Contents
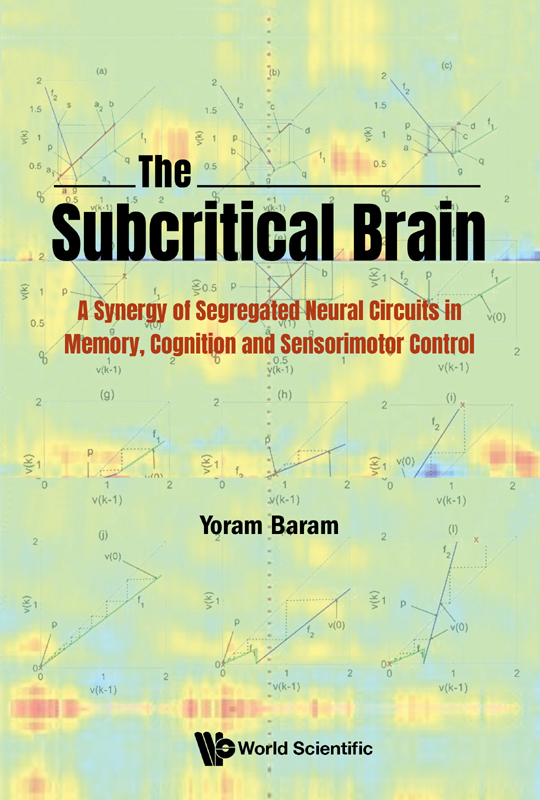
Chapter 1
Introduction
1.1General
Traditionally, the experimental nature of biological research has required a high degree of phenomenological specificity. Consequently, seemingly separate issues such as cortical development, cortical functions, inter-neuron connectivity, neuronal firing dynamics, learning and memory have been studied in seemingly complete mutual isolation. Yet, recent years have seen a trend towards integrative neuroscience, driven by a realization that, on the one hand, practically every cortical function combines a variety of molecular, biological and physical processes, and, on the other, different cortical functions are often executed by similar mechanisms. It is also becoming increasingly clear that a complete understanding of such integration would involve a new look at the cortical arena, incorporating advanced theoretical reasoning and new methods of analysis. The relatively recent emergence of numerous publications presenting advanced mathematical analysis of neurobiological structures and processes is strong evidence of this effect. Recent interdisciplinary interest in general common notions such as information, linguistics, learning, memory, computation, cognition, dynamics and control is further evidence of the same effect. It is the purpose of this book to address these issues in an analytically rigorous manner, combining relevant experimental findings and advanced theoretical concepts. While the general methodology and many of its consequential outcomes are original, they are supported by several recent publications by the author along with a wide range of references to publications by others, old and recent, related to the issues under consideration.
1.2Historical account
1.2.1The nature of cortical information
The nature of cortically embedded information has been intensely studied for over a century. Yet, it remains one of the most highly evasive mysteries of neuroscience. Following Cajals introduction of the neuron in the late 19th century (e.g., Cajal, 1890), early investigations of cortically embedded information have concerned the nature of neuronal firing (Lapicque, 1907). Elaborate chemical, physical and mathematical analysis has led to the celebrated conductance-based model of action potential (Hodgkin and Huxley, 1952). The dynamic nature of empirically observed trains of neuronal firing impulses (Adrian and Zotterman, 1926) has remained, however, more enigmatic. The very need for variability in the neuronal firing dynamics has been questioned (Stein et al., 2005; Faisal et al., 2008). While empirically observed firing sequences have been described as tonic (Murthy and Fetz, 1996; Bennett et al., 2000), oscillatory (Murthy & Fetz, 1996; Elson et al., 2002; Cymbalyuk and Shilnikov 2005; Wang, 2010), periodic (So et al., 1998), quasi-periodic (Lankheet et al., 2012), multiplexed (Panzeri et al., 2009), or silent (Melnick, 1994; Epsztein et al., 2011), some have been characterized as random (Gerstein and Mandelbrot, 1964), or as chaotic, representing functional bursting (Hayashi and Ishizuka, 1992), or deficient states of neural information processing (Fell et al., 1993). Individual neurons of the same type are often capable of producing different firing modes, switching from one to another in a seemingly unpredictable manner (Hyland et al., 2002). However, the mathematical rules underlying such behavior, the purpose it might serve, or the harm it might cause, have not been well understood. The transition from one dynamic mode to another has been called global bifurcation when caused by the landscape of the underlying map subject to fixed parameter values, and local bifurcation when caused by a change in parameter values (Blanchard et al., 2006). Bifurcations with chaos (Ren et al., 1997) and