Dan Darnell - The Future of Analytics
Here you can read online Dan Darnell - The Future of Analytics full text of the book (entire story) in english for free. Download pdf and epub, get meaning, cover and reviews about this ebook. year: 2020, publisher: OReilly Media, Inc., genre: Business. Description of the work, (preface) as well as reviews are available. Best literature library LitArk.com created for fans of good reading and offers a wide selection of genres:
Romance novel
Science fiction
Adventure
Detective
Science
History
Home and family
Prose
Art
Politics
Computer
Non-fiction
Religion
Business
Children
Humor
Choose a favorite category and find really read worthwhile books. Enjoy immersion in the world of imagination, feel the emotions of the characters or learn something new for yourself, make an fascinating discovery.
- Book:The Future of Analytics
- Author:
- Publisher:OReilly Media, Inc.
- Genre:
- Year:2020
- Rating:3 / 5
- Favourites:Add to favourites
- Your mark:
- 60
- 1
- 2
- 3
- 4
- 5
The Future of Analytics: summary, description and annotation
We offer to read an annotation, description, summary or preface (depends on what the author of the book "The Future of Analytics" wrote himself). If you haven't found the necessary information about the book — write in the comments, we will try to find it.
The Future of Analytics — read online for free the complete book (whole text) full work
Below is the text of the book, divided by pages. System saving the place of the last page read, allows you to conveniently read the book "The Future of Analytics" online for free, without having to search again every time where you left off. Put a bookmark, and you can go to the page where you finished reading at any time.
Font size:
Interval:
Bookmark:
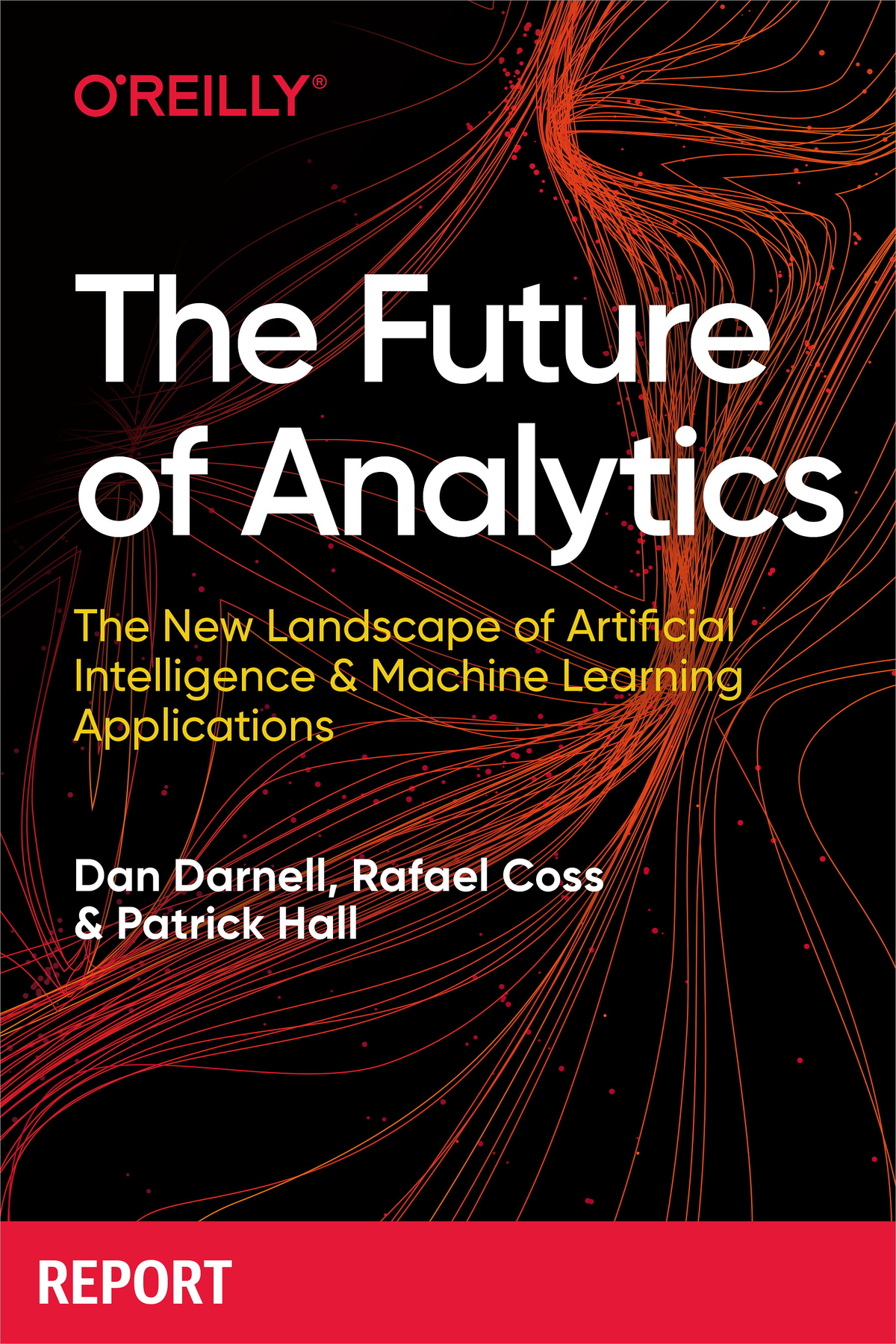
by Dan Darnell , Rafael Coss , and Patrick Hall
Copyright 2021 OReilly Media, Inc. All rights reserved.
