Kamath Uday - Transformers for Machine Learning
Here you can read online Kamath Uday - Transformers for Machine Learning full text of the book (entire story) in english for free. Download pdf and epub, get meaning, cover and reviews about this ebook. year: 2022, publisher: CRC Press LLC, genre: Home and family. Description of the work, (preface) as well as reviews are available. Best literature library LitArk.com created for fans of good reading and offers a wide selection of genres:
Romance novel
Science fiction
Adventure
Detective
Science
History
Home and family
Prose
Art
Politics
Computer
Non-fiction
Religion
Business
Children
Humor
Choose a favorite category and find really read worthwhile books. Enjoy immersion in the world of imagination, feel the emotions of the characters or learn something new for yourself, make an fascinating discovery.
- Book:Transformers for Machine Learning
- Author:
- Publisher:CRC Press LLC
- Genre:
- Year:2022
- Rating:5 / 5
- Favourites:Add to favourites
- Your mark:
- 100
- 1
- 2
- 3
- 4
- 5
Transformers for Machine Learning: summary, description and annotation
We offer to read an annotation, description, summary or preface (depends on what the author of the book "Transformers for Machine Learning" wrote himself). If you haven't found the necessary information about the book — write in the comments, we will try to find it.
Transformers for Machine Learning — read online for free the complete book (whole text) full work
Below is the text of the book, divided by pages. System saving the place of the last page read, allows you to conveniently read the book "Transformers for Machine Learning" online for free, without having to search again every time where you left off. Put a bookmark, and you can go to the page where you finished reading at any time.
Font size:
Interval:
Bookmark:
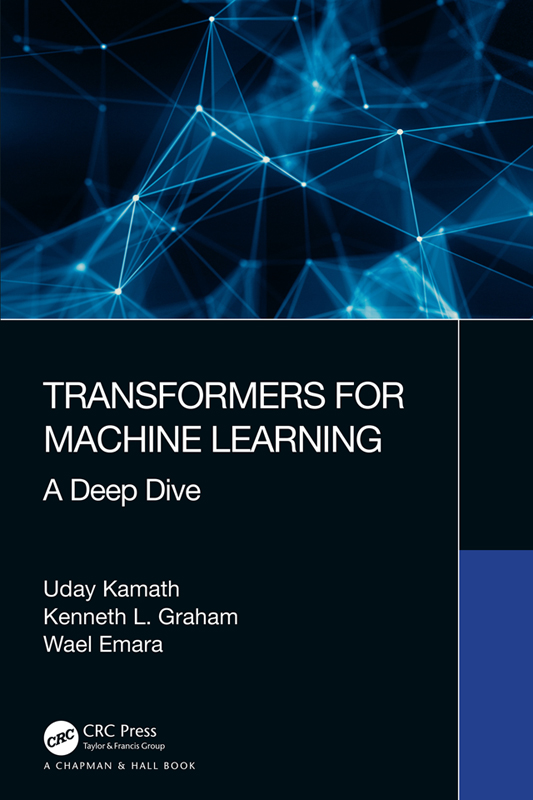
A First Course in Machine Learning
Simon Rogers, Mark Girolami
Statistical Reinforcement Learning: Modern Machine Learning Approaches
Masashi Sugiyama
Sparse Modeling: Theory, Algorithms, and Applications
Irina Rish, Genady Grabarnik
Computational Trust Models and Machine Learning
Xin Liu, Anwitaman Datta, Ee-Peng Lim
Regularization, Optimization, Kernels, and Support Vector Machines
Johan A.K. Suykens, Marco Signoretto, Andreas Argyriou
Machine Learning: An Algorithmic Perspective, Second Edition
Stephen Marsland
Bayesian Programming
Pierre Bessiere, Emmanuel Mazer, Juan Manuel Ahuactzin, Kamel Mekhnacha
Multilinear Subspace Learning: Dimensionality Reduction of Multidimensional Data
Haiping Lu, Konstantinos N. Plataniotis, Anastasios Venetsanopoulos
Data Science and Machine Learning: Mathematical and Statistical Methods
Dirk P. Kroese, Zdravko Botev, Thomas Taimre, Radislav Vaisman
Deep Learning and Linguistic Representation
Shalom Lappin
Artificial Intelligence and Causal Inference
Momiao Xiong
Introduction to Machine Learning with Applications in Information Security, Second Edition
Mark Stamp
Entropy Randomization in Machine Learning
Yuri S. Popkov, Alexey Yu. Popkov, Yuri A. Dubno
Transformers for Machine Learning: A Deep Dive
Uday Kamath, Kenneth L. Graham, and Wael Emara
For more information on this series please visit: https://www.routledge.com/Chapman--HallCRC-Machine-Learning--Pattern-Recognition/book-series/CRCMACLEAPAT
First edition published 2022
by CRC Press
6000 Broken Sound Parkway NW, Suite 300, Boca Raton, FL 33487-2742
and by CRC Press
4 Park Square, Milton Park, Abingdon, Oxon, OX14 4RN
CRC Press is an imprint of Taylor & Francis Group, LLC
2022 Uday Kamath, Kenneth L. Graham and Wael Emara
Reasonable efforts have been made to publish reliable data and information, but the author and publisher cannot assume responsibility for the validity of all materials or the consequences of their use. The authors and publishers have attempted to trace the copyright holders of all material reproduced in this publication and apologize to copyright holders if permission to publish in this form has not been obtained. If any copyright material has not been acknowledged please write and let us know so we may rectify in any future reprint.
