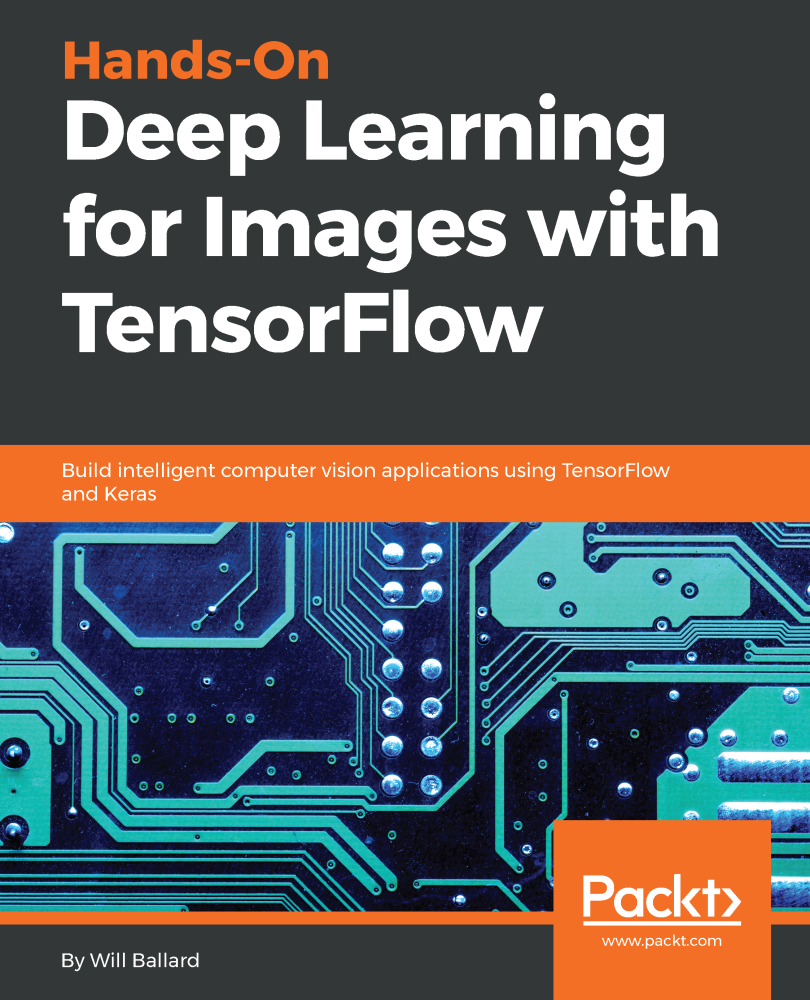
Hands-On Deep Learning for Images with TensorFlow
Build intelligent computer vision applications using TensorFlow and Keras
Will Ballard
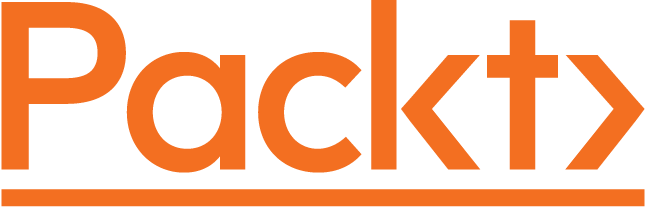
BIRMINGHAM - MUMBAI
Hands-On Deep Learning for Images with TensorFlow
Copyright 2018 Packt Publishing
All rights reserved. No part of this book may be reproduced, stored in a retrieval system, or transmitted in any form or by any means, without the prior written permission of the publisher, except in the case of brief quotations embedded in critical articles or reviews.
Every effort has been made in the preparation of this book to ensure the accuracy of the information presented. However, the information contained in this book is sold without warranty, either express or implied. Neither the author, nor Packt Publishing or its dealers and distributors, will be held liable for any damages caused or alleged to have been caused directly or indirectly by this book.
Packt Publishing has endeavored to provide trademark information about all of the companies and products mentioned in this book by the appropriate use of capitals. However, Packt Publishing cannot guarantee the accuracy of this information.
