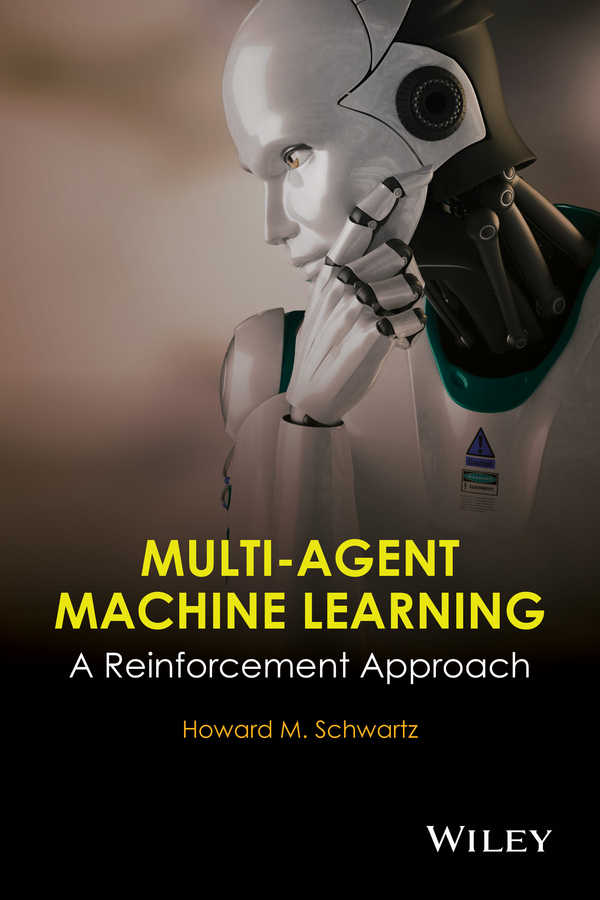
Copyright 2014 by John Wiley & Sons, Inc. All rights reserved
Published by John Wiley & Sons, Inc., Hoboken, New Jersey
Published simultaneously in Canada
No part of this publication may be reproduced, stored in a retrieval system, or transmitted in any form or by any means, electronic, mechanical, photocopying, recording, scanning, or otherwise, except as permitted under Section 107 or 108 of the 1976 United States Copyright Act, without either the prior written permission of the Publisher, or authorization through payment of the appropriate per-copy fee to the Copyright Clearance Center, Inc., 222 Rosewood Drive, Danvers, MA 01923, (978) 750-8400, fax (978) 750-4470, or on the web at www.copyright.com. Requests to the Publisher for permission should be addressed to the Permissions Department, John Wiley & Sons, Inc., 111 River Street, Hoboken, NJ 07030, (201) 748-6011, fax (201) 748-6008, or online at http://www.wiley.com/go/permissions.
Limit of Liability/Disclaimer of Warranty: While the publisher and author have used their best efforts in preparing this book, they make no representations or warranties with respect to the accuracy or completeness of the contents of this book and specifically disclaim any implied warranties of merchantability or fitness for a particular purpose. No warranty may be created or extended by sales representatives or written sales materials. The advice and strategies contained herein may not be suitable for your situation. You should consult with a professional where appropriate. Neither the publisher nor author shall be liable for any loss of profit or any other commercial damages, including but not limited to special, incidental, consequential, or other damages.
For general information on our other products and services or for technical support, please contact our Customer Care Department within the United States at (800) 762-2974, outside the United States at (317) 572-3993 or fax (317) 572-4002.
Wiley also publishes its books in a variety of electronic formats. Some content that appears in print may not be available in electronic formats. For more information about Wiley products, visit our web site at www.wiley.com.
Library of Congress Cataloging-in-Publication Data:
Schwartz, Howard M., editor.
Multi-agent machine learning : a reinforcement approach / Howard M. Schwartz.
pages cm
Includes bibliographical references and index.