Printed in the United States of America.
Published by OReilly Media, Inc. , 1005 Gravenstein Highway North, Sebastopol, CA 95472.
OReilly books may be purchased for educational, business, or sales promotional use. Online editions are also available for most titles (http://oreilly.com). For more information, contact our corporate/institutional sales department: 800-998-9938 or corporate@oreilly.com .
- Acquisitions Editor: Rebecca Novack
- Development Editor: Angela Rufino
- Production Editor: Katherine Tozer
- Copyeditor: nSight, Inc.
- Proofreader: Abby Wheeler
- Interior Designer: David Futato
- Cover Designer: Randy Comer
- Illustrator: Kate Dullea
- October 2020: First Edition
- 2020-10-16: First Release
The OReilly logo is a registered trademark of OReilly Media, Inc. The Future of Analytics, the cover image, and related trade dress are trademarks of OReilly Media, Inc.
The views expressed in this work are those of the authors, and do not represent the publishers views. While the publisher and the authors have used good faith efforts to ensure that the information and instructions contained in this work are accurate, the publisher and the authors disclaim all responsibility for errors or omissions, including without limitation responsibility for damages resulting from the use of or reliance on this work. Use of the information and instructions contained in this work is at your own risk. If any code samples or other technology this work contains or describes is subject to open source licenses or the intellectual property rights of others, it is your responsibility to ensure that your use thereof complies with such licenses and/or rights.
This work is part of a collaboration between OReilly and H2O.ai. See our statement of editorial independence.
978-1-492-09175-2
[LSI]
In 2015, when I started writing The Evolution of Analytics with my colleagues Wen Phan and Katie Whitson, we made the case for machine learning in business. Five years later, its time to make the case to use machine learning the right way in business. While I certainly dont know all the answers, a few themes stand out to me, looking back over the past five years. On the negative side, theres the endless hype about artificial intelligence (AI) and the tendency to deploy it in creepy and discriminatory ways. On the positive side, I see the growing government and public awareness of AI. I hope this awareness translates into the regulation of AI, improved interaction design in AI apps, and more corporate responsibility and governance for AI.
In this report, well introduce AI-driven applications that boost traditional data analytics with machine learning. While these apps may beat the odds, provide useful insights, and drive organizational value, such success stories dont serve as guarantees. In fact, everyone involved in organizational AI projects would be wise to take an inventory of AIs impacts on businesses, consumers, and the general public.
Another bright spot in AI over the last five years has been the development of technologies that increase human trust and understanding in machine learning. These inventions have transformed machine learning from a field of black-box algorithms to a field that is now capable of fierce debate around the concepts of algorithmic transparency, accountability, and fairness. This technological progress not only enables the nuts and bolts of regulatory oversight, but it also gives companies the power to govern their AI systems like the enterprise software assets they are. If you can block out the hype, youll see that AI is really just software. And like all other enterprise IT resources, AI systems should be documented, managed, monitored, and governed.
Looking forward, I see successful AI deployments being aware of the risks of AI, taking on the associated governance burdens, and enabling humans to work together with computers to solve big problems. For businesses climbing to the next plateau in digital transformation, dont settle for any AI system. Youll need AI systems that are documented, transparent, managed, monitored, and minimally discriminatory. Moreover, these AI systems must support AI apps that are flexible, explainable, and, when appropriate, automatic. Thats why Dan, Rafael, and I have written this new report, The Future of Analytics. Its a necessary update to the original Evolution of Analytics report, and we hope you find it to be a timely and useful guide through the new world of AI-powered analytics apps.
