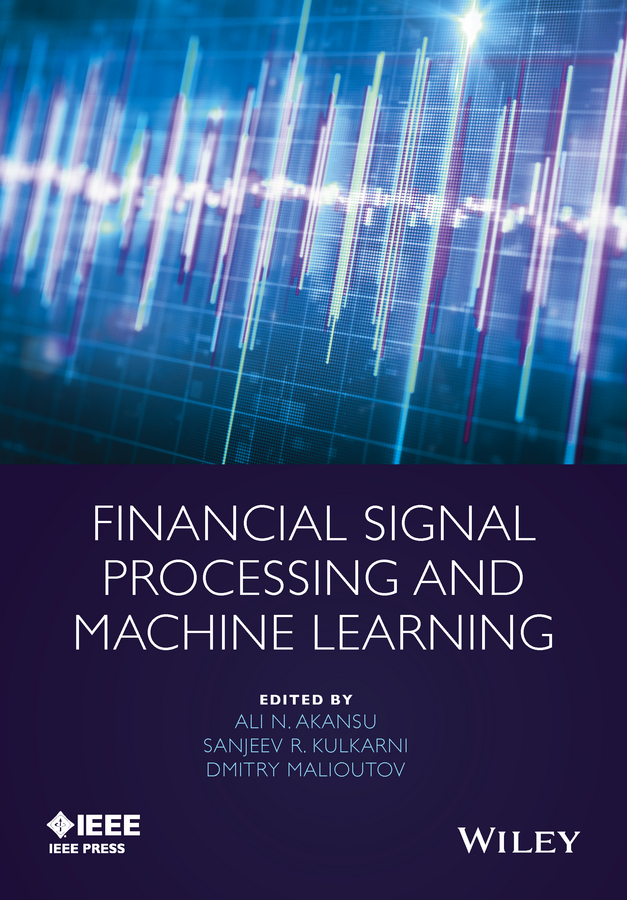
This edition first published 2016
2016 John Wiley & Sons, Ltd
First Edition published in 2016
Registered office
John Wiley & Sons Ltd, The Atrium, Southern Gate, Chichester, West Sussex, PO19 8SQ, United Kingdom
For details of our global editorial offices, for customer services and for information about how to apply for permission to reuse the copyright material in this book please see our website at www.wiley.com.
The right of the author to be identified as the author of this work has been asserted in accordance with the UK Copyright, Designs and Patents Act 1988.
All rights reserved. No part of this publication may be reproduced, stored in a retrieval system, or transmitted, in any form or by any means, electronic, mechanical, photocopying, recording or otherwise, except as permitted by the UK Copyright, Designs and Patents Act 1988, without the prior permission of the publisher.
Wiley also publishes its books in a variety of electronic formats. Some content that appears in print may not be available in electronic books.
Designations used by companies to distinguish their products are often claimed as trademarks. All brand names and product names used in this book are trade names, service marks, trademarks or registered trademarks of their respective owners. The publisher is not associated with any product or vendor mentioned in this book.
Limit of Liability/Disclaimer of Warranty: While the publisher and author(s) have used their best efforts in preparing this book, they make no representations or warranties with respect to the accuracy or completeness of the contents of this book and specifically disclaim any implied warranties of merchantability or fitness for a particular purpose. It is sold on the understanding that the publisher is not engaged in rendering professional services and neither the publisher nor the author shall be liable for damages arising herefrom. If professional advice or other expert assistance is required, the services of a competent professional should be sought.
Library of Congress Cataloging-in-Publication Data applied for
ISBN: 9781118745670
A catalogue record for this book is available from the British Library.