ISBN 978-1-118-36208-2 (hardback)
1. Reinforcement learning. 2. Differential games. 3. Swarm intelligence. 4. Machine learning. I. Title.
Q325.6.S39 2014
519.3dc23
2014016950
Preface
For a decade I have taught a course on adaptive control. The course focused on the classical methods of system identification, using such classic texts as Ljung [1, 2]. The course addressed traditional methods of model reference adaptive control and nonlinear adaptive control using Lyapunov techniques. However, the theory had become out of sync with current engineering practice. As such, my own research and the focus of the graduate course changed to include adaptive signal processing, and to incorporate adaptive channel equalization and echo cancellation using the least mean squares (LMS) algorithm. The course name likewise changed, from Adaptive Control to Adaptive and Learning Systems. My research was still focused on system identification and nonlinear adaptive control with application to robotics. However, by the early 2000s, I had started work with teams of robots. It was now possible to use handy robot kits and low-cost microcontroller boards to build several robots that could work together. The graduate course in adaptive and learning systems changed again; the theoretical material on nonlinear adaptive control using Lyapunov techniques was reduced, replaced with ideas from reinforcement learning. A whole new range of applications developed. The teams of robots had to learn to work together and to compete.
Today, the graduate course focuses on system identification using recursive least squares techniques, some model reference adaptive control (still using Lyapunov techniques), adaptive signal processing using the LMS algorithm, and reinforcement learning using Q-learning. The first two chapters of this book present these ideas in an abridged form, but in sufficient detail to demonstrate the connections among the learning algorithms that are available; how they are the same; and how they are different. There are other texts that cover this material in detail [24].
The research then began to focus on teams of robots learning to work together. The work examined applications of robots working together for search and rescue applications, securing important infrastructure and border regions. It also began to focus on reinforcement learning and multiagent reinforcement learning. The robots are the learning agents. How do children learn how to play tag? How do we learn to play football, or how do police work together to capture a criminal? What strategies do we use, and how do we formulate these strategies? Why can I play touch football with a new group of people and quickly be able to assess everyone's capabilities and then take a particular strategy in the game?
As our research team began to delve further into the ideas associated with multiagent machine learning and game theory, we discovered that the published literature covered many ideas but was poorly coordinated or focused. Although there are a few survey articles [5], they do not give sufficient details to appreciate the different methods. The purpose of this book is to introduce the reader to a particular form of machine learning. The book focuses on multiagent machine learning, but it is tied together with the central theme of learning algorithms in general. Learning algorithms come in many different forms. However, they tend to have a similar approach. We will present the differences and similarities of these methods.
This book is based on my own work and the work of several doctoral and masters students who have worked under my supervision over the past 10 years. In particular, I would like to thank Prof. Sidney Givigi. Prof. Givigi was instrumental in developing the ideas and algorithms presented in Chapter 6. The doctoral research of Xiaosong (Eric) Lu has also found its way into this book. The work on guarding a territory is largely based on his doctoral dissertation. Other graduate students who helped me in this work include Badr Al Faiya, Mostafa Awheda, Pascal De Beck-Courcelle, and Sameh Desouky. Without the dedicated work of this group of students, this book would not have been possible.
H. M. Schwartz
Ottawa, Canada
September, 2013
References
- [1] L. Ljung, System Identification: Theory for the User. Upper Saddle River, NJ: Prentice Hall, 2nd ed., 1999.
- [2] L. Ljung and T. Soderstrom, Theory and Practice of Recursive Identification. Cambridge, Massachusetts: The MIT Press, 1983.
- [3] R. S. Sutton and A. G. Barto, Reinforcement learning: An Introduction. Cambridge, Massachusetts: The MIT Press, 1998.
- [4] Astrom, K. J. and Wittenmark, B., Adaptive Control. Boston, Massachusetts: Addison-Wesley Longman Publishing Co., Inc., 2nd ed., 1994, ISBN = 0201558661.
- [5] L. Bu
oniu and R. Babuka, and B. D. Schutter, A comprehensive survey of multiagent reinforcement learning, IEEE Trans. Syst. Man Cybern. Part C, Vol. , no. 2, pp. 156172, 2008.
Chapter 1
A Brief Review of Supervised Learning
There are a number of algorithms that are typically used for system identification, adaptive control, adaptive signal processing, and machine learning. These algorithms all have particular similarities and differences. However, they all need to process some type of experimental data. How we collect the data and process it determines the most suitable algorithm to use. In adaptive control, there is a device referred to as the
Next page