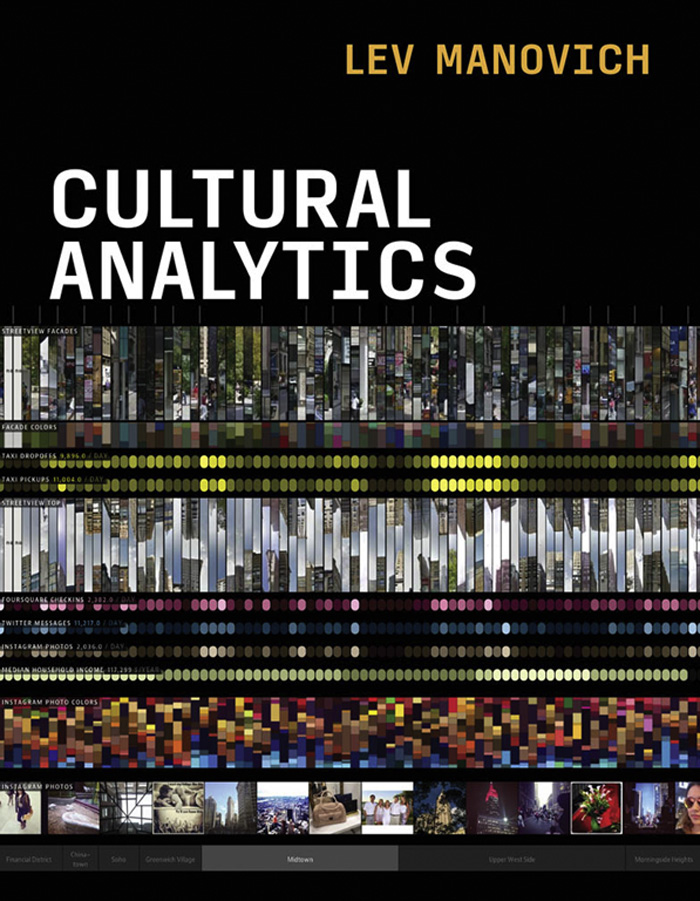
Cultural Analytics
Cultural Analytics
Lev Manovich
The MIT Press
Cambridge, Massachusetts
London, England
2020 Massachusetts Institute of Technology
All rights reserved. No part of this book may be reproduced in any form by any electronic or mechanical means (including photocopying, recording, or information storage and retrieval) without permission in writing from the publisher.
This book was set in ITC Stone Serif Std and ITC Stone Sans Std by New Best-set Typesetters Ltd.
Library of Congress Cataloging-in-Publication Data
Names: Manovich, Lev, author.
Title: Cultural analytics / Lev Manovich.
Description: Cambridge, Massachusetts : The MIT Press, [2020] | Includes bibliographical references and index.
Identifiers: LCCN 2020003045 | ISBN 9780262037105 (hardcover)
Subjects: LCSH: CultureResearchStatistical methods. | CultureResearchData processing. | Mass mediaResearchStatistical methods. | Mass mediaResearchData processing. | Information visualization.
Classification: LCC HM623 .M365 2020 | DDC 306.0285dc23
LC record available at https://lccn.loc.gov/2020003045
10 9 8 7 6 5 4 3 2 1
d_r0
Contents
Acknowledgments
I am very grateful to all the people and institutions that made this book possible:
The MIT Press: Doug Sery, senior acquisitions editor, Noah Springer, assistant acquisitions editor, Kathleen Caruso, manuscript editor, and Melinda Rankin, copyeditor.
Larry Star, director of the California Institute for Telecommunications and Information Technology (Calit2), Ramesh Rao, director of the UCSD Division of Calit2, and all the staff at Calit2, which has been supporting the work of our lab since its start in 2007.
Noah Wardrip-Fruin, who cofounded Software Studies Initiative (later renamed Cultural Analytics Lab) with me in 2007. Sheldon Brown, who invited us to the Center for Research in Computing and the Arts, which became our labs home from 2008 to 2012. Mathew Gold who supported my work at The Graduate Center, CUNY, after I started teaching there in 2013.
Lab members, 20072018: Jeremy Douglass, William Huber, Tara Zepel, Cicero Inacio da Silva, Jay Chow, Everardo Reyes, Mehrdad Yazdani, Damon Crockett, Nadav Hochman, Alise Tifentale, and Agustin Indaco.
Lab collaborators and visiting fellows: Moritz Stefaner, Dominikus Baur, Daniel Goddemeyer, Miriam Redi, Nadav Hochman, Almila Akdag, Jean-Franois Lucas, Tristan Thielmann, Hijoo Son, Kay OHalloran, Isabel Galhano Rodrigues, Falko Kuester, Jim Hollan, Matthew Fuller, Brynn Shepherd, and Leah Meisterlin.