List of Contributors
- Ali N. Akansu New Jersey Institute of Technology, USA
- Marco Cuturi Kyoto University, Japan
- Alexandre d'Aspremont CNRS - Ecole Normale suprieure, France
- Christine De Mol Universit Libre de Bruxelles, Belgium
- Jianqing Fan Princeton University, USA
- Jun-ya Gotoh Chuo University, Japan
- Nicholas A. James Cornell University, USA
- Prabhanjan Kambadur Bloomberg L.P., USA
- Alexander Kreinin Risk Analytics, IBM, Canada
- Sanjeev R. Kulkarni Princeton University, USA
- Yuan Liao University of Maryland, USA
- Han Liu Princeton University, USA
- Matthew Lorig University of Washington, USA
- Aurlie C. Lozano IBM T.J. Watson Research Center, USA
- Ronny Luss IBM T.J. Watson Research Center, USA
- Dmitry Malioutov IBM T.J. Watson Research Center, USA
- David S. Matteson Cornell University, USA
- William B. Nicholson Cornell University, USA
- Ronnie Sircar Princeton University, USA
- Akiko Takeda The University of Tokyo, Japan
- Mustafa U. Torun New Jersey Institute of Technology, USA
- Stan Uryasev University of Florida, USA
- Onur Yilmaz New Jersey Institute of Technology, USA
Preface
This edited volume collects and unifies a number of recent advances in the signal-processing and machine-learning literature with significant applications in financial risk and portfolio management. The topics in the volume include characterizing statistical dependence and correlation in high dimensions, constructing effective and robust risk measures, and using these notions of risk in portfolio optimization and rebalancing through the lens of convex optimization. It also presents signal-processing approaches to model return, momentum, and mean reversion, including both theoretical and implementation aspects. Modern finance has become global and highly interconnected. Hence, these topics are of great importance in portfolio management and trading, where the financial industry is forced to deal with large and diverse portfolios in a variety of asset classes. The investment universe now includes tens of thousands of international equities and corporate bonds, and a wide variety of other interest rate and derivative products-often with limited, sparse, and noisy market data.
Using traditional risk measures and return forecasting (such as historical sample covariance and sample means in Markowitz theory) in high-dimensional settings is fraught with peril for portfolio optimization, as widely recognized by practitioners. Tools from high-dimensional statistics, such as factor models, eigen-analysis, and various forms of regularization that are widely used in real-time risk measurement of massive portfolios and for designing a variety of trading strategies including statistical arbitrage, are highlighted in the book. The dramatic improvements in computational power and special-purpose hardware such as field programmable gate arrays (FPGAs) and graphics processing units (GPUs) along with low-latency data communications facilitate the realization of these sophisticated financial algorithms that not long ago were hard to implement.
The book covers a number of topics that have been popular recently in machine learning and signal processing to solve problems with large portfolios. In particular, the connections between the portfolio theory and sparse learning and compressed sensing, robust optimization, non-Gaussian data-driven risk measures, graphical models, causal analysis through temporal-causal modeling, and large-scale copula-based approaches are highlighted in the book.
Although some of these techniques already have been used in finance and reported in journals and conferences of different disciplines, this book attempts to give a unified treatment from a common mathematical perspective of high-dimensional statistics and convex optimization. Traditionally, the academic quantitative finance community did not have much overlap with the signal and information-processing communities. However, the fields are seeing more interaction, and this trend is accelerating due to the paradigm in the financial sector which has embraced state-of-the-art, high-performance computing and signal-processing technologies. Thus, engineers play an important role in this financial ecosystem. The goal of this edited volume is to help to bridge the divide, and to highlight machine learning and signal processing as disciplines that may help drive innovations in quantitative finance and electronic trading, including high-frequency trading.
The reader is assumed to have graduate-level knowledge in linear algebra, probability, and statistics, and an appreciation for the key concepts in optimization. Each chapter provides a list of references for readers who would like to pursue the topic in more depth. The book, complemented with a primer in financial engineering, may serve as the main textbook for a graduate course in financial signal processing.
We would like to thank all the authors who contributed to this volume as well as all of the anonymous reviewers who provided valuable feedback on the chapters in this book. We also gratefully acknowledge the editors and staff at Wiley for their efforts in bringing this project to fruition.
Next page