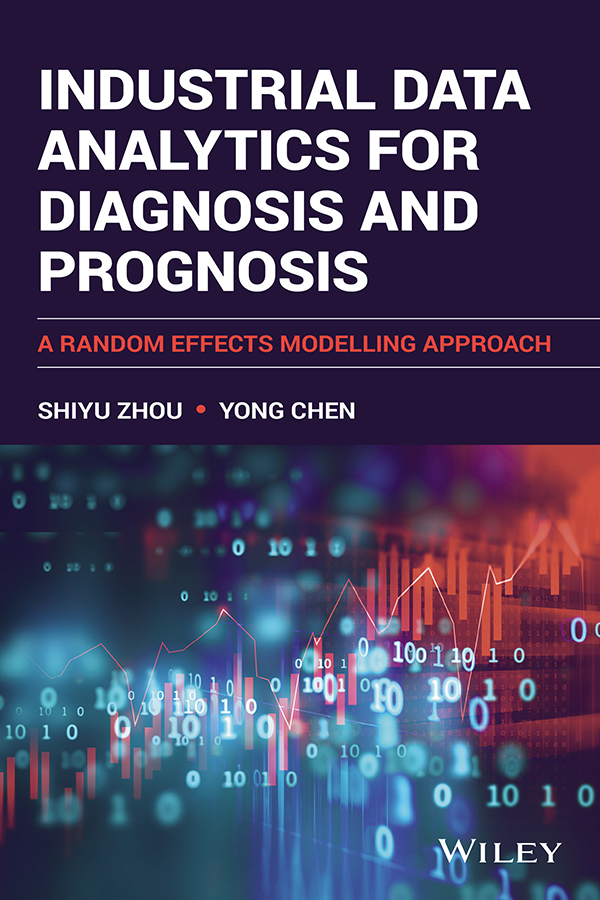
Introduction
1.1 Background and Motivation
Today, we are facing a data rich world that is changing faster than ever before. The ubiquitous availability of data provides great opportunities for industrial enterprises to improve their process quality and productivity. Indeed, the fast development of sensing, communication, and information technology has turned modern industrial systems into a data rich environment. For example, in a modern manufacturing process, it is now common to conduct a 100% inspection of product quality through automatic inspection stations. In addition, many modern manufacturing machines are numerically-controlled and equipped with many sensors and can provide various sensing data of the working conditions to the outside world.
One particularly important enabling technology in this trend is the Internet of Things (IoT) technology. IoT represents a network of physical devices, which enables ubiquitous data collection, communication, and sharing. One typical application of the IoT technology is the remote condition monitoring, diagnosis, and failure prognosis system for after-sales services. Such a system typically consists of three major components as shown in : (i) the in-field units (e.g., cars on the road), (ii) the communication network, and (iii) the back-office/cloud data processing center. The sensors embedded in the in-field unit continuously generate data, which are transmitted through the communication network to the back office. The aggregated data are then processed and analyzed at the back-office to assess system status and produce prognosis. The analytics results and the service alerts are passed individually to the in-field unit. Such a remote monitoring system can effectively improve the user experience, enhance the product safety, lower the ownership cost, and eventually gain competitive advantage for the manufacturer. Driven by the rapid development of information technology and the critical needs of providing fast and effective after-sales services to the products in a globalized market, the remote monitoring systems are becoming increasingly available.
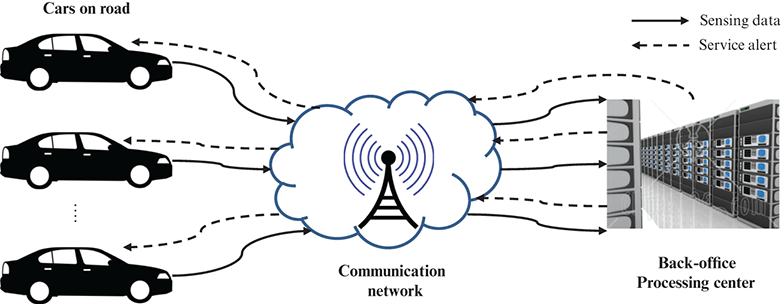
A diagram of an IoT enabled remote condition monitoring system.
The unprecedented data availability provides great opportunities for more precise and contextualized system condition monitoring, diagnosis, and prognosis, which are very challenging to achieve if only scarce data are available. Industrial data analytics is the process of collecting, exploring, and analyzing data generated from industrial operations throughout the product life cycle in order to gain insights and improve decision-making. Industrial data analytics encompasses a vast set of applied statistics and machine learning tools and techniques, including data visualization, data-driven process modeling, statistical process monitoring, root cause identification and diagnosis, predictive analytics, system reliability and robustness, and design of experiments, to name just a few. The focus of this book is industrial data analytics approaches that can take advantage of the unprecedented data availability. Particularly, we focus on the concept of random effects and its applications in system diagnosis and prognosis.
