Brett Koonce - Image Recognition and Dataset Categorization
Here you can read online Brett Koonce - Image Recognition and Dataset Categorization full text of the book (entire story) in english for free. Download pdf and epub, get meaning, cover and reviews about this ebook. year: 2021, publisher: Apress, genre: Home and family. Description of the work, (preface) as well as reviews are available. Best literature library LitArk.com created for fans of good reading and offers a wide selection of genres:
Romance novel
Science fiction
Adventure
Detective
Science
History
Home and family
Prose
Art
Politics
Computer
Non-fiction
Religion
Business
Children
Humor
Choose a favorite category and find really read worthwhile books. Enjoy immersion in the world of imagination, feel the emotions of the characters or learn something new for yourself, make an fascinating discovery.
- Book:Image Recognition and Dataset Categorization
- Author:
- Publisher:Apress
- Genre:
- Year:2021
- Rating:3 / 5
- Favourites:Add to favourites
- Your mark:
- 60
- 1
- 2
- 3
- 4
- 5
Image Recognition and Dataset Categorization: summary, description and annotation
We offer to read an annotation, description, summary or preface (depends on what the author of the book "Image Recognition and Dataset Categorization" wrote himself). If you haven't found the necessary information about the book — write in the comments, we will try to find it.
Image Recognition and Dataset Categorization — read online for free the complete book (whole text) full work
Below is the text of the book, divided by pages. System saving the place of the last page read, allows you to conveniently read the book "Image Recognition and Dataset Categorization" online for free, without having to search again every time where you left off. Put a bookmark, and you can go to the page where you finished reading at any time.
Font size:
Interval:
Bookmark:
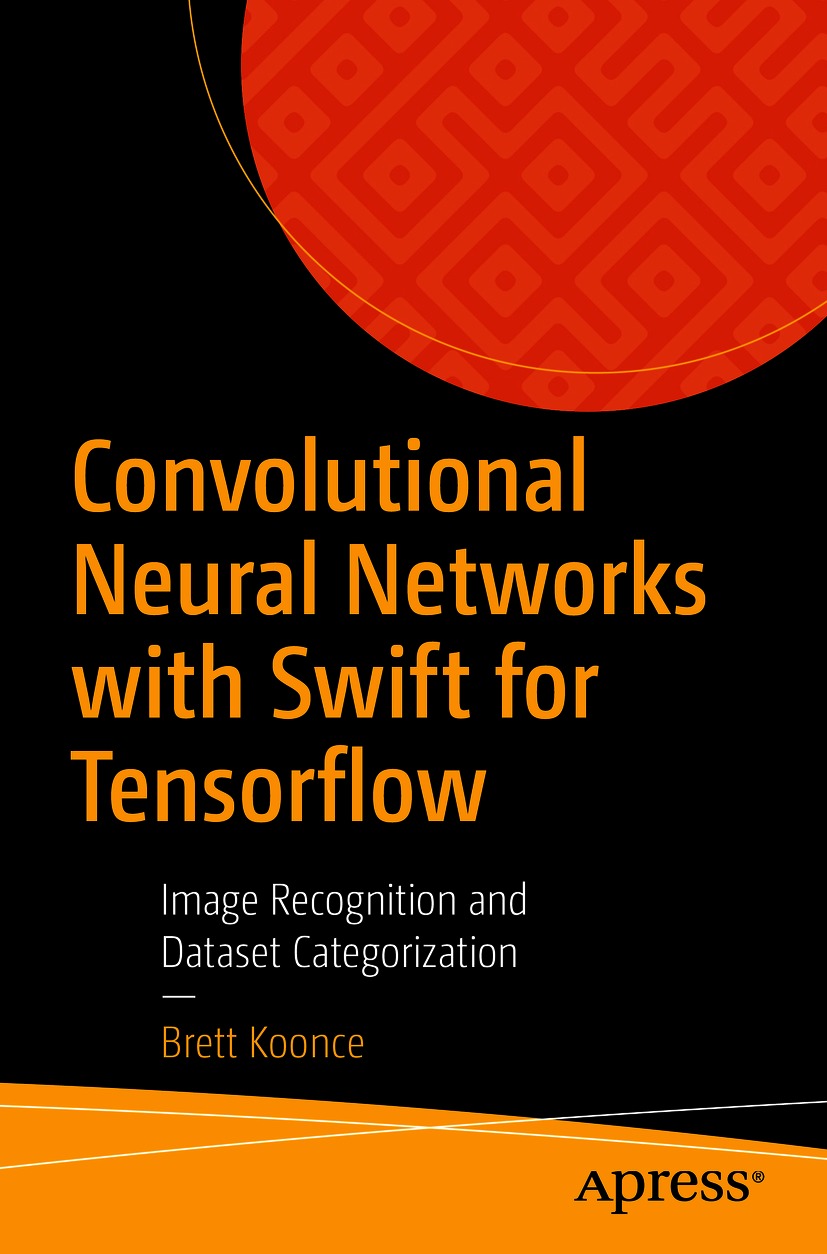
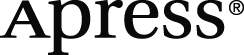
Any source code or other supplementary material referenced by the author in this book is available to readers on GitHub via the books product page, located at www.apress.com/978-1-4842-6167-5 . For more detailed information, please visit http://www.apress.com/source-code .