Commissioning Editor: Sunith Shetty
Acquisition Editor: Joshua Nadar
Content Development Editor: Dinesh Pawar
Technical Editor: Suwarna Patil
Copy Editor: SAFIS
Project Coordinator: Nidhi Joshi
Proofreader: SAFIS
Indexer: Pratik Shirodkar
Graphics: Jisha Chirayil
Production Coordinator: Shantanu Zagade
First published: July 2018
Production reference: 1300718
Published by Packt Publishing Ltd.
Livery Place
35 Livery Street
Birmingham
B3 2PB, UK.
ISBN 978-1-78953-867-0
www.packtpub.com
mapt.io
Mapt is an online digital library that gives you full access to over 5,000 books and videos, as well as industry leading tools to help you plan your personal development and advance your career. For more information, please visit our website.
Why subscribe?
Spend less time learning and more time coding with practical eBooks and Videos from over 4,000 industry professionals
Improve your learning with Skill Plans built especially for you
Get a free eBook or video every month
Mapt is fully searchable
Copy and paste, print, and bookmark content
PacktPub.com
Did you know that Packt offers eBook versions of every book published, with PDF and ePub files available? You can upgrade to the eBook version at www.PacktPub.com and as a print book customer, you are entitled to a discount on the eBook copy. Get in touch with us at service@packtpub.com for more details.
At www.PacktPub.com , you can also read a collection of free technical articles, sign up for a range of free newsletters, and receive exclusive discounts and offers on Packt books and eBooks.
Contributors
About the author
Will Ballard is the chief technology officer at GLG, responsible for engineering and IT. He was also responsible for the design and operation of large data centers that helped run site services for customers including Gannett, Hearst Magazines, NFL.com, NPR, The Washington Post, and Whole Foods. He has also held leadership roles in software development at NetSolve (now Cisco), NetSpend, and Works.com (now Bank of America).
Packt is searching for authors like you
If you're interested in becoming an author for Packt, please visit authors.packtpub.com and apply today. We have worked with thousands of developers and tech professionals, just like you, to help them share their insight with the global tech community. You can make a general application, apply for a specific hot topic that we are recruiting an author for, or submit your own idea.
Preface
TensorFlow is Google's popular offering for machine learning and deep learning. It has quickly become a popular choice of tool for performing fast, efficient, and accurate deep learning tasks.
This book shows you practical implementations of real-world projects, teaching you how to leverage TensorFlow 's capabilities to perform efficient deep learning. In this book, you will be acquainted with the different paradigms of performing deep learning, such as deep neural nets, convolutional neural networks, recurrent neural networks, and more, and how they can be implemented using TensorFlow.
This will be demonstrated with the help of end-to-end implementations of three real-world projects on popular topic areas such as natural language processing, image classification, and fraud detection.
By the end of this book, you will have mastered all the concepts of deep learning and their implementations with TensorFlow and Keras.
Who this book is for
This book is for application developers, data scientists, and machine learning practitioners looking to integrate machine learning into application software and master deep learning by implementing practical projects in TensorFlow. Knowledge of Python programming and the basics of deep learning is required to get the most out of this book.
What this book covers
, Machine Learning Toolkit , looks into installing Docker, setting up a machine learning Docker file, sharing data back with your host computer, and running a REST service to provide the environment.
, Image Data , teaches MNIST digits, how to acquire them, how tensors are really just multidimensional arrays, and how we can encode image data and categorical or classification data as a tensor. Then, we have a quick review and a cookbook approach to consider dimensions and tensors, in order to get data prepared for machine learning.
, Classical Neural Network , covers an awful lot of material! We see the structure of the classical, or dense, neural network. We learn about activation, nonlinearity, and softmax. We then set up testing and training data and learn how to construct the network with Dropout and Flatten. We also learn all about solvers, or how machine actually learns. We then explore hyperparameters, and finally, we fine-tune our model by means of grid search.
, A Convolutional Neural Network , teaches you convolutions, which are a loosely connected way of moving over an image to extract features. Then we learn about pooling, which summarizes the most important features. We will build a convolutional neural network using these techniques and we combine many layers of convolution and pooling in order to generate a deep neural network.
, An Image Classification Server , uses a Swagger API definition to create a REST API model, which then declaratively generates the Python framework in order for us to serve that API. Then, we create a Docker container that captures not only our running code (that is, our service) but also our pre-trained machine learning model. This then forms a package so that we are able to deploy and use our container. Finally, we use this container to serve and make predictions.
Next page