributions that address new theories and their real world applications related to autonomic, data-driven, and industrial computing. The area of research covered in the series includes theory and applications of parallel computing, cyber trust and security, grid computing, optical computing, distributed sensor networks, bioinformatics, fuzzy computing and uncertainty quantification, neurocomputing and deep learning, smart grids, data-driven power engineering, smart home informatics, machine learning, mobile computing, internet of things, privacy preserving computation, big data analytics, cloud computing, blockchain and edge computing, data-driven green computing, symbolic computing, swarm intelligence and evolutionary computing, intelligent systems for industry 4.0, as well as other pertinent methods for autonomic, data-driven, and industrial computing.
The series will publish monographs, edited volumes, textbooks and proceedings of important conferences, symposia and meetings in the field of autonomic, data-driven and industrial computing.
More information about this series at https://link.springer.com/bookseries/16624
Editors
Mahdi Khosravy , Isao Echizen and Noboru Babaguchi
Frontiers in Fake Media Generation and Detection
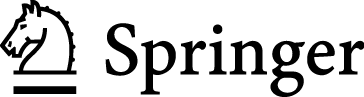
Logo of the publisher
Editors
Mahdi Khosravy
Cross Compass, Cross Labs, Tokyo, Japan
Isao Echizen
Information and Society Research Division, National Institute of Informatics (NII), Tokyo, Japan
Noboru Babaguchi
Graduate School of Engineering, Osaka University, Suita Osaka, Japan
ISSN 2730-6437 e-ISSN 2730-6445
Studies in Autonomic, Data-driven and Industrial Computing
ISBN 978-981-19-1523-9 e-ISBN 978-981-19-1524-6
https://doi.org/10.1007/978-981-19-1524-6
The Editor(s) (if applicable) and The Author(s), under exclusive license to Springer Nature Singapore Pte Ltd. 2022
This work is subject to copyright. All rights are solely and exclusively licensed by the Publisher, whether the whole or part of the material is concerned, specifically the rights of translation, reprinting, reuse of illustrations, recitation, broadcasting, reproduction on microfilms or in any other physical way, and transmission or information storage and retrieval, electronic adaptation, computer software, or by similar or dissimilar methodology now known or hereafter developed.
The use of general descriptive names, registered names, trademarks, service marks, etc. in this publication does not imply, even in the absence of a specific statement, that such names are exempt from the relevant protective laws and regulations and therefore free for general use.
The publisher, the authors, and the editors are safe to assume that the advice and information in this book are believed to be true and accurate at the date of publication. Neither the publisher nor the authors or the editors give a warranty, expressed or implied, with respect to the material contained herein or for any errors or omissions that may have been made. The publisher remains neutral with regard to jurisdictional claims in published maps and institutional affiliations.
This Springer imprint is published by the registered company Springer Nature Singapore Pte Ltd.
The registered company address is: 152 Beach Road, #21-01/04 Gateway East, Singapore 189721, Singapore
Preface
With the spread of smartphones and social media, anyone can now share media such as images, videos, sounds, and texts. Artificial intelligence (AI) technologies greatly enhance the convenience and value of social media, making it easy to analyze user preferences from a large amount of data as well as to perform media processing such as machine translation and speech-to-text conversion in accordance with user needs. However, with the evolution of AI technology and the enrichment of computer resources stemming from the ability to acquire more information related to human-recognition modalities such as the face, speech, body, and natural language, malicious actors can now generate fake media such as fake facial images, fake speech, and fake messages that can pass for the real thing. The generation of fake media has thus become a serious social problem.
The appearance and spread of the novel coronavirus infection (COVID-19) led to the generation and spread on social media of fake news regarding preventive and therapeutic methods without scientific basis. Such an infodemic of uncertain information can cause anxiety and confusion in society. We can easily envision an enterprising criminal group with a clear intention using AI to readily generate fake media that can pass for the real thing and then spreading them on social media to create confusion and doubt. To achieve a healthy human-centered cyber society, it is essential to improve the reliability of information by appropriately dealing with such threats.
This book, Frontiers in Fake Media Generation and Detection, describes recent advances in the generation and detection of fake media as well as techniques for defending against skillfully crafted fake media. The ultimate purpose of the research direction presented in this book is to build up a healthy human-centered cyber society protected against fake media by an iron dome. The book presents recent advances in cyber society promotion based on social information technology and techniques for guaranteeing media reliability. Advances in detection and prevention of sophisticated attacks by fake media are also presented. The book focuses on (1) the application of deep generative models for generating fake media and (2) the techniques for detecting and defending against fake media. Its 11 chapters are grouped into four parts: (i) Introduction (Chapters ).
In chapter , Dilrukshi Gamage et al. first provide comprehension of deepfakes in connection with their societal implications through a systematic literature review and then provide an empirical understanding of the societal implications of deepfakes by analyzing Reddit conversations related to deepfakes from 2018 to 2021.
Maung Maung April Pyone et al. present a comprehensive survey of the state-of-the-art adversarial examples and defenses in chapter . Adversarial examples are greatly important when DNNs are used for security-sensitive applications such as self-driving vehicles, healthcare, and finance.
In addition to the above-mentioned comprehensive survey, Minoru Kuribayashi explains adversarial attacks and defenses in chapters , Trung-Nghia Le et al. explore the evolution of and challenges in deepfake generation and detection and discuss possible ways to improve the robustness of deepfake detection for a wide variety of media.
Jeongwoo Lim et al. in chapter propose a method for generating spoofing messages that appear to be based on the experiences and points of interest (PoIs) selected in accordance with the estimated interests of the target user. Deep-learning-based spoofing message generators can be trained to mimic the writing style of the target user. Besides research on skillfully generating fake media, the chapter covers research on detecting such media, which is getting more important.