In this book, we are going to learn convolutional neural networks by focusing on the specific problem of image recognition, using Swift for Tensorflow and a command-line Unix approach. If you are new to this field, then I would suggest you read the first few chapters and get a working system bootstrapped and then spend your time going through the basics with MNIST and CIFAR repeatedly, in particular familiarizing yourself with how neural networks work. If you feel comfortable with the core concepts already, then feel free to skip ahead to the middle where we explore some more powerful convolutional neural networks.
The short version is that I believe swift is a modern, open source, beginner-friendly language that has proven itself by solving real problems for iOS developers daily. By integrating automatic differentiation into the programming language, a number of interesting compiler techniques to address the limitations of current machine learning software and hardware become possible in the long term. This is in my opinion where the world is headed, one way or another.
Image recognition is one of the oldest, most well-understood uses of neural networks. As a result, we can introduce the basics and then build up to advanced state-of-the-art approaches in a logically consistent manner. With this foundation, you will be able to branch out to tackle other image-related tasks (e.g., object detection and segmentation) easily. The deep learning techniques needed to build large-scale convolutional neural networks translate easily to reinforcement learning and generative adversarial networks (GANs) , two important areas of modern research. In addition, I believe this foundation will make it easy to make the transition to time sequence models such as recurrent neural networks (RNNs) and long short-term memory (LSTM) once you have mastered CNNs.
We can control costs very effectively. In the worst-case scenario, you can perform the majority of your work using a local machine built for under a thousand dollars, and your only remaining cost will be electricity and time.
We can scale easily from anywhere in the world. Using cloud instances full time can quickly become expensive, and so many people avoid learning cloud workflows. But using on-demand cloud-based resources periodically to augment your local workflow means you can learn the cloud in a very practical and efficient way. Eventually, you will be able to prototype and build solutions on your primary machine , then quickly scale them up in the cloud to parallelize computation and access more powerful hardware when needed or available.
We can get the best of both worlds. While minimizing costs is certainly important, I have found that focusing on how much money you are spending tends to produce a mindset where you are afraid to try new things and experiment in general. Building your own machine puts you into the mindset of putting in more cycles to reduce your costs, which is in my opinion the key to success.
We will use a local terminal interface to log in to all of our machines, so that there is literally no difference between our approaches on the desktop and in the cloud.
We will utilize the same operating system and software locally and in the cloud so that we do not have to learn about differences between platforms. Then, by definition, any workflow you can do on your computer, you will be able to do in the cloud, and vice versa.
Ultimately, by blurring the line between your personal computer and the cloud, my goal is for you to understand that there is fundamentally no difference between doing things locally or remotely. The real limiting factor then is your imagination, not resources.
Doing things this way will be more work at first, I will admit. But once you have mastered this workflow, it will be much easier for you to scale in the future. If you are willing to put in the time now, this approach will make your skills much more flexible and powerful in the future. What you do with them is up to you.
This book is organized as follows.
Neural networks (1D MLP/multilayer perceptron) and MNIST
Convolutional neural networks (2D CNN) and MNIST
Font size:
Interval:
Bookmark:
Similar books «Image Recognition and Dataset Categorization»
Look at similar books to Image Recognition and Dataset Categorization. We have selected literature similar in name and meaning in the hope of providing readers with more options to find new, interesting, not yet read works.
Discussion, reviews of the book Image Recognition and Dataset Categorization and just readers' own opinions. Leave your comments, write what you think about the work, its meaning or the main characters. Specify what exactly you liked and what you didn't like, and why you think so.