bifurcations without chaos (Li et al., 2004) have been reported. Biologically-based, analytically derived bifurcation models have been shown to produce spiking and bursting sequences (Izhikevich, 2000, Kuznetsov et al., 2006). Periodic bifurcations may in themselves represent a dynamic mode, as in the case of periodic bursting (Elson et al. 2002) instigated by postinhibitory rebound (postinhibitory facilitation, Perkel and Mulloney 1974). The choice between the spiking paradigm, putting the spotlight on the changing time intervals between neuronal spiking, and the firing-rate paradigm, seeking the information in the dynamics of interval-averaged number of spikes has been highly debated (Gerstner and Kistler, 2002). The firing-rate paradigm, while lacking in detail with respect to the spiking paradigm, has been found to offer a certain mathematical convenience, and, at the same time, reliably reproduce empirically observed firing sequences (Wilson and Cowan, 1972; Gerstner, 1995; Dayan and Abbott, 2001; Jolivet et al., 2004). Following a mathematical analysis of discrete iteration maps for neural network firing-rate, a global attractor code, relating the modes of firing-rate dynamics to internal neuron properties, has been derived (Baram, 2012, 2013a).
1.2.2Developmental aspects
Neurophysiological and molecular studies have distinguished between precritical excitability, instrumental in initial circuit formation (Hsia et al., 1998; Hensch et al., 1998; Hensch, 2005; Ashby and Isaac, 2011) and persistent plasticity, evidenced during critical development of ocular dominance in early life (Hensch, 2005; Miyata et al., 2012). Empirical characterizations of developmental stages have employed sensory responses. High sensitivity to sensory experience is crucial for early neural circuit formation (Hooks and Chen, 2007). Excitatory plasticity in precritical period immediately following birth has been found to govern activity independent synapse and dendrite generation and arborization, and axon growth, branching and targeting (Hsia et al., 1998; Ashby and Isaac, 2011; Tessier and Broadie, 2009; Gibson and Ma, 2011; Weiner et al., 2013). At the same time, the Hebbian paradigm supports activity-dependent inter-neural connectivity (Caporale and Yang, 2008; Buzsaki, 2010; Doll and Broadie, 2014). Molecular mechanisms for cortical plasticity control have been suggested, with both excitatory and inhibitory synapses playing critical roles (Constantine-Paton et al., 1990; Huang et al., 1999; Fagiolini and Hensch, 2000; Goold and Nicoll, 2010; Phillips et al., 2011; Miyata et al., 2012; Wang et al., 2012). It is largely believed that initial excitability enhances sensitivity to inhibitory effects, holding the key to plasticity modification (Huang et al., 1999; Fagiolini and Hensch, 2000; Feller and Scanziani, 2005; Goold and Nicoll, 2010; Wang et al., 2012). Beyond activity-independent neural circuit formation in early development (Hsia et al., 1998; Gibson and Ma, 2011; Weiner et al., 2013), activity-dependent circuit formation (Katz and Shatz, 1996; Gage, 2002) and later modulation (Tessier and Broadie, 2009), neural circuit modification by activation and silencing of neuronal membrane and individual synapses has been observed in early development (Melnick, 1994; Atwood and Wojtowicz, 1999; Liao et al., 1999; Losi et al., 2002; Kerchner and Nicoll, 2008), maturation (Ashby and Isaac, 2011) and later life (McGahon et al., 1999). The distinction between developmental stages has not been matched by the mathematical representation of cortical plasticity on the one hand, and of neural firing, on the other. Yet, a generalized developmental approach to biologically realistic mathematical modeling and prediction of neural connectivity has been proposed, relating neural network growth to activity and function (Borisyuk