The terms diagnosis and prognosis were originally used in the medical field. Diagnosis is the identification of the disease that is responsible to the symptoms of the patients illness, and prognosis is a forecast of the likely course of the disease. In the field of engineering, these terms have similar meanings: for an industrial system, diagnosis is the identification of the root cause of a system failure or abnormal working condition; and prognosis is the prediction of the system degradation status and the future failure or break down. Obviously, diagnosis and prognosis play a critical role in assuring smooth, efficient, and safe system operations. Indeed, diagnosis and prognosis have attracted ever-growing interest in recent years. This trend has been driven by the fact that capital goods manufacturers have been coming under increasing pressure to open up new sources of revenue and profit in recent years. Maintenance service costs constitute around 6090% of the life-cycle costs of industrial machinery and installations. Systematic extension of the after-sales service business will be an increasingly important driver of profitable growth.
Due to the importance of diagnosis and prognosis in industrial system operations, a relatively large number of books/research monographs exist on this topic [Lewis et al., 2011, Niu, 2017, Wu et al., 2006, Talebi et al., 2009, Gertler, 1998, Chen and Patton, 2012, Witczak, 2007, Isermann, 2011, Ding, 2008, Si et al., 2017]. As implied by their titles, many of these books focus on model-based diagnosis and prognosis problems in dynamic systems. A model-based approach adopts a dynamic model, often in the form of a state space model, as the basis for diagnosis and prognosis. Then the difference between the observations and the model predictions, called residuals, are examined to achieve fault identification and diagnosis. For the prognosis, data-driven dynamic forecasting methods, such as time series modeling methods, are used to predict the future values of the interested system signals. The modeling and analysis of the system dynamics are the focus of the existing literature.
Different from the existing literature, this book focuses on the concept of random effects and its applications in system diagnosis and prognosis. Random effects, as the name implies, refer to the underlying random factors in an industrial process that impact on the outcome of the process. In diagnosis and prognosis applications, random effects can be used to model the sources of variation in a process and the variation among individual characteristics of multiple heterogeneous units. The following two examples illustrate the random effects in industrial processes.
Example 1.1Random effects in automotive body sheet metal assembly processes
The concept of variation source is illustrated for an assembly operation in which two parts are welded together. In an automotive sheet metal assembly process, the sheet metals will be positioned and clamped on the fixture system through the matching of the locators (also called pins) on the fixture system and the holes on the sheet metals. Then the sheet metals will be welded together. Clearly, the accuracy of the positions of the locating pins and the tightness of the matching between the pins and the holes significantly influence the dimensional accuracy of the final assembly. (a) shows the final product as designed. The assembly process is as follows: Part 1 is first located on the fixture and constrained by 4-way Pin L1 and 2-way Pin L2. A 4-way pin constrains the movement in two directions, while a 2-way pin only constrains the movement in one direction. Then, Part 2 is located by 4-way Pin L3 and 2-way Pin L4. The two parts are then welded together in a joining operation and released from the fixture.
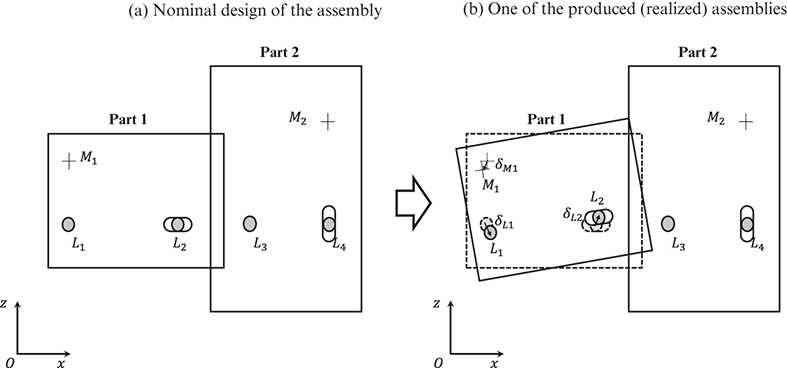
Random effects in an assembly operation.
If the position or diameter of Pin L1 deviates from design nominal, then Part 1 will consequently not be in its designed nominal position, as shown in (b) only shows one possible realization of produced assemblies. If we produce another assembly, the deviation of the position of Part 1 could be different. For instance, if the diameter of a pin is reduced due to pin wear, then the matching between the pin and the corresponding hole will be loose, which will lead to random wobble of the final position of part. This will in turn cause increased variation in the dimension of the produced final assemblies. As a result, mislocations of the pin can be manifested by either mean shift or variance change in the dimensional quality measurement such as
Next page