Donald Farmer - Embedded Analytics
Here you can read online Donald Farmer - Embedded Analytics full text of the book (entire story) in english for free. Download pdf and epub, get meaning, cover and reviews about this ebook. year: 2023, publisher: OReilly Media, Inc., genre: Home and family. Description of the work, (preface) as well as reviews are available. Best literature library LitArk.com created for fans of good reading and offers a wide selection of genres:
Romance novel
Science fiction
Adventure
Detective
Science
History
Home and family
Prose
Art
Politics
Computer
Non-fiction
Religion
Business
Children
Humor
Choose a favorite category and find really read worthwhile books. Enjoy immersion in the world of imagination, feel the emotions of the characters or learn something new for yourself, make an fascinating discovery.
- Book:Embedded Analytics
- Author:
- Publisher:OReilly Media, Inc.
- Genre:
- Year:2023
- Rating:5 / 5
- Favourites:Add to favourites
- Your mark:
- 100
- 1
- 2
- 3
- 4
- 5
Embedded Analytics: summary, description and annotation
We offer to read an annotation, description, summary or preface (depends on what the author of the book "Embedded Analytics" wrote himself). If you haven't found the necessary information about the book — write in the comments, we will try to find it.
Embedded Analytics — read online for free the complete book (whole text) full work
Below is the text of the book, divided by pages. System saving the place of the last page read, allows you to conveniently read the book "Embedded Analytics" online for free, without having to search again every time where you left off. Put a bookmark, and you can go to the page where you finished reading at any time.
Font size:
Interval:
Bookmark:
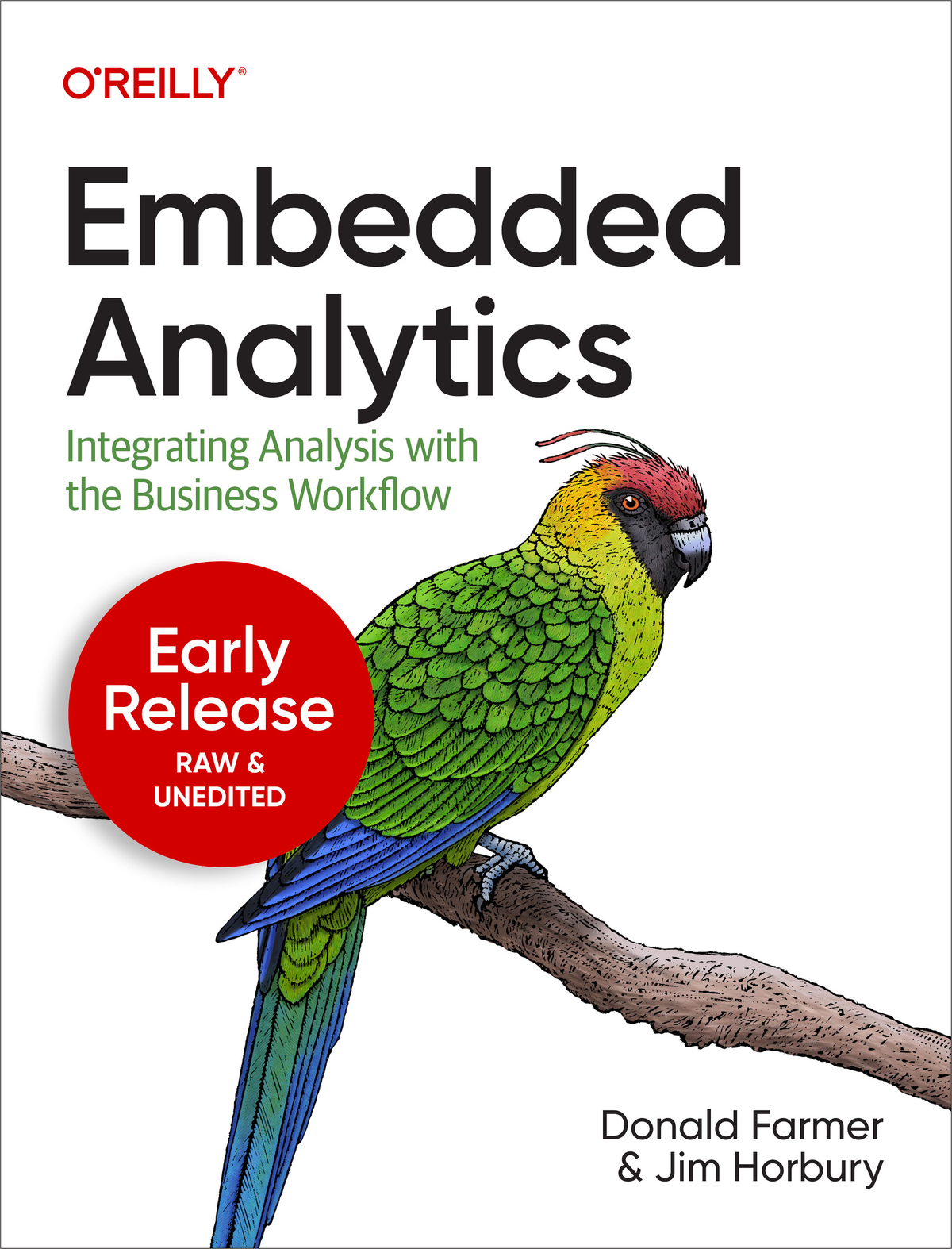
by Donald Farmer and Jim Horbury
Copyright 2023 TreeHive Strategy. All rights reserved.
Printed in the United States of America.
