Volume 979
Studies in Computational Intelligence
Series Editor
Janusz Kacprzyk
Polish Academy of Sciences, Warsaw, Poland
The series Studies in Computational Intelligence (SCI) publishes new developments and advances in the various areas of computational intelligencequickly and with a high quality. The intent is to cover the theory, applications, and design methods of computational intelligence, as embedded in the fields of engineering, computer science, physics and life sciences, as well as the methodologies behind them. The series contains monographs, lecture notes and edited volumes in computational intelligence spanning the areas of neural networks, connectionist systems, genetic algorithms, evolutionary computation, artificial intelligence, cellular automata, self-organizing systems, soft computing, fuzzy systems, and hybrid intelligent systems. Of particular value to both the contributors and the readership are the short publication timeframe and the world-wide distribution, which enable both wide and rapid dissemination of research output.
Indexed by SCOPUS, DBLP, WTI Frankfurt eG, zbMATH, SCImago.
All books published in the series are submitted for consideration in Web of Science.
More information about this series at http://www.springer.com/series/7092
Jaromr Vrbka
Using Artificial Neural Networks for Timeseries Smoothing and Forecasting
Case Studies in Economics
1st ed. 2021
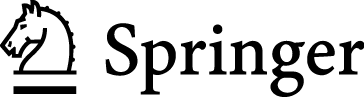
Logo of the publisher
Jaromr Vrbka
Institute of Technology and Business in esk Budjovice, esk Budjovice, Czech Republic
ISSN 1860-949X e-ISSN 1860-9503
Studies in Computational Intelligence
ISBN 978-3-030-75648-2 e-ISBN 978-3-030-75649-9
https://doi.org/10.1007/978-3-030-75649-9
The Editor(s) (if applicable) and The Author(s), under exclusive license to Springer Nature Switzerland AG 2021
This work is subject to copyright. All rights are solely and exclusively licensed by the Publisher, whether the whole or part of the material is concerned, specifically the rights of translation, reprinting, reuse of illustrations, recitation, broadcasting, reproduction on microfilms or in any other physical way, and transmission or information storage and retrieval, electronic adaptation, computer software, or by similar or dissimilar methodology now known or hereafter developed.
The use of general descriptive names, registered names, trademarks, service marks, etc. in this publication does not imply, even in the absence of a specific statement, that such names are exempt from the relevant protective laws and regulations and therefore free for general use.
The publisher, the authors and the editors are safe to assume that the advice and information in this book are believed to be true and accurate at the date of publication. Neither the publisher nor the authors or the editors give a warranty, expressed or implied, with respect to the material contained herein or for any errors or omissions that may have been made. The publisher remains neutral with regard to jurisdictional claims in published maps and institutional affiliations.
This Springer imprint is published by the registered company Springer Nature Switzerland AG
The registered company address is: Gewerbestrasse 11, 6330 Cham, Switzerland
Introduction
The majority of collected data show a certain time structure. In some cases, this structure is hidden; however, there are methods to extract relevant information from the available data using this time structure. The knowledge of time series modelling is a basic skill in the field of data science, since there as specific structures for this data type which can be examined in all situations. Time series enables the analysis of the main patterns, such as trends, seasonality, cyclicality, or data irregularities. Time series analysis is applied, e.g. for analysis of stock markets, recognition of patterns, earthquake prediction, economic predictions, census, etc. Time series forecasts use historical empirical regularities for creating future projections, which are guided by theoretical understanding of economic processes. Economic forecasts are used for a wide range of activities, including setting monetary and fiscal policy, state and local budget, financial management and financial engineering. The key elements of economic forecasts include selecting the model(s) of predictions suitable for a given problem, assessment and communicating the uncertainty related to forecasts and protection against the model instability. For example, in the case of investments, time series follows the movement of selected data points, such as security price, for a specified period of time, where the data points are recorded at regular intervals. There is no minimum or maximum period, which enables the collection of the data in a way that provides the information required by an investor or analyst that analyses the activity. Time series analysis can be useful to determine how a given asset, security or economic variable changes over time. The method of predicting time series is the most reliable in the case that the data represent a longer period of time. The information about the conditions can be obtained by measuring the data at various time intervalshourly, daily, monthly, quarterly, yearly, or at any other time interval. Predictions are most accurate if they are based on a large number of observations for a longer period of time so that it is possible to measure the patterns in given conditions. The main advantages of time series analysis include the fact that there are certain functions that cannot be captured by means of normal regression models, while the methods connected with time series can. Furthermore, there can be mentioned certain dependencies that cannot be captured by means of conventional models, especially factors such as seasonality, where the effects of trends cannot be fully explained by means of models such as linear regression, but by means of time series models. This shows that time series is useful if we try to capture aspects that usually change over time. For example, data on prices at the end of the day are very difficult to model if only regression models are used; however, time series uses a wide range of models which were proved to capture such complex phenomena as the dependence between random variables, also known as serial correlation. The most important advantage of time series consists in its ability to predict the future value of data. There are various methods to obtain prediction. There can be used, e.g. regression models, models based on the decomposition of time series, models of so-called smoothing by means of function or models based on neural networks (machine learning).
Before examining the methods of machine learning for time series, it is recommended to make sure that conventional methods of predicting linear time series cannot be used. Such models can be focussed on linear relationships; however, they are sophisticated and work well in a wide range of problems, provided that the data are prepared properly and the method is well-configured. From the conventional methods, there should be mentioned, e.g. autoregressive model (AR), moving average (MA), autoregressive moving average (ARMA), ARIMA, seasonal autoregressive integrated moving average (SARIMA), seasonal autoregressive integrated moving average with exogenous regressors (SARIMAX), vector autoregression (VAR), vector autoregressive moving average (VARMA), vector autoregressive moving average with exogenous regressors (VARMAX), simple exponential smoothing (SES), or holt winter exponential smoothing (HWES). Time series predictions have been dominated by linear methods for decades. Linear methods show an easy development and implementation and are relatively easy to understand and interpret. However, it is important to understand the limitations of linear models; they are not able to capture nonlinear relationships in data. Nevertheless, neural networks have recently appeared to be an alternative tool for predicting. The naturally nonlinear structure of neural networks is particularly useful to capture a complex basic relationship in many real-world problems. Neural networks are perhaps more versatile methods for predicting, as they are able to find nonlinear structures in a problem but can also model linear processes.