Don Kulasiri and Rahul Kosarwal
Chemical Master Equation for Large Biological Networks
State-space Expansion Methods Using AI
1st ed. 2021
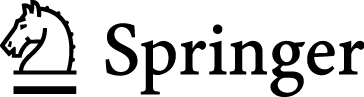
Logo of the publisher
Don Kulasiri
Centre for Advanced Computational Solutions (C-fACS), Lincoln University, Canterbury, New Zealand
Rahul Kosarwal
OAARs CORP, London, UK
Centre for Advanced Computational Solutions (C-fACS), Lincoln University, Canterbury, New Zealand
ISBN 978-981-16-5350-6 e-ISBN 978-981-16-5351-3
https://doi.org/10.1007/978-981-16-5351-3
The Editor(s) (if applicable) and The Author(s), under exclusive license to Springer Nature Singapore Pte Ltd. 2021
This work is subject to copyright. All rights are solely and exclusively licensed by the Publisher, whether the whole or part of the material is concerned, specifically the rights of translation, reprinting, reuse of illustrations, recitation, broadcasting, reproduction on microfilms or in any other physical way, and transmission or information storage and retrieval, electronic adaptation, computer software, or by similar or dissimilar methodology now known or hereafter developed.
The use of general descriptive names, registered names, trademarks, service marks, etc. in this publication does not imply, even in the absence of a specific statement, that such names are exempt from the relevant protective laws and regulations and therefore free for general use.
The publisher, the authors and the editors are safe to assume that the advice and information in this book are believed to be true and accurate at the date of publication. Neither the publisher nor the authors or the editors give a warranty, expressed or implied, with respect to the material contained herein or for any errors or omissions that may have been made. The publisher remains neutral with regard to jurisdictional claims in published maps and institutional affiliations.
This Springer imprint is published by the registered company Springer Nature Singapore Pte Ltd.
The registered company address is: 152 Beach Road, #21-01/04 Gateway East, Singapore 189721, Singapore
I dedicate this book to my late mother, Jayawathie Sinhabahu of Keselhenawa, Horana, Sri Lanka, who dedicated her life to educate me, and taught me that knowledge for its own sake was worth pursuing in a world where shallow rivers make the most noise, when the deep oceans are imbued with silence.
Don Kulasiri
I dedicate this book to my parents, Mr. O. P. Kosarwal, and Mrs. Anita Kosarwal, who have raised me to the person I am today. You have been with me every step of the way, through good times and bad. Thank you for all the unconditional love, guidance, and support that you have given me, helping me to succeed and instilling in me the confidence that I am capable of doing anything I put my mind to.
Rahul Kosarwal
Preface
Our endeavour to understand the biological phenomena at intra- and inter- cellular levels has now become the studies of biomolecular interactions conceptualized as dynamic networks. There is a large body of literature on the experimental and theoretical research in developing the networks related to genetic regulations, metabolic pathways, protein-protein interactions, membranes to name a few. We also have advancements in mathematical and computational sciences to analyze and to model these networks to understand their behaviours. One of the main research issues in these modelling efforts is the fact that molecular numbers that belong to each species in the network can greatly differ in magnitude making the molecular number landscape of a particular network heterogenous. Further, the time complexity involved in the dynamics of the networks is certainly not trivial in deciphering and conceptualizing the networks. Understanding of these irregular dynamisms of the networks is a challenge not only mathematically but also computationally. Stochastic processes provide us with a deep and sophisticated mathematics to formulate the probabilistic models of the networks and within that probabilistic conceptualization of network dynamics, the Chemical Master Equations (CMEs) bestow us with a solid foundation in understanding these complex dynamics.
In this book, we present our recent research in developing novel algorithms related to the state expansion of CMEs of large biological networks based on artificial intelligence strategies guided by Bayesian theorem. Our work here is rooted in Markovian stochastic processes and graph theory. Our aim is to reduce the time- and space complexities in algorithms when CMEs are applied to large networks. We introduce the CMEs and roles of molecular fluctuations in biological networks in Chap. deals with one of the largest networks, which has been deterministically modelled in the literature. It is seen that probabilistic interpretations of the network behaviours show very complex patterns which encapsulate the deterministic behaviours as well.
Don Kulasiri
Rahul Kosarwal
Canterbury, New Zealand
Acknowledgments
We acknowledge the Centre for Advanced Computational Solutions (C-fACS), Lincoln University, New Zealand for the facilitation of this research.
The author (DK) is also thankful to Prof. Philip Maini, Director of Wolfson Centre of Mathematical Biology, Mathematical Institute, Oxford University, United Kingdom for facilitating and supporting DK and hosting him in his numerous study-leave periods over the last 13 years.
The author (RK) expresses appreciation to OAARs CORP, United Kingdom for providing the packages and tools for this research. RK is also thankful to Mr. Anshul Kosarwal for being great source of inspiration and support throughout all my research projects.
Both of us (DK and RK) acknowledge Prof. Sandhya Samarasinghe, Lincoln University, New Zealand, and Dr. Shamayitri Ghosh, University Hospitals Sussex NHS Foundation trust, United Kingdom for their constant encouragement and support in completing this book.
Canterbury, New Zealand
London, UK
June 2021
Don Kulasiri
Rahul Kosarwal
Abbreviations
Terminology
AD
Alzheimers Disease
AI
Artificial Intelligence
Algo
Algorithm
AWS
Amazon Web Services
BLNP
Bayesian Likelihood Node Projection
CK
Chapman-Kolmogorov Equation
CME
Chemical Master Equation
CPU
Central Processing Unit
CSR
Compressed Row Format
CTHMC
Continuous Time Homogeneous Markov Chain
CTMC
Continuous Time Markov Chain
DAGs
Directed Acyclic Graphs
DDR3
Double Data Rate Version 3
EBS
Elastic Block Storage
EC2
Elastic Computing Version 2
ECUs
Electronic Controlling Units
EFS
Elastic File System
FIFO
First In, First Out
FSP
Finite State Projection
GORDE
Gated One Reaction Domain Expansion
GP2
General Purpose Version 2 (SSD)
HVM
Hardware Virtual Environment
IOPS
Input Output per second
ISP
Intelligent State Projection Method
LAS
Latitudinal Search
LIFO
Last In, First Out
LOLAS
Longitudinal Latitudinal Search
ML
Machine Learning
ODE or ODEs