CHAPTER 1

NoSQL: Cassandra Basics
The purpose of this chapter is to discuss NoSQL, let users dive into NoSQL elements, and then introduce big data problems, distributed database concepts, and finally Cassandra concepts. Topics covered in this chapter are:
- NoSQL introduction
- CAP theorem
- Data distribution concepts
- Big data problems
- Cassandra configurations
- Cassandra storage architecture
- Setup and installation
- Logging with Cassandra
The intent of the detailed introductory chapter is to dive deep into the NoSQL ecosystem by discussing problems and solutions, such as distributed programming concepts, which can help in solving scalability, availability, and other data-related problems.
This chapter will introduce the reader to Cassandra and discuss Cassandras storage architecture, various other configurations, and the Cassandra cluster setup over local and AWS boxes.
Introducing NoSQL
Big datas existence can be traced back to the mid 1990s. However, the actual shift began in the early 2000s. The evolution of the Internet and mobile technology opened many doors for more people to participate and share data globally. This resulted in massive data production, in various formats, flowing across the globe. A wider distributed network resulted in incremental data growth. Due to this massive data generation, there is a major shift in application development and many new domain business possibilities have emerged, like:
- Social trending
- OLAP and Data mining
- Sentiment analysis
- Behavior targeting
- Real-time data analysis
With high data growth into peta/zeta bytes, challenges like scalability and managing data structure would be very difficult with traditional relational databases. Here big data and NoSQL technologies are considered an alternative to building solutions. In todays scenario, existing business domains are also exploring the possibilities of new functional aspects and handling massive data growth simultaneously.
NoSQL Ecosystem
NoSQL, often called Not Only SQL, implies thinking beyond traditional SQL in a distributed way. There are more than 150 NoSQL databases available today. The following are a few popular databases:
- Columnar databases, such as Cassandra & HBase
- Document based storage like MongoDB & Couchbase
- Graph based access like Neo4J & Titan Graph DB
- Simple key-value store like Redis & Couch DB
With so many options and categories, the most important question is, what, how, and why to choose! Each NoSQL database category is meant to deal with a specific set of problems. Specific technology for specific requirement paradigm is leading the current era of technology. It is certain that a single database for all business needs is clearly not a solution, and thats where the need for NoSQL databases arises. The best way to adopt databases is to understand the requirements first. If the application is polyglot in nature, then you may need to choose more than one database from the available options. In the next section, we will discuss a few points that describe why Cassandra could be an answer to your big data problem.
CAP Theorem
CAP theorem, which was introduced in early 2000 by Eric Brewer, states that no database can offer Consistency, Availability, and Partitiontolerance ), but depending on use case may allow for any two of them.
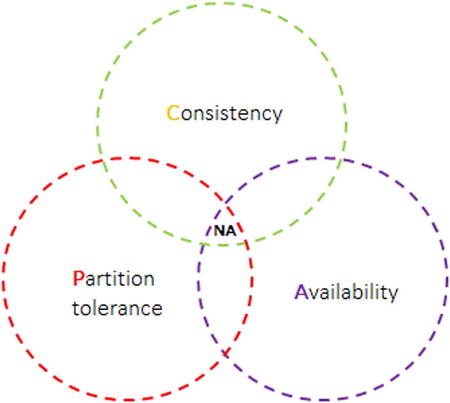
. CAP theorem excludes the possibility of a database with all three characteristics (the NA area)
Traditional relational database management systems (RDBMS) provide atomicity, consistency, isolation, and durability (ACID) semantics and advocate for strong consistency. Thats where most of NoSQL databases differ and strongly advocate for partition tolerance and high availability with eventual consistency.
- Highavailability of data means data must be available with minimal latency. For distributed databases where data is distributed across multiple nodes, one way to achieve high availability is to replicate it across multiple nodes. Like most of NoSQL databases, Cassandra also provides high availability.
- Partitiontolerance implies if a node or couple of nodes is down, the system would still be able to serve read/write requests. In scalable systems, built to deal with a massive volume of data (in peta bytes) it is highly likely that situations may occur often. Hence, such systems have to be partition tolerant. Cassandras storage architecture enables this as well.
