Stephan Raaijmakers - Deep Learning for Natural Language Processing MEAP V04
Here you can read online Stephan Raaijmakers - Deep Learning for Natural Language Processing MEAP V04 full text of the book (entire story) in english for free. Download pdf and epub, get meaning, cover and reviews about this ebook. year: 2020, publisher: Manning Publications Co., genre: Children. Description of the work, (preface) as well as reviews are available. Best literature library LitArk.com created for fans of good reading and offers a wide selection of genres:
Romance novel
Science fiction
Adventure
Detective
Science
History
Home and family
Prose
Art
Politics
Computer
Non-fiction
Religion
Business
Children
Humor
Choose a favorite category and find really read worthwhile books. Enjoy immersion in the world of imagination, feel the emotions of the characters or learn something new for yourself, make an fascinating discovery.
- Book:Deep Learning for Natural Language Processing MEAP V04
- Author:
- Publisher:Manning Publications Co.
- Genre:
- Year:2020
- Rating:3 / 5
- Favourites:Add to favourites
- Your mark:
- 60
- 1
- 2
- 3
- 4
- 5
Deep Learning for Natural Language Processing MEAP V04: summary, description and annotation
We offer to read an annotation, description, summary or preface (depends on what the author of the book "Deep Learning for Natural Language Processing MEAP V04" wrote himself). If you haven't found the necessary information about the book — write in the comments, we will try to find it.
Deep Learning for Natural Language Processing MEAP V04 — read online for free the complete book (whole text) full work
Below is the text of the book, divided by pages. System saving the place of the last page read, allows you to conveniently read the book "Deep Learning for Natural Language Processing MEAP V04" online for free, without having to search again every time where you left off. Put a bookmark, and you can go to the page where you finished reading at any time.
Font size:
Interval:
Bookmark:
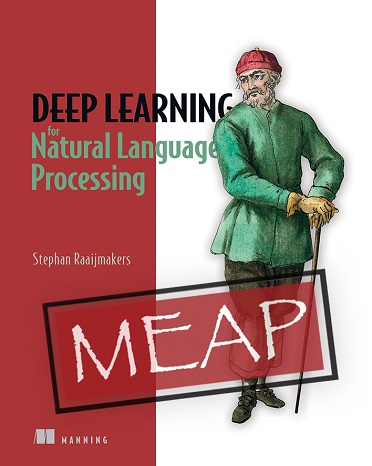

You can download the most up-to-date version of your electronic books from your Manning Account at .
Manning Publications Co. We welcome reader comments about anything in the manuscript - other than typos and other simple mistakes. These will be cleaned up during production of the book by copyeditors and proofreaders.
https://forums.manning.com/forums/deep-learning-for-natural-language-processing
Thank you for purchasing the MEAP for Deep Learning for Natural Language Processing. This book will teach you to apply deep learning to one of most vibrant applications of current AI: the analysis of natural language. The book is addressed to anyone who needs a practical guide to current deep learning techniques, from developers to students and researchers of computational linguistics.
While rooted in linguistics, the field of computational linguistics, or Natural Language Processing (NLP, for short), borrows many tools and techniques from neighboring fields such as computer science, logic, statistics and machine learning. Much of the current research in NLP now uses deep learning. It is quite hard to keep track of the many papers and software repositories that are being produced in this fast-paced field. You may even feel youre missing out on the cutting edge stuff. This is exactly what this book attempts to remedy: it will open up the toolbox of deep learning for NLP practitioners, outlining the pros and cons of various actual techniques and architectures. It speaks the language of NLP and is actually written by a computational linguist.
The book is written to be self-contained, but cannot cover all details of deep learning and NLP. Two excellent companion books, which can either be used as reference or primer, would be Mannings Deep Learning with Python, and Natural Language Processing in Action. All examples in this book are written in Python and the elegant Keras library. This is a code-intensive book, with lots of examples of deep learning solutions for well-known NLP problems. The book contains references to recent literature and some of our code draws inspiration from great public domain software repositories, including the source code examples in the Keras distribution.