Except as permitted under U.S. Copyright Law, no part of this book may be reprinted, reproduced, transmitted, or utilized in any form by any electronic, mechanical, or other means, now known or hereafter invented, including photocopying, microfilming, and recording, or in any information storage or retrieval system, without written permission from the publishers.
For permission to photocopy or use material electronically from this work, access
Trademark notice: Product or corporate names may be trademarks or registered trademarks and are used only for identification and explanation without intent to infringe.
Library of Congress CataloginginPublication Data
Names: Kamath, Uday, author.
Title: Transformers for machine learning : a deep dive / Uday Kamath, Kenneth L. Graham, Wael Emara.
Description: First edition. | Boca Raton : CRC Press, 2022. | Includes bibliographical references and index.
Identifiers: LCCN 2021059529 | ISBN 9780367771652 (hardback) | ISBN 9780367767341 (paperback) | ISBN 9781003170082 (ebook)
Subjects: LCSH: Neural networks (Computer science). | Computational intelligence. | Machine learning.
Classification: LCC QA76.87 .K354 2022 | DDC 006.3/2dc23/eng/20220218
LC record available at https://lccn.loc.gov/2021059529
ISBN: 978-0-367-77165-2 (hbk)
ISBN: 978-0-367-76734-1 (pbk)
ISBN: 978-1-003-17008-2 (ebk)
DOI: 10.1201/9781003170082
Typeset in Latin Modern font
by KnowledgeWorks Global Ltd.
Publisher's note: This book has been prepared from camera-ready copy provided by the authors.
To all the researchers and frontline COVID workers for their extraordinary service.
Uday Kamath, Kenneth L. Graham, and Wael Emara
To my parents Krishna and Bharathi, my wife Pratibha, the kids Aaroh and Brandy, my family and friends for their support.
Uday Kamath
To my wife Alyson, to my mother, my in-laws, my family and friends, thank you for the support and your willingness to sacrifice your time with me.
Kenneth L. Graham
To my wife Noha, my parents Ali and Zainab, my sister Wesam, my extended family and friends, thank you all for being there for me all the time.
Wael Emara
Renowned AI pioneer and Nobel laureate Herbert Simon underscored attention as the most valuable resource of the information economy, as necessary to allocate attention efficiently among the overabundance of information resources. Having written the foundational paper on meaning-aware AI and recently having served as MIT-Princeton-USAF-AFRL AI Faculty-SME, I had the privilege of publishing by invitation in the same journal's special issue of ASQ, and of being the Malcolm Baldrige National Quality Award administrator, as well as being ranked along with Dr. Simon in the same global academic citation impact studies.
Given the above background, I am thrilled to share with you the most thorough and up-to-date compendium of research, practices, case studies, and applications available today that can provide the best ROI on the latest AI technological advances on transformers inspired by the paper, Attention is All You Need. Since Google introduced transformer architecture in 2017, transformers have provided exponential improvements in context-focused realization toward meaning-aware AI as deep (neural network) learning models based upon attention mechanisms such as dot-product attention and multi-head attention. Resulting advances in enhanced parallel processing of sequential data have made efficient context sensitive and hence more meaningful for ever-larger datasets and much more feasible than earlier.
Covering the latest advances in neural network architectures related to transformers spanning applications such as Natural Language Processing (NLP), speech recognition, time series analysis, and computer vision and domain-specific models spanning science, medicine, and finance, the book aims to meet the theoretical, research, application, and practical needs across academia and industry for multiple audiences including postgraduate students and researchers, undergraduate students, industry practitioners, and professionals. The book rounds off its theory-driven applied and practical coverage with hands-on case studies with focus on AI explainability, an increasingly important theme in practice imposed by greater focus on issues such as ethical AI and trustable AI.
Dr. Yogesh Malhotra
Founding Chairman and CEO
U.S. Venture Capital and Private Equity Firm
Global Risk Management Network LLC
scientist
www.yogeshmalhotra.com
Since 2012 deep learning architectures have started to dominate the machine learning field. However, most of the breakthroughs were in computer vision applications. The main driver of that success was convolutional neural network (CNN) architecture. The efficiency and parallelization of CNN have allowed computer vision architectures to pre-train on enormous data which proved to be a key factor in their success. For years afterward natural language processing (NLP) applications did not see much impact from the new deep learning revolution. Traditional sequence modeling architectures, such as recurrent neural networks (RNNs) and long short-term memory (LSTM), have been used for NLP applications. The sequential nature of such architectures has limited the possibilities to train on the same scale of data that showed value for computer vision.
Font size:
Interval:
Bookmark:
Similar books «Transformers for Machine Learning»
Look at similar books to Transformers for Machine Learning. We have selected literature similar in name and meaning in the hope of providing readers with more options to find new, interesting, not yet read works.
Discussion, reviews of the book Transformers for Machine Learning and just readers' own opinions. Leave your comments, write what you think about the work, its meaning or the main characters. Specify what exactly you liked and what you didn't like, and why you think so.