Patrick Hall
.
In the broadest sense, analytics is the systematic analysis of data. This analysis makes the data consumable by people and systems, with the goal of understanding past outcomes and helping to predict future events. The adoption of analytics has driven a wave of digital transformation across industries where companies use data to power decision-making processes. Analytics projects, however, have not been without their drawbacks.
Like many changes in business thinking, the first forays into data-driven decisions led down accessible but less useful paths. One such path was using dashboards to view historical trends to drive human insights from data, as shown in . We now know that these traditional analytics dashboards alone can be insufficient to make better decisions as they provide only a historical summary.
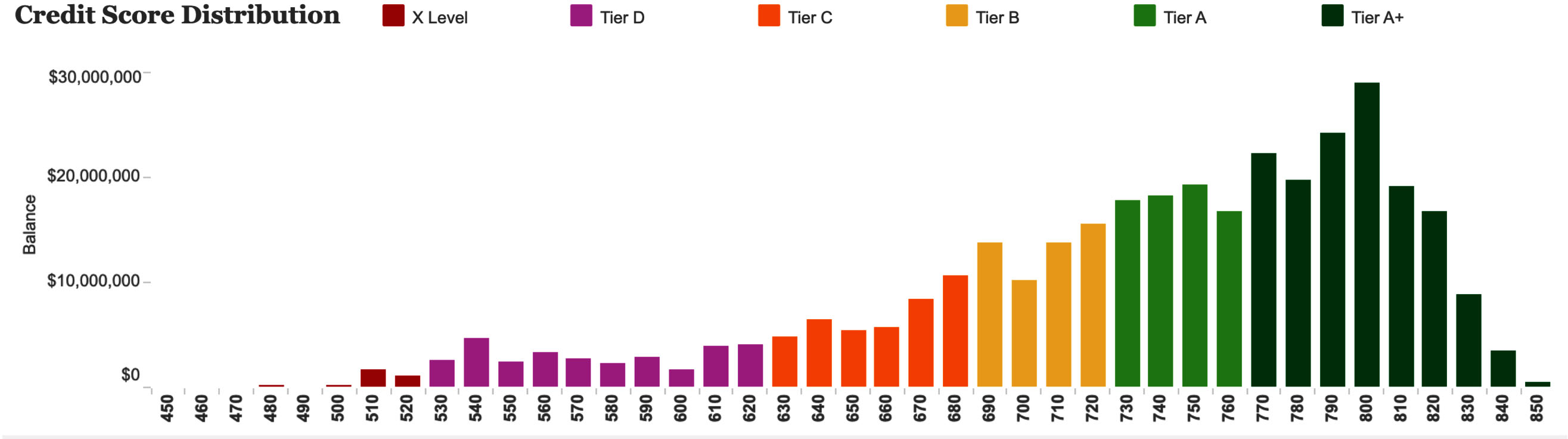
While historical trends are useful and can be predictive, they can also provide a false sense of confidence, when the future does not mirror the past. In the end, historical information and descriptive analytics alone leave business leaders to use their best judgment about trends in order to make decisions based on their own experience and limited view of the data. The result of this process is then highly dependent on the individual decision-makers expertise, which yields highly variable outputs.
Using machines to find patterns in data and make predictions is another area of great promise for decision support. Machine learning models, trained on historical data, can look at new data and predict what is likely to happen. For example, credit card companies use machine learning models to determine who has access to credit and is likely to carry a balance on their credit card bill each month. Such models can even prescribe actions for users to take and recommend products or content of interest. This ability to predict future outcomes and prescribe actions has made machine learning a hot technologyand data science a hot profession.
For all its promise, machine learning has not reached widespread usage in production or within business applications where it can provide value and support business decisions. The challenges vary by organization and use case. However, the common themes in AI and machine learning adoption revolve around a few key areas, including a lack of resources, lack of business trust in models and their outputs, difficulty putting models into production and keeping them running, lack of consistent business involvement, and bottlenecks in putting predictive results into business applications. Lets discuss some of these dilemmas below:
Font size:
Interval:
Bookmark:
Similar books «The Future of Analytics»
Look at similar books to The Future of Analytics. We have selected literature similar in name and meaning in the hope of providing readers with more options to find new, interesting, not yet read works.
Discussion, reviews of the book The Future of Analytics and just readers' own opinions. Leave your comments, write what you think about the work, its meaning or the main characters. Specify what exactly you liked and what you didn't like, and why you think so.