Deep learning is under continuous development and may seem like a volatile moving target. I hope this practical and hands-on book will help you become proficient in the current types of deep learning, and will provide you with a solid basis for keeping up with new developments.
Writing an ambitious book like this one is a daunting task, and is prone to imperfection. Your feedback is more than welcome. If you have any questions, comments, or suggestions, please share them in Mannings Author Online forum for my book.
Stephan Raaijmakers

Part 1Introduction
Part 2Deep NLP
Part 3Advanced topics
Attention
Multitask learning
Appendixes:
Matrix algebra
Hyperparameter estimation and classifier performance evaluation
Language comes naturally to humans, but is traditionally hard to grasp for computers. This book addresses the application of recent and cutting-edge deep learning techniques to automated language analysis. Deep learning has emerged in the last decade as the vehicle of the latest wave in AI. Results have consistently redefined the state-of-the-art for a plethora of data analysis tasks in a variety of domains. For an increasing amount of deep learning algorithms, better-than-human (human-parity or superhuman) performance has been reported: for instance, speech recognition in noisy conditions, and medical diagnosis based on images. Current deep learning-based natural language processing (NLP) outperforms all pre-existing approaches with a large margin. What exactly makes deep learning so adequate for these intricate analysis tasks, in particular language processing? This chapter presents some of the background necessary for answering this question. We will guide you through a selection of important topics in machine learning for NLP.
The intended audience for this book consists of anyone working in natural language processing: computational linguists, software engineers, and machine learning or NLP students. The field of natural language processing is vast, and comprises a daunting amount of formalisms and approaches. With deep learning entering the stage, many are eager to get their feet wet, but are shied away by the highly technical nature of deep learning, and the fast pace of this field, with new approaches, software and papers emerging on a daily basis.
What you will get from this book is a thorough introduction to deep learning applied to a variety of language analysis tasks, supported by actual hands-on code. Explicitly linking the evergreens of computational linguistics, such as part-of-speech tagging, or query-answering, to deep learning, will help you become a proficient deep learning NLP expert. Beyond this, the book covers state of the art approaches to challenging new problems, such as explainable AI and the role of semantics.
This first chapter covers
A short roadtrip through machine learning applied to NLP.
A brief historical overview of deep learning.
An introduction to vector-based representations of language.
Figure 1.1. Chapter organization.
You will find a succinct overview of NLP in Appendix 1. Lets start off with discussing a few well-known machine learning-based NLP algorithms in some detail, illustrated with a handful of practical examples to wet your appetite. After that, we motivate the case for deep learning-based NLP.
Figure 1.2. Machine learning for NLP.
Current natural language processing heavily relies on machine learning. Machine learning has its roots in statistics, building among others on the seminal work by Thomas Bayes and LaPlace in the 18-th and 19-th century ( Bayes , LaPlace ) and the least squares methods for curve approximation by Legendre in 1812 ( legendre ). The field of neural computing started with the work of McCulloch and Pitts in 1943, who put forward a formal theory (and logical calculus) of neural networks ( mccullochpits43 ). It would take until 1950 before learning machines were proposed by Alan Turing ( Turing .
All machine learning algorithms that perform classification (labeling) share a single goal: to arrive at linear separability of data that is labeled with classes
Font size:
Interval:
Bookmark:
Similar books «Deep Learning for Natural Language Processing MEAP V04»
Look at similar books to Deep Learning for Natural Language Processing MEAP V04. We have selected literature similar in name and meaning in the hope of providing readers with more options to find new, interesting, not yet read works.
Discussion, reviews of the book Deep Learning for Natural Language Processing MEAP V04 and just readers' own opinions. Leave your comments, write what you think about the work, its meaning or the main characters. Specify what exactly you liked and what you didn't like, and why you think so.