THE SEVEN PILLARS OF
STATISTICAL
WISDOM
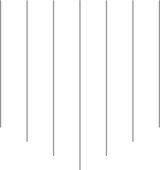
STEPHEN M. STIGLER

Cambridge, Massachusetts
London, England
2016
Copyright 2016 by the President and Fellows of Harvard College
All rights reserved
Jacket illustration: DigitalVision Vectors, DrAfter123, royalty free/Getty Images
Jacket design: Jill Breitbarth
978-0-674-08891-7 (pbk. : alk. paper)
978-0-674-97021-2 (EPUB)
978-0-674-97020-9 (MOBI)
The Library of Congress has cataloged the printed edition as follows:
Names: Stigler, Stephen M., author.
Title: The seven pillars of statistical wisdom / Stephen M. Stigler.
Description: Cambridge, Massachusetts : Harvard University Press, 2016. | Includes bibliographical references and index.
Identifiers: LCCN 2015033367
Subjects: LCSH: StatisticsHistory. | Mathematical statisticsHistory.
Classification: LCC QA276.15 S754 2016 | DDC 519.5dc23
LC record available at http://lccn.loc.gov/2015033367
To my grandchildren,
Ava and Ethan
CONTENTS
What is Statistics? This question was asked as early as 1838in reference to the Royal Statistical Societyand it has been asked many times since. The persistence of the question and the variety of answers that have been given over the years are themselves remarkable phenomena. Viewed together, they suggest that the persistent puzzle is due to Statistics not being only a single subject. Statistics has changed dramatically from its earliest days to the present, shifting from a profession that claimed such extreme objectivity that statisticians would only gather datanot analyze themto a profession that seeks partnership with scientists in all stages of investigation, from planning to analysis. Also, Statistics presents different faces to different sciences: In some applications, we accept the scientific model as derived from mathematical theory; in some, we construct a model that can then take on a status as firm as any Newtonian construction. In some, we are active planners and passive analysts; in others, just the reverse. With so many faces, and the consequent challenges of balance to avoid missteps, it is no wonder that the question, What is Statistics? has arisen again and again, whenever a new challenge arrives, be it the economic statistics of the 1830s, the biological questions of the 1930s, or the vaguely defined big data questions of the present age.
With all the variety of statistical questions, approaches, and interpretations, is there then no core science of Statistics? If we are fundamentally dedicated to working in so many different sciences, from public policy to validating the discovery of the Higgs boson, and we are sometimes seen as mere service personnel, can we really be seen in any reasonable sense as a unified discipline, even as a science of our own? This is the question I wish to address in this book. I will not try to tell you what Statistics is or is not; I will attempt to formulate seven principles, seven pillars that have supported our field in different ways in the past and promise to do so into the indefinite future. I will try to convince you that each of these was revolutionary when introduced, and each remains a deep and important conceptual advance.
My title is an echo of a 1926 memoir, Seven Pillars of Wisdom, by T. E. Lawrence, Lawrence of Arabia. Its relevance comes from Lawrences own source, the Old Testaments Book of Proverbs 9:1, which reads, Wisdom hath built her house, she hath hewn out her seven pillars. According to Proverbs, Wisdoms house was constructed to welcome those seeking understanding; my version will have an additional goal: to articulate the central intellectual core of statistical reasoning.
In calling these seven principles the Seven Pillars of Statistical Wisdom, I hasten to emphasize that these are seven support pillarsthe disciplinary foundation, not the whole edifice, of Statistics. All seven have ancient origins, and the modern discipline has constructed its many-faceted science upon this structure with great ingenuity and with a constant supply of exciting new ideas of splendid promise. But without taking away from that modern work, I hope to articulate a unity at the core of Statistics both across time and between areas of application.
The first pillar I will call Aggregation, although it could just as well be given the nineteenth-century name, The Combination of Observations, or even reduced to the simplest example, taking a mean. Those simple names are misleading, in that I refer to an idea that is now old but was truly revolutionary in an earlier dayand it still is so today, whenever it reaches into a new area of application. How is it revolutionary? By stipulating that, given a number of observations, you can actually gain information by throwing information away! In taking a simple arithmetic mean, we discard the individuality of the measures, subsuming them to one summary. It may come naturally now in repeated measurements of, say, a star position in astronomy, but in the seventeenth century it might have required ignoring the knowledge that the French observation was made by an observer prone to drink and the Russian observation was made by use of an old instrument, but the English observation was by a good friend who had never let you down. The details of the individual observations had to be, in effect, erased to reveal a better indication than any single observation could on its own.
The earliest clearly documented use of an arithmetic mean was in 1635; other forms of statistical summary have a much longer history, back to Mesopotamia and nearly to the dawn of writing. Of course, the recent important instances of this first pillar are more complicated. The method of least squares and its cousins and descendants are all averages; they are weighted aggregates of data that submerge the identity of individuals, except for designated covariates. And devices like kernel estimates of densities and various modern smoothers are averages, too.
The second pillar is Information, more specifically Information Measurement, and it also has a long and interesting intellectual history. The question of when we have enough evidence to be convinced a medical treatment works goes back to the Greeks. The mathematical study of the rate of information accumulation is much more recent. In the early eighteenth century it was discovered that in many situations the amount of information in a set of data was only proportional to the square root of the number n of observations, not the number n itself. This, too, was revolutionary: imagine trying to convince an astronomer that if he wished to double the accuracy of an investigation, he needed to quadruple the number of observations, or that the second 20 observations were not nearly so informative as the first 20, despite the fact that all were equally accurate? This has come to be called the root-n rule; it required some strong assumptions, and it required modification in many complicated situations. In any event, the idea that information in data could be measured, that accuracy was related to the amount of data in a way that could be precisely articulated in some situations, was clearly established by 1900.
By the name I give to the third pillar, Likelihood, I mean the calibration of inferences with the use of probability. The simplest form for this is in significance testing and the common P-value, but as the name Likelihood hints, there is a wealth of associated methods, many related to parametric families or to Fisherian or Bayesian inference. Testing in one form or another goes back a thousand years or more, but some of the earliest tests to use probability were in the early eighteenth century. There were many examples in the 1700s and 1800s, but systematic treatment only came with the twentieth-century work of Ronald A. Fisher and of Jerzy Neyman and Egon S. Pearson, when a full theory of likelihood began serious development. The use of probability to calibrate inference may be most familiar in testing, but it occurs everywhere a number is attached to an inference, be it a confidence interval or a Bayesian posterior probability. Indeed, Thomas Bayess theorem was published 250 years ago for exactly that purpose.
Next page