Published by OReilly Media, Inc. , 1005 Gravenstein Highway North, Sebastopol, CA 95472.
OReilly books may be purchased for educational, business, or sales promotional use. Online editions are also available for most titles (http://oreilly.com). For more information, contact our corporate/institutional sales department: 800-998-9938 or corporate@oreilly.com.
- Acquisitions Editor: Michelle Smith
- Development Editor: Jeff Bleiel
- Production Editor: Kate Galloway
- Interior Designer: David Futato
- Cover Designer: Karen Montgomery
- Illustrator: Kate Dullea
- June 2023: First Edition
- 2022-09-01: First Release
- 2022-10-25: First Release
See http://oreilly.com/catalog/errata.csp?isbn=9781098120931 for release details.
The OReilly logo is a registered trademark of OReilly Media, Inc. Embedded Analytics, the cover image, and related trade dress are trademarks of OReilly Media, Inc.
The views expressed in this work are those of the authors and do not represent the publishers views. While the publisher and the authors have used good faith efforts to ensure that the information and instructions contained in this work are accurate, the publisher and the authors disclaim all responsibility for errors or omissions, including without limitation responsibility for damages resulting from the use of or reliance on this work. Use of the information and instructions contained in this work is at your own risk. If any code samples or other technology this work contains or describes is subject to open source licenses or the intellectual property rights of others, it is your responsibility to ensure that your use thereof complies with such licenses and/or rights.
978-1-098-12087-0
With Early Release ebooks, you get books in their earliest formthe authors raw and unedited content as they writeso you can take advantage of these technologies long before the official release of these titles.
This will be the 1st chapter of the final book. Please note that the GitHub repo will be made active later on.
If you have comments about how we might improve the content and/or examples in this book, or if you notice missing material within this chapter, please reach out to the authors at .
Most of us are familiar today with business intelligence (BI). At one time, it was a new and exciting capability, but now, thanks to self-service technologies, the cloud and the power of in-memory processing, richly featured analytic applications, data visualisations, reports and dashboards are available to almost any business user who wants wants them.
However, each of these capabilities typically depend on separate applications. To perform business analysis, you need to open your BI suite. If you want to create a special charting, you may a look to a data visualisation application.
Embedded analytics takes a somewhat different approach. The aim of embedding is to integrate visualisations, dashboards, reports and even predictive analytics or artificial intelligence capabilities inside your everyday business applications. So if you are managing a production line, preparing a budget or reviewing HR issues you can have analytic insights ready to hand to guide you.
For a business user, this means that the analytic process takes place in the context of a business process. It also means that the data and the analytic experience becomes part of your everyday work. This makes analysis more accessible to a business user.
In this chapter, we will review some of the benefits of analytics for enterprises and well also consider what makes for a successful implementation. With that knowledge, we will also look at how we could map out a strategy to take us from our current situation to being a more fully-informed, analytics-driven enterprise.
There are several advantages to embedded analytics which business users and IT teams appreciate.
From the point of view of an IT team, who are very concerned with issues such as security and data governance, embedding your analytics in another application which is already secured has a simple but significant benefit. There is only environment and one login to be managed; the analytic application does not add an additional layer of complexity, We will look at this topic in more detail in Chapter 8 .
Business users will be most familiar with their existing operational applications. Embedding analytics in this well-understood environment affords a non-specialist user insights and information in a way that works with their existing skills and practices.
In addition to being familiar, it is also more efficient to embed analytics in a commonly used application. If we need to switch from our working application to an analytic application, we interrupt our workflow. In fact, we lose focus a little while were doing it, in addition to the simple hassle of opening and navigating to another application.
How does this work in practice?
In a call centre, an operator taking customer calls for support can see on one screen not only the customer record, but (thanks to the embedded analytics) but also trends and patterns related to this and similar cases. These patterns - perhaps the customer is calling increasingly often over time - may prompt the operator to route the call to a specialist who can better diagnose and resolve the underlying issues at hand. The result should be a better resolution for the customer and more efficient handling by the call center.
A salesperson on the road with an application that helps them select the next customer to visit and optimise the offers they make could see, embedded in that application, even on a mobile device, a recommendation driven by machine learning.
Other applications may sound more advanced, but in principle are very similar. A doctor may use an embedded algorithm to assist with diagnosis. A production-line manager in a busy factory needs may need to compare the performance of different machines in order to see where improvements can be made. An HR person, interviewing an employee with performance issues, may need to compare their work and achievements with peers within and across organizational boundaries in order to suggest a best course of action.
We can see some of these principles not only in our work but in our personal use of technology as consumers. Although we grapple with a different set of challenges, as consumers we still make decisions and take actions. As our consumption increasingly moves on line, so do our decisions and actions. In e-commerce, you expect to be given recommendations of products that may interest you, the famous example being customers who bought this also bought that. These recommendations are in fact analytics, embedded inside the web experience of e-commerce site. We dont think, when browsing for books or clothes online, that its an analytic experience. Yet analytics are driving our interactions in a way that is so seamlessly embedded, we dont even notice it.
The same is true if were watching movies online. Our watch-list likely includes recommendations made by the artificial intelligence engines of the streaming company. Again, the analytics are there, but seamlessly embedded.
Font size:
Interval:
Bookmark:
Similar books «Embedded Analytics»
Look at similar books to Embedded Analytics. We have selected literature similar in name and meaning in the hope of providing readers with more options to find new, interesting, not yet read works.
Discussion, reviews of the book Embedded Analytics and just readers' own opinions. Leave your comments, write what you think about the work, its meaning or the main characters. Specify what exactly you liked and what you didn't like, and why you think so.