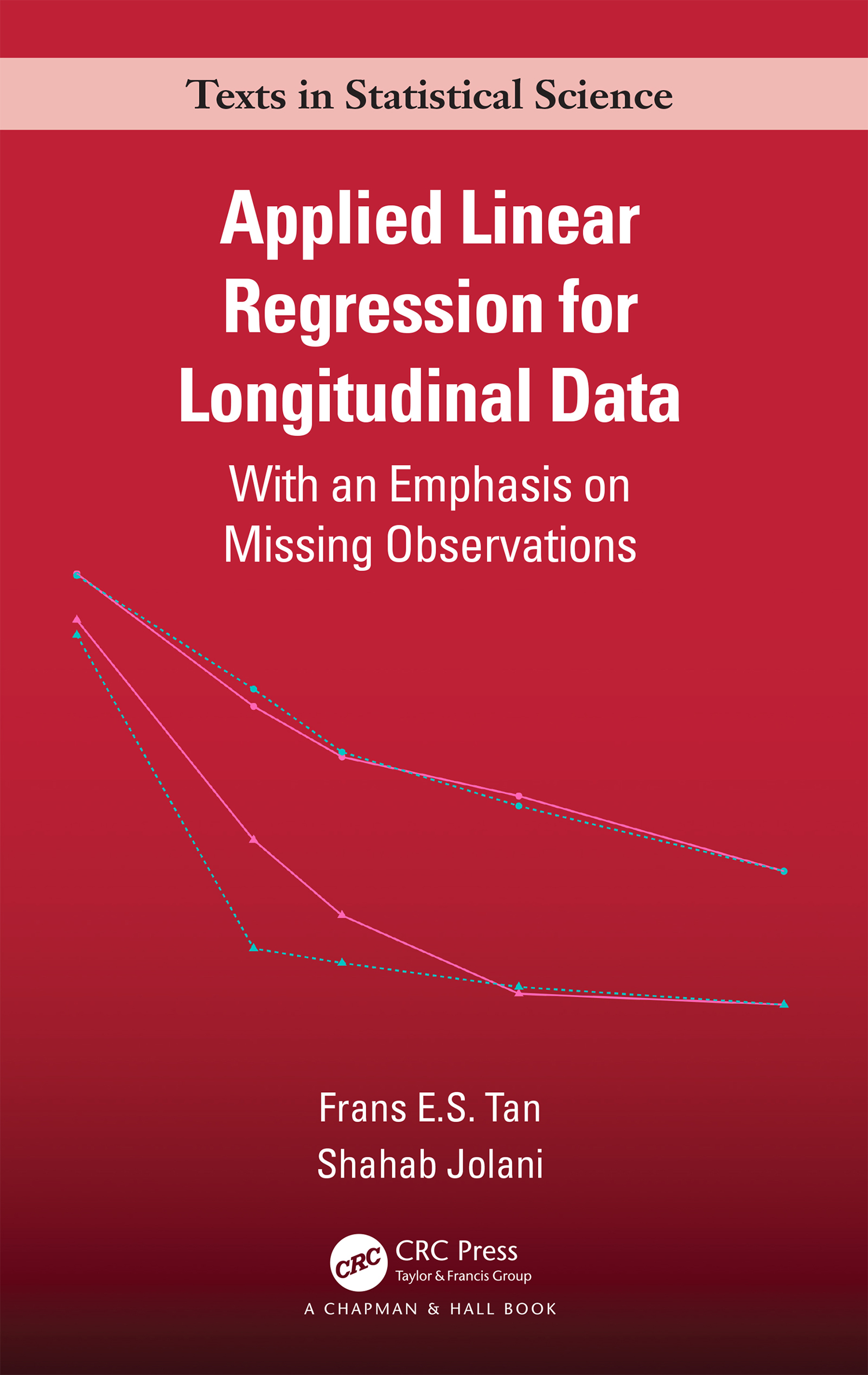
Applied Linear Regression for Longitudinal Data
This book introduces best practices in longitudinal data analysis at an intermediate level, with a minimum number of formulas without sacrificing depths. It meets the need to understand statistical concepts of longitudinal data analysis by visualising important techniques instead of using abstract mathematical formulas. Different solutions such as multiple imputation are explained conceptually, and consequences of missing observations are clarified using visualisation techniques. Key features include the following:
- Provides datasets and examples online
- Gives state-of-the-art methods of dealing with missing observations in a nontechnical way with a special focus on sensitivity analysis
- Conceptualises the analysis of comparative (experimental and observational) studies
It is the ideal companion for researchers and students in epidemiological, health, and social and behavioural sciences working with longitudinal studies without a mathematical background.
Frans E.S. Tan is an associate professor (retired) of methodology and statistics at Maastricht University, The Netherlands.
Shahab Jolani is an assistant professor of methodology and statistics at Maastricht University, The Netherlands.
Chapman & Hall/CRC
Texts in Statistical Science Series
Joseph K. Blitzstein, Harvard University, USA
Julian J. Faraway, University of Bath, UK
Martin Tanner, Northwestern University, USA
Jim Zidek, University of British Columbia, Canada
Recently Published Titles
Probability, Statistics, and Data
A Fresh Approach Using R
Darrin Speegle and Brain Claire
Bayesian Modeling and Computation in Python
Osvaldo A. Martin, Raviv Kumar and Junpeng Lao
Bayes Rules!