Graduate and undergraduate students who worked in the lab: So Yamaoka, Sunsern Cheamanunku, Matias Giachino, Xiangfei Zeng, Cherie Huang, Chanda L. Carey, Daniel Rehn, Laura Hoeger, Rachel Cody, Devon Merill, Jia Gu, Agatha Man, Nichol Bernardo, Bob Li, Kedar Reddy, Christa Lee, Victoria Azurin, Xiaoda Wang, and Nadia Xiangfei Zeng.
The organizers of the UCLA IPAM Culture Analytics Institute (2016): Timothy Tangherlini, Tina Eliassi-Rad, Mauro Maggioni, and Vwani Roychowdhury.
The universities and educational programs where I have been permanent or visiting faculty between 2005 and 2020 (from the moment I first thought of cultural analytics to finishing this book): University of California, San Diego (UCSD); The Graduate Center, City University of New York (CUNY), National University of Singapore (NUS); Strelka Institute for Media, Architecture and Design; the European Graduate School (EGS); and the Institute of Social Sciences and Humanities, Tyumen State University (UTMN).
Illustrations
Examples of projects from our lab are used throughout the book to illustrate the concepts and techniques being presented. In my classes and workshops, I use the same approach because it allows me to show students the concrete steps involved in creating such projects, to discuss the multiple choices each step entails, and to point out what remained outside the analysis. Each of our projects has its own website or a web page where you will find descriptions, high-resolution color visualizations, and in some cases interactive interfaces with the datasets. You can access them from the Projects page of the Cultural Analytics Lab website:
- http://lab.culturalanalytics.info/p/projects.html
Note that although some of the visualizations appear in the book as color plates and others as grayscale figures, the originals are all in full color. Most of the visualizations are the result of joint work between lab members, with a few people working on each projectcreating the data, analyzing it and interpreting results, and making visualizations.
Introduction: How to See One Billion Images
The impact of the computer in the human sciences, however, is likely proportionally to be more revolutionary in the long run [than in physical and life sciences].... Some of it has to do simply with willingness to take advantage of the opportunity, or predisposition through already extensive use of processes, especially statistical, facilitated by the computer. More, perhaps, has to do with what a computer, in a sense like a telescope or a microscope, can enable us to see. In simplest terms, computer processing, properly prepared, can enable us to see relations and patterns in masses of data previously too large to comprehend; and to see the literal consequences of an idea applied to data, if not uniquely, then certainly far more inexorably and quickly.
Dell H. Hymes, Introduction, in The Use of Computers in Anthropology, 1965
This book is situated at the intersection of data science, media studies, and digital culture studies. It presents selected concepts and methods for computational analysis of cultural data. These methods can be used to explore digitized historical artifacts and contemporary digital media. While we can apply them to a single or a few artifacts, they become especially important if we want to explore millions of artifacts.
In fact, the astonishing scale of digital culture is what motivated me to start exploring these methods in 2005 and eventually write this book. How can we understand contemporary popular photography that grows by billions of images every day? Or contemporary music as represented by hundreds of millions of songs shared by twenty million creators on SoundCloud? Or the content of four billion boards on Pinterest? This is also digital culture because these physical events are enabled by the Meetup web platform. In my view, the only possible way to study the patterns, trends, and dynamics of contemporary culture at that scale is to use data science methods.
You do not need to have a background in data science, programming, statistics, or math to use this book. My intended audiences are academic researchers and students in art, design, the humanities, social sciences, media studies, data science, and computer science; professionals working in design, photography, film, urban design, architecture, journalism, museum and library fields, curating, and culture management; and everybody who works with social media and the web in any role (creator, blogger, strategist, manager, developer, marketer, etc.).
Even if you have no interest in analyzing cultural datasets yourself, you are encountering such analysis on a daily basis. Maybe you are looking at your Facebook, Instagram, or Weibo analytics, or Google Analytics for your blog or website, or using a social media monitoring dashboard at work. And if you dont pay attention to such data, you are constantly interacting with the results of computational analysis when you do anything digital. For example, every time you capture a photo, the phone camera algorithms automatically choose the exposure and adjust the contrast of the photo and also identify the type of scene and objects in the photo. Computational analysis of media artifacts and user interactions is what enables web search, recommendations, filtering, customization, interactions with digital devices, behavioral advertising, and other operations that form the vocabulary of digital culture. For example, web search engines such as Baidu, Bing, Yandex, or Google rely on continuous computational analysis of contents of billions of web pages, online images, and other web content to bring you relevant results.
Next page