Volume 972
Studies in Computational Intelligence
Series Editor
Janusz Kacprzyk
Polish Academy of Sciences, Warsaw, Poland
The series Studies in Computational Intelligence (SCI) publishes new developments and advances in the various areas of computational intelligencequickly and with a high quality. The intent is to cover the theory, applications, and design methods of computational intelligence, as embedded in the fields of engineering, computer science, physics and life sciences, as well as the methodologies behind them. The series contains monographs, lecture notes and edited volumes in computational intelligence spanning the areas of neural networks, connectionist systems, genetic algorithms, evolutionary computation, artificial intelligence, cellular automata, self-organizing systems, soft computing, fuzzy systems, and hybrid intelligent systems. Of particular value to both the contributors and the readership are the short publication timeframe and the world-wide distribution, which enable both wide and rapid dissemination of research output.
Indexed by SCOPUS, DBLP, WTI Frankfurt eG, zbMATH, SCImago.
All books published in the series are submitted for consideration in Web of Science.
More information about this series at http://www.springer.com/series/7092
Editors
Sanjay Misra and Amit Kumar Tyagi
Artificial Intelligence for Cyber Security: Methods, Issues and Possible Horizons or Opportunities
1st ed. 2021
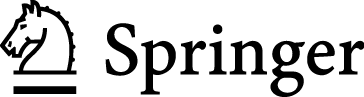
Logo of the publisher
Editors
Sanjay Misra
Center of ICT/ICE, Covenant University, Ota, Nigeria
Amit Kumar Tyagi
Vellore Institute of Technology, Chennai, Tamil Nadu, India
ISSN 1860-949X e-ISSN 1860-9503
Studies in Computational Intelligence
ISBN 978-3-030-72235-7 e-ISBN 978-3-030-72236-4
https://doi.org/10.1007/978-3-030-72236-4
The Editor(s) (if applicable) and The Author(s), under exclusive license to Springer Nature Switzerland AG 2021
This work is subject to copyright. All rights are solely and exclusively licensed by the Publisher, whether the whole or part of the material is concerned, specifically the rights of translation, reprinting, reuse of illustrations, recitation, broadcasting, reproduction on microfilms or in any other physical way, and transmission or information storage and retrieval, electronic adaptation, computer software, or by similar or dissimilar methodology now known or hereafter developed.
The use of general descriptive names, registered names, trademarks, service marks, etc. in this publication does not imply, even in the absence of a specific statement, that such names are exempt from the relevant protective laws and regulations and therefore free for general use.
The publisher, the authors and the editors are safe to assume that the advice and information in this book are believed to be true and accurate at the date of publication. Neither the publisher nor the authors or the editors give a warranty, expressed or implied, with respect to the material contained herein or for any errors or omissions that may have been made. The publisher remains neutral with regard to jurisdictional claims in published maps and institutional affiliations.
This Springer imprint is published by the registered company Springer Nature Switzerland AG
The registered company address is: Gewerbestrasse 11, 6330 Cham, Switzerland
Preface
As COVID-19 started spreading out of China in early 2020, most countries began imposing partial or total lockdown to save their citizens from this deadly virus. At the same time, organizations started implementing work from home policies for many of their employees. Work from home was acceptable from software and information technology-related companies. Still, it was not practical for all sectors, and as a result, a considerable number of professionals lost their jobs. Studies have not been done yet, but the high rate of job losers also caused high cyber-crimes. Artificial intelligence plays a crucial role in assessing, predicting, and solving problems, and its role in cyber security cannot be ignored.
It is with great pleasure that we present our book: Artificial Intelligence for Cyber security: Methods, Issues, and Possible Horizons or Opportunities. The book provides useful in-depth information regarding current research in artificial intelligence on various cyber security challenges. It comprises 18 papers from authors around the world.
In the paper , Ganesh et al. presented a system for multiple parties to learn a neural network model without disclosing their local training data to a central cloud server. The system is achieved by using distributed stochastic gradient descent combined with additively homomorphic encryption (HE). This process thereby bridges both deep learning and cryptography. A privacy-preserving deep learning framework is discussed in which all clients encrypt using a different public key in contrast to where each client encrypts using the same key.
Bindu and Thomas have presented the . In this paper, the authors have illustrated the necessity of a suitable preprocessing stage to eliminate the irrelevant and redundant features before the classifier for enhancing its performance, facilitating data visualization, and reducing storage requirements and modeling time.
In the paper , Ugot et al. trained deep convolutional generative adversarial network (DCGAN) to generate fake biometric fingerprint images. The quality of the generated images was measured using the NFIQ2 algorithm, and qualitative analysis of the fake fingerprints indicates that the images are of good quality.
In the paper , Kumar et al. have presented an assessment procedure for evaluating economic risks in virtual power plants (VPPs) using two deep learning techniques, viz. Nave Bayes algorithm and J48 bagging tree model. A comprehensive matrix of cyber security economic assessment based on threats, motives, impacts, challenges, and economic impacts is provided in this paper.
Aniche et al. have presented a Bio-EVS system in their work . Authors have adopted artificial intelligence (AI) pattern recognition and cyber security algorithms to encrypt and decrypt data. The presented system BIO-EVS was an excellent contribution as this system contains necessary features for e-voting systems and be adopted by government organizations in Sub-Saharan African countries.
Olalekan et al. have presented work on . In this paper, the authors developed a new methodology for intrusion detection by combining a wrapper feature selection approach based on a genetic algorithm with synthetic minority oversampling (SMOTE) and resample techniques to balance the class distribution.
In their paper entitled , Abirami and Palanikumar proposed a system to identify and detect malicious packets over a wide range of attacks using a machine learning classifier model. In their work, the KDD dataset is trained and evaluated for performance in terms of accuracy to provide a high-quality detection system. The proposed work uses machine learning algorithms such as support vector machine (SVM) and Nave Bayesian to train the model and validate using incoming live network packets for denial of service (DoS) type of attack.