An Introduction to Applied Bayesian Modeling
Alicia Johnson, Miles Ott and Mine Dogucu
Stochastic Processes with R
An Introduction
Olga Korosteleva
Introduction to Design and Analysis of Scientific Studies
Nathan Taback
Practical Time Series Analysis for Data Science
Wayne A. Woodward, Bivin Philip Sadler and Stephen Robertson
Statistical Theory
A Concise Introduction, Second Edition
Felix Abramovich and Yaacov Ritov
Applied Linear Regression for Longitudinal Data
With an Emphasis on Missing Observations
Frans E.S. Tan and Shahab Jolani
For more information about this series, please visit: https://www.routledge.com/Chapman--HallCRC-Texts-in-Statistical-Science/book-series/CHTEXSTASCI
Applied Linear Regression for Longitudinal Data With an Emphasis on Missing Observations
Frans E.S. Tan
Shahab Jolani
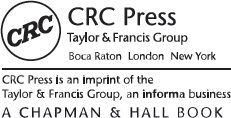
First edition published 2022
by CRC Press
6000 Broken Sound Parkway NW, Suite 300, Boca Raton, FL 33487-2742
and by CRC Press
4 Park Square, Milton Park, Abingdon, Oxon, OX14 4RN
CRC Press is an imprint of Taylor & Francis Group, LLC
2023 Taylor & Francis Group, LLC
Reasonable efforts have been made to publish reliable data and information, but the author and publisher cannot assume responsibility for the validity of all materials or the consequences of their use. The authors and publishers have attempted to trace the copyright holders of all material reproduced in this publication and apologize to copyright holders if permission to publish in this form has not been obtained. If any copyright material has not been acknowledged please write and let us know so we may rectify in any future reprint.
Except as permitted under U.S. Copyright Law, no part of this book may be reprinted, reproduced, transmitted, or utilized in any form by any electronic, mechanical, or other means, now known or hereafter invented, including photocopying, microfilming, and recording, or in any information storage or retrieval system, without written permission from the publishers.
For permission to photocopy or use material electronically from this work, access
Trademark notice: Product or corporate names may be trademarks or registered trademarks and are used only for identification and explanation without intent to infringe.
ISBN: 978-0-367-63431-5 (hbk)
ISBN: 978-0-367-63937-2 (pbk)
ISBN: 978-1-003-12138-1 (ebk)
DOI: 10.1201/9781003121381
Typeset in Palatino
by SPi Technologies India Pvt Ltd (Straive)
Access the Support Material: https://www.routledge.com/Applied-Linear-Regression-for-Longitudinal-Data-With-an-Emphasis-on-Missing/Tan-Jolani/p/book/9780367634315
To Jacqueline, Indji and Ailin
To Maryam, Ava and Tara
Preface
This book is a product of more than a decade experience in teaching multilevel analysis of longitudinal data (MALD) to PhD students and researchers in health, (bio)medical, social and behavioural sciences. Basically, the MALD course covers only linear regression methods, and extensions to nonlinear (e.g. logistic) models are not part of the course.
This book covers advanced methods for the analysis of longitudinal data, where the observations are correlated and/or subject to missingness. The standard regression method that assumes uncorrelated errors typically leads to biased estimates of regression parameters with incorrect standard errors and thus are inappropriate.
Special attention is given to the analysis of longitudinal intervention and life-event studies, where the objective is to evaluate a treatment or life-event effect. Several statistical methods to deal with missing observations are presented, depending on the type of missing data mechanism and whether the dependent variable (outcome), the independent variables (covariates) or both are partly missing.
serve as case-studies to perform a full analysis on longitudinal data in observational and experimental studies, respectively.
This book can be used as a text for a 12-week course. We recommend starting with a brief introduction to the statistical software/packages that will be used during the course. The books companion website offers a manual to perform the analyses in SPSS and R. Although the theory is explained independently of any statistical package, SPSS and R were mainly used to produce tables and figures. We also provide data files in the SPSS system-file format that may be used (not necessarily in SPSS) when working on the assignments. An introduction in SPSS (or R) can be covered in 1 week. can be covered in 1 week.
Basic knowledge of linear regression analysis and testing theory would be an advantage for a smooth understanding of the topics of this book. In fact, we have used it in courses where participants already had some experience analysing data with regression methods.
Acknowledgements
We are indebted to the Department of Methodology and Statistics at Maastricht University, The Netherlands, for facilitating an environment to write this book. We thank all colleagues and students who ever followed the MALD course and inspired us to even start this project. Their comments during and after the course were extremely useful.
Special thanks to Miranda Janssen for developing the companion website and a close collaboration in this project. We wish to thank David Grubbs and Lara Spieker, Statistics Editors at Chapman & Hall/CRC for their encouragement and patience during this project. Jessica Poile and Curtis Hill have been very helpful in facilitation of this project. Finally, several people read and commented on parts of the book. Many thanks to Martijn Berger for reviewing the manuscript and providing many helpful suggestions. We also thank Bjorn Winkens, Gerard van Breukelen, Math Candel, Jan Schepers, Sophie Vanbelle, Alberto Cassese, Francesco Innocenti, Andrea Gabrio, Gavin van der Nest and Monique Reusken for their insightful feedback. This has been very helpful to clarify the ideas.
Next page