- Consistency means consistent across distributed nodes. Strong consistency refers to most updated or consistent data on each node in a cluster. On each read/write request most stable rows can be read or written to by introducing latency (downside of NoSQL) on each read and write request, ensuring synchronized data on all the replicas. Cassandra offers eventual consistency, and levels of configuration consistency for each read/write request. We will discuss various consistency level options in detail in the coming chapters.
Budding Schema
Structured or fixed schema defines the number of columns and data types before implementation. Any alteration to schema like adding column(s) would require a migration plan across the schema. For semistructured or unstructured data formats where number of columns and data types may vary across multiple rows, static schema doesnt fit very well. Thats where budding or dynamic schema is best fit for semistructured or unstructured data.
presents four records containing twitter-like data for a particular user id. Here, the user id imvivek consists of three columns tweet body, followers, and retweeted by. But on the row for user apress_team there is only the column followers. For unstructured schema such as server logs, the number of fields may vary from row to row. This requires the addition of columns on the fly a strong requirement for NoSQL databases. Traditional RDBMS can handle such data set in a static way, but unlike Cassandra RDBMS cannot scale to have up to a million columns per row in each partition. With predefined models in the RDBMS world, handling frequent schema changes is certainly not a workable option. Imagine if we attempt to support dynamic columns we may end up having many null columns! Having default null values for multiple columns per row is certainly not desirable. With Cassandra we can have as many columns as we want (up to 2 billion)! Also another possible option is to define datatype for column names (comparator) which is not possible with RDBMS (to have a column name of type integer).
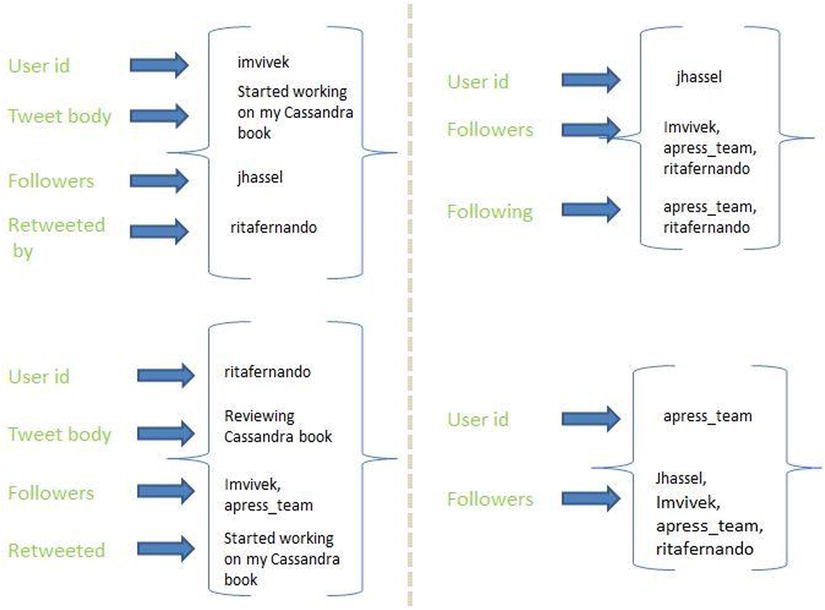
. A dynamic column, a.k.a. budding schema, is one way to relax static schema constraint of RDBMS world
Scalability
Traditional RDBMSs offer vertical scalability, that is, scaling by adding more processors or RAM to a single unit. Whereas, NoSQL databases offer horizontal scalability, and add more nodes. Mostly NoSQL databases are schemaless and can perform well over commodity servers. Adding nodes to an existing RDBMS cluster is a cumbersome process and relatively expensive whereas it is relatively easy to add data nodes with a NoSQL database, such as Cassandra. We will discuss adding nodes to Cassandra in coming chapters.
No Single Point of Failure
With centralized databases or master/slave architectures, where database resources or a master are available on a single machine, database services come to a complete halt if the master node goes down. Such database architectures are discouraged where high availability of data is a priority. NoSQL distributed databases generally prefer multiple master/slave configuration or peer-to-peer architecture to avoid a single point of failure. Cassandra delivers peer-to-peer architecture where each Cassandra node would have an identical configuration. We will discuss this at length in the coming